UNIMO-3: Multi-granularity Interaction for Vision-Language Representation Learning
CoRR(2023)
摘要
Vision-and-language (VL) pre-training, which aims to learn a general representation of image-text pairs that can be transferred to various vision-and-language tasks. Compared with modeling uni-modal data, the main challenge of the VL model is: how to learn the cross-modal interaction from multimodal data, especially the fine-grained interaction. Existing works have shown that fully transformer-based models that adopt attention mechanisms to learn in-layer cross-model interaction can demonstrate impressive performance on various cross-modal downstream tasks. However, they ignored that the semantic information of the different modals at the same layer was not uniform, which leads to the cross-modal interaction collapsing into a limited multi-modal semantic information interaction. In this work, we propose the UNIMO-3 model, which has the capacity to simultaneously learn the multimodal in-layer interaction and cross-layer interaction. UNIMO-3 model can establish effective connections between different layers in a cross-modal encoder, and adaptively capture the interaction between two modalities at different levels. The experimental results show that our model achieves state-of-the-art performance in various downstream tasks, and through ablation study can prove that effective cross-layer learning improves the model's ability of multimodal representation.
更多查看译文
关键词
representation,learning,multi-granularity,vision-language
AI 理解论文
溯源树
样例
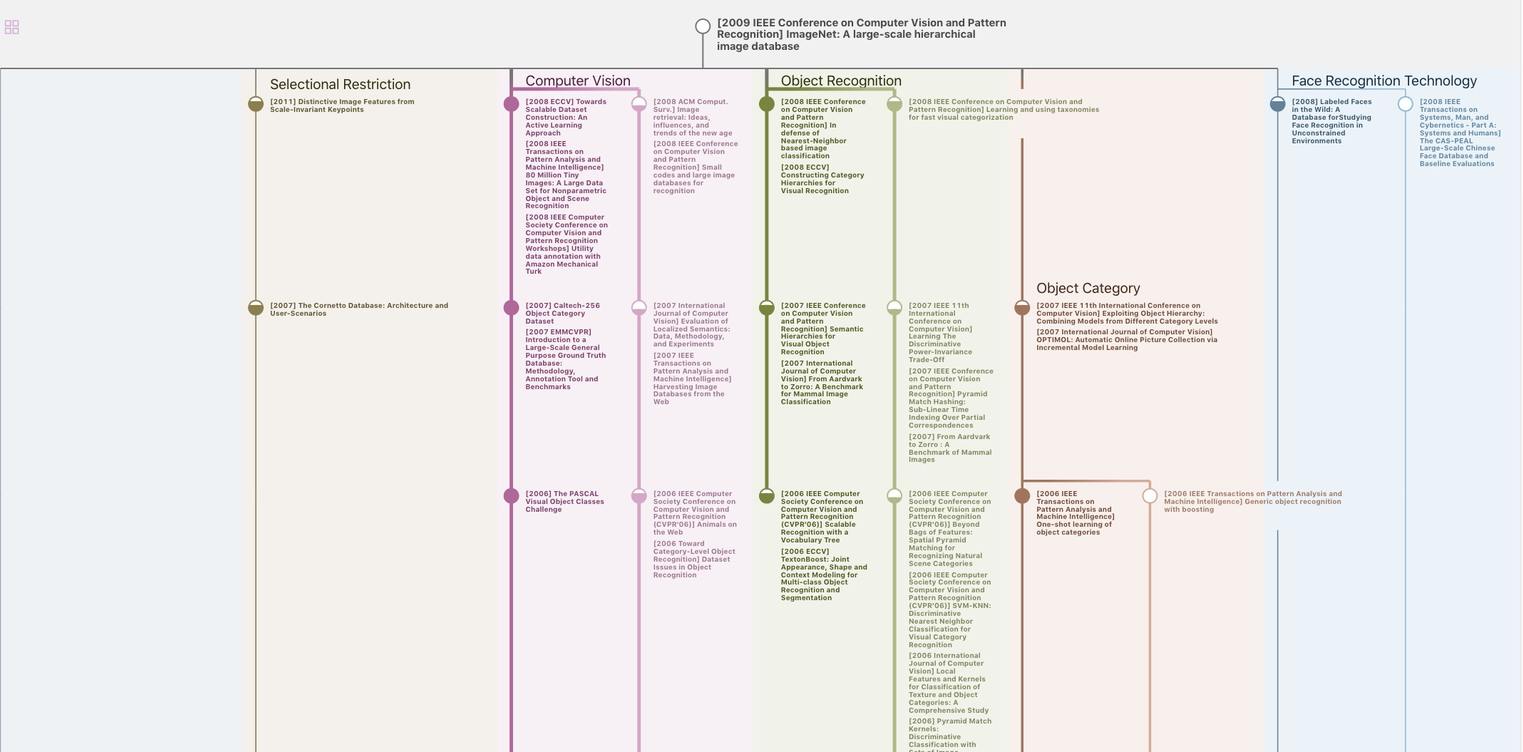
生成溯源树,研究论文发展脉络
Chat Paper
正在生成论文摘要