VIP5: Towards Multimodal Foundation Models for Recommendation.
CoRR(2023)
Abstract
Computer Vision (CV), Natural Language Processing (NLP), and Recommender Systems (RecSys) are three prominent AI applications that have traditionally developed independently, resulting in disparate modeling and engineering methodologies. This has impeded the ability for these fields to directly benefit from each other's advancements. With the increasing availability of multimodal data on the web, there is a growing need to consider various modalities when making recommendations for users. With the recent emergence of foundation models, large language models have emerged as a potential general-purpose interface for unifying different modalities and problem formulations. In light of this, we propose the development of a multimodal foundation model by considering both visual and textual modalities under the P5 recommendation paradigm (VIP5) to unify various modalities and recommendation tasks. This will enable the processing of vision, language, and personalization information in a shared architecture for improved recommendations. To achieve this, we introduce multimodal personalized prompts to accommodate multiple modalities under a shared format. Additionally, we propose a parameter-efficient training method for foundation models, which involves freezing the backbone and fine-tuning lightweight adapters, resulting in improved recommendation performance and increased efficiency in terms of training time and memory usage.
MoreTranslated text
Key words
multimodal foundation models,recommendation
AI Read Science
Must-Reading Tree
Example
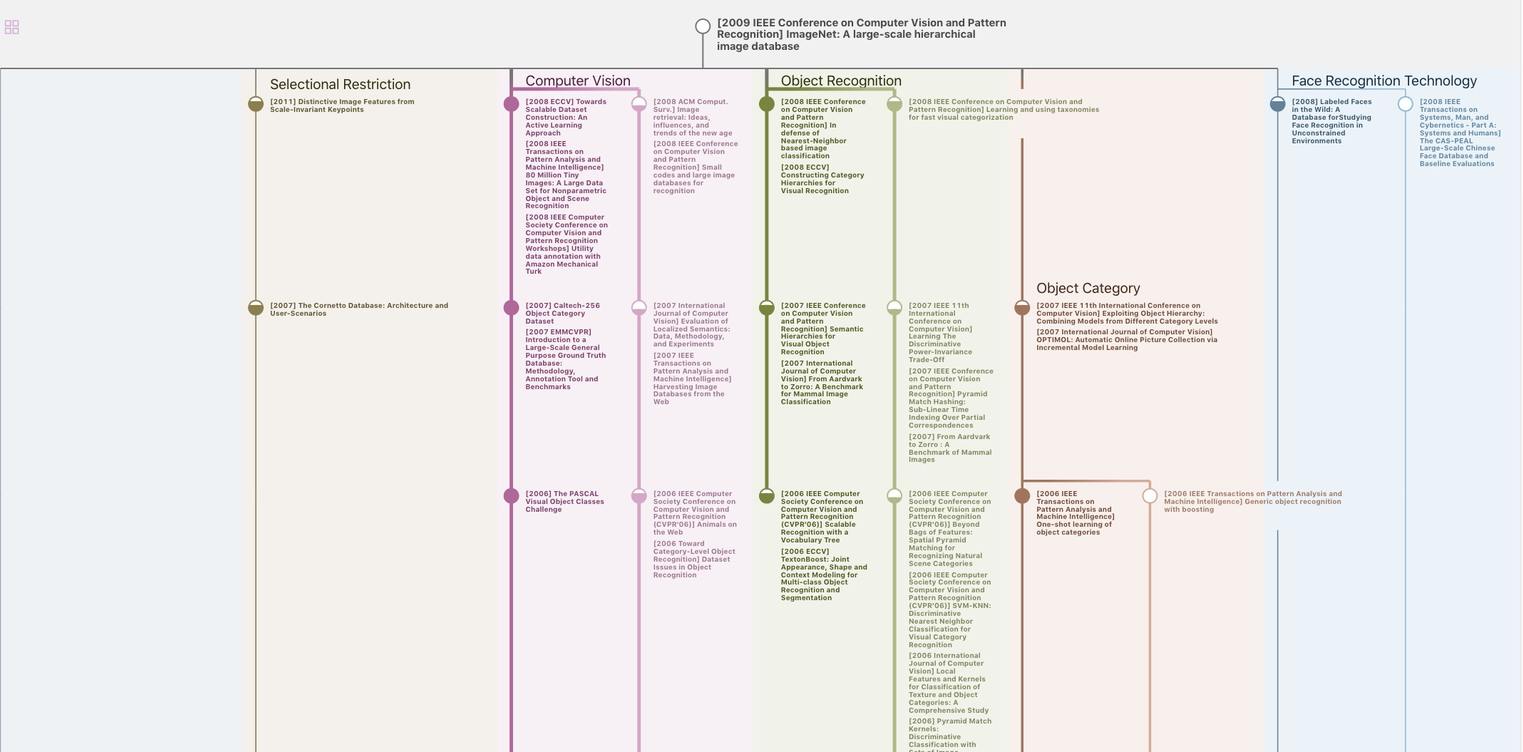
Generate MRT to find the research sequence of this paper
Chat Paper
Summary is being generated by the instructions you defined