Adversarial Nibbler: A Data-Centric Challenge for Improving the Safety of Text-to-Image Models
CoRR(2023)
摘要
The generative AI revolution in recent years has been spurred by an expansion in compute power and data quantity, which together enable extensive pre-training of powerful text-to-image (T2I) models. With their greater capabilities to generate realistic and creative content, these T2I models like DALL-E, MidJourney, Imagen or Stable Diffusion are reaching ever wider audiences. Any unsafe behaviors inherited from pretraining on uncurated internet-scraped datasets thus have the potential to cause wide-reaching harm, for example, through generated images which are violent, sexually explicit, or contain biased and derogatory stereotypes. Despite this risk of harm, we lack systematic and structured evaluation datasets to scrutinize model behavior, especially adversarial attacks that bypass existing safety filters. A typical bottleneck in safety evaluation is achieving a wide coverage of different types of challenging examples in the evaluation set, i.e., identifying 'unknown unknowns' or long-tail problems. To address this need, we introduce the Adversarial Nibbler challenge. The goal of this challenge is to crowdsource a diverse set of failure modes and reward challenge participants for successfully finding safety vulnerabilities in current state-of-the-art T2I models. Ultimately, we aim to provide greater awareness of these issues and assist developers in improving the future safety and reliability of generative AI models. Adversarial Nibbler is a data-centric challenge, part of the DataPerf challenge suite, organized and supported by Kaggle and MLCommons.
更多查看译文
关键词
adversarial nibbler,models,challenge,data-centric,text-to-image
AI 理解论文
溯源树
样例
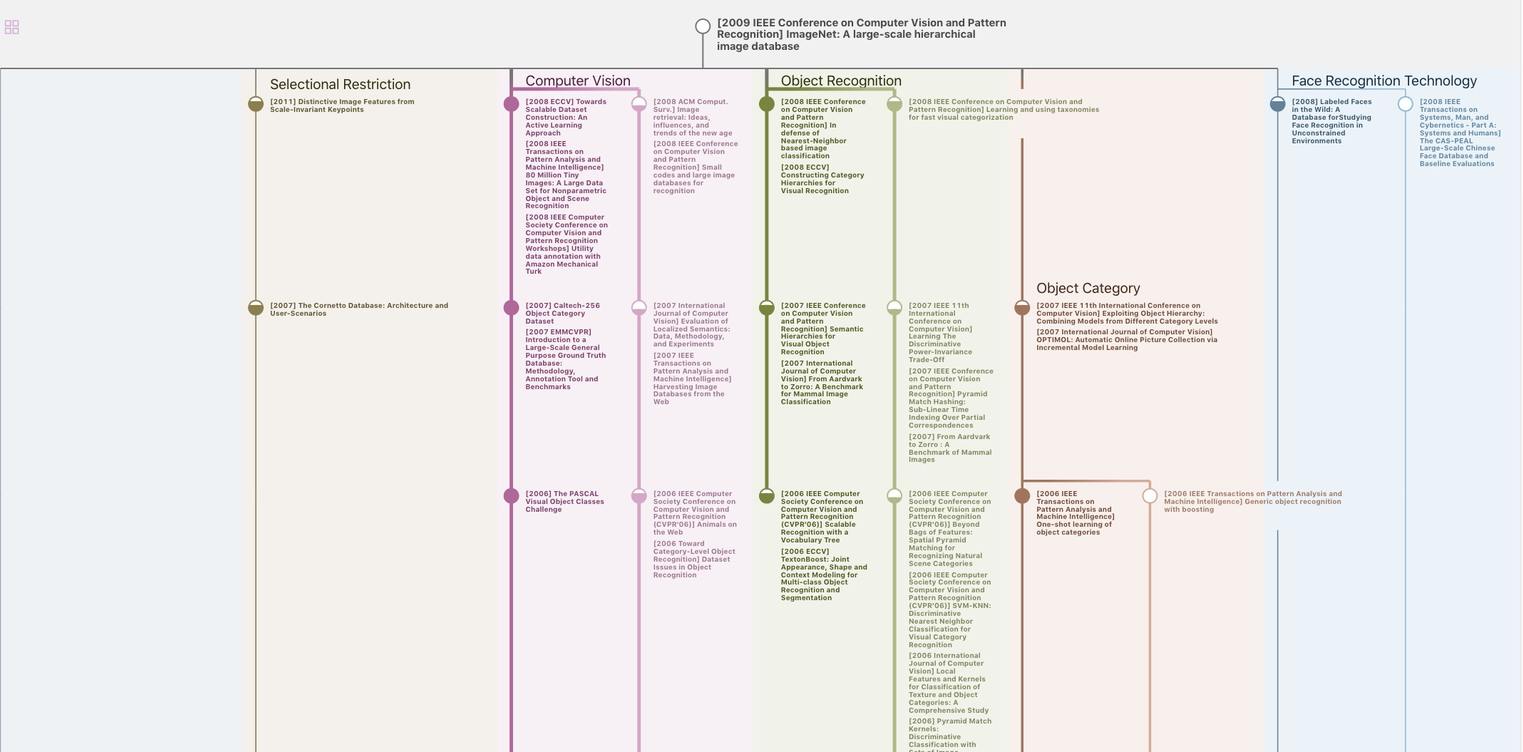
生成溯源树,研究论文发展脉络
Chat Paper
正在生成论文摘要