Efficient Convolutional Processing of Spiking Neural Network With Weight-Sharing Filters
IEEE Electron Device Letters(2023)
摘要
The importance of implementing an efficient convolutional neural network (CNN) is increasing. A weight-sharing spiking CNN inference system (WS-SCNN) employing efficient convolution layers (ECLs) is proposed and modeled to enable the compact convolutional processing of the spiking neural network (SNN) inference. The proposed ECL efficiently maps convolutional features between inputs and filter weights. The ECL does not replicate the synaptic filter array with respect to input sliding, which minimizes the number of synaptic devices required to implement hardware SNNs. A four-bit weight quantization capability of a fabricated charge-trap flash synaptic device is used to verify the accurate multiplication and summation of weights in the ECL. Moreover, a nine-layer WS-SCNN consisting of multiple ECLs is modeled, and the benefits of the WS-SCNN in terms of the area and energy are evaluated. Simulation results show that the WS-SCNN has 5.68 and 103.5 times higher energy and area efficiency than conventional SCNN systems, respectively.
更多查看译文
关键词
Charge trap flash (CTF),efficient convolutional processing,spiking neural network (SNN)
AI 理解论文
溯源树
样例
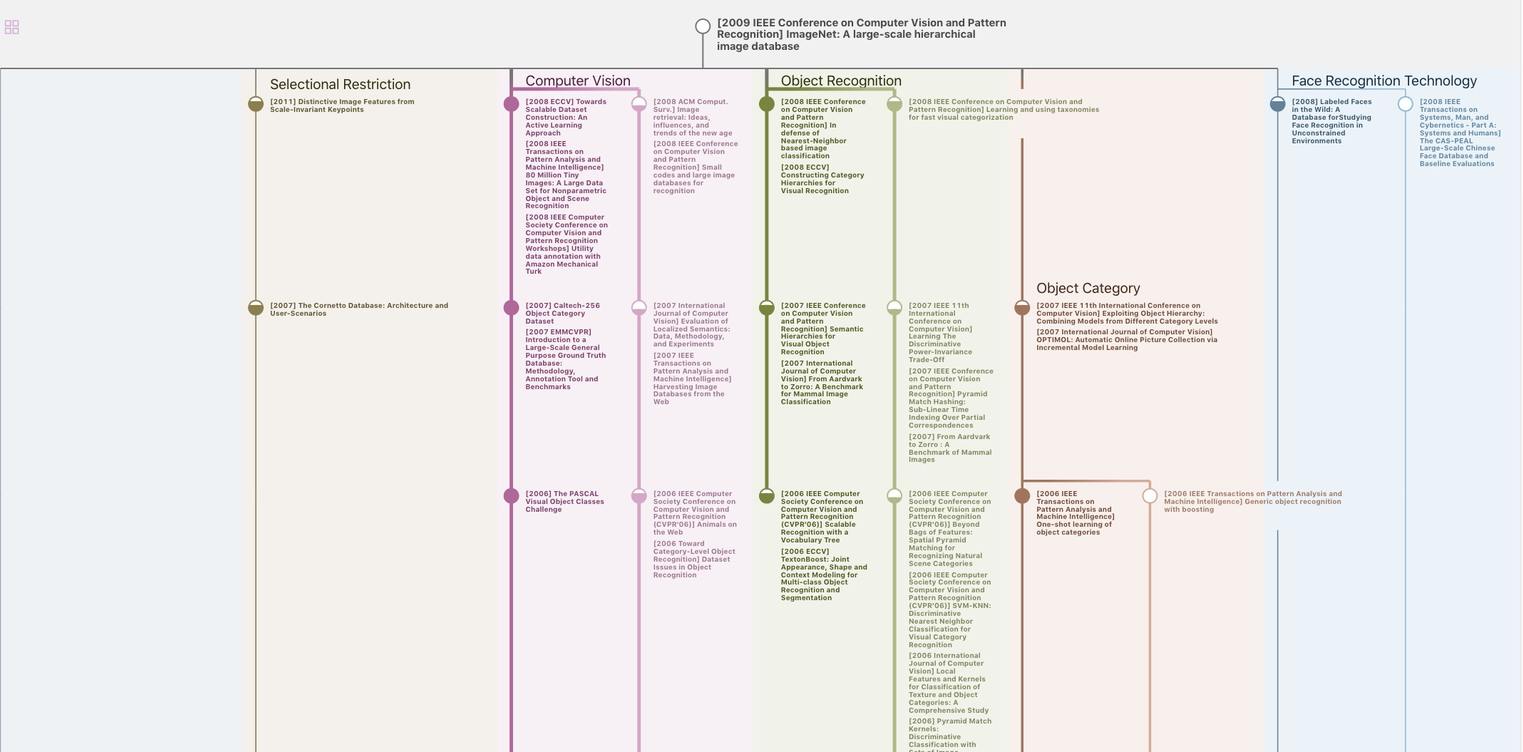
生成溯源树,研究论文发展脉络
Chat Paper
正在生成论文摘要