On the Transferability of Whisper-based Representations for "In-the-Wild" Cross-Task Downstream Speech Applications
CoRR(2023)
摘要
Large self-supervised pre-trained speech models have achieved remarkable success across various speech-processing tasks. The self-supervised training of these models leads to universal speech representations that can be used for different downstream tasks, ranging from automatic speech recognition (ASR) to speaker identification. Recently, Whisper, a transformer-based model was proposed and trained on large amount of weakly supervised data for ASR; it outperformed several state-of-the-art self-supervised models. Given the superiority of Whisper for ASR, in this paper we explore the transferability of the representation for four other speech tasks in SUPERB benchmark. Moreover, we explore the robustness of Whisper representation for ``in the wild'' tasks where speech is corrupted by environment noise and room reverberation. Experimental results show Whisper achieves promising results across tasks and environmental conditions, thus showing potential for cross-task real-world deployment.
更多查看译文
关键词
transferability,speech,representations,whisper-based,in-the-wild,cross-task
AI 理解论文
溯源树
样例
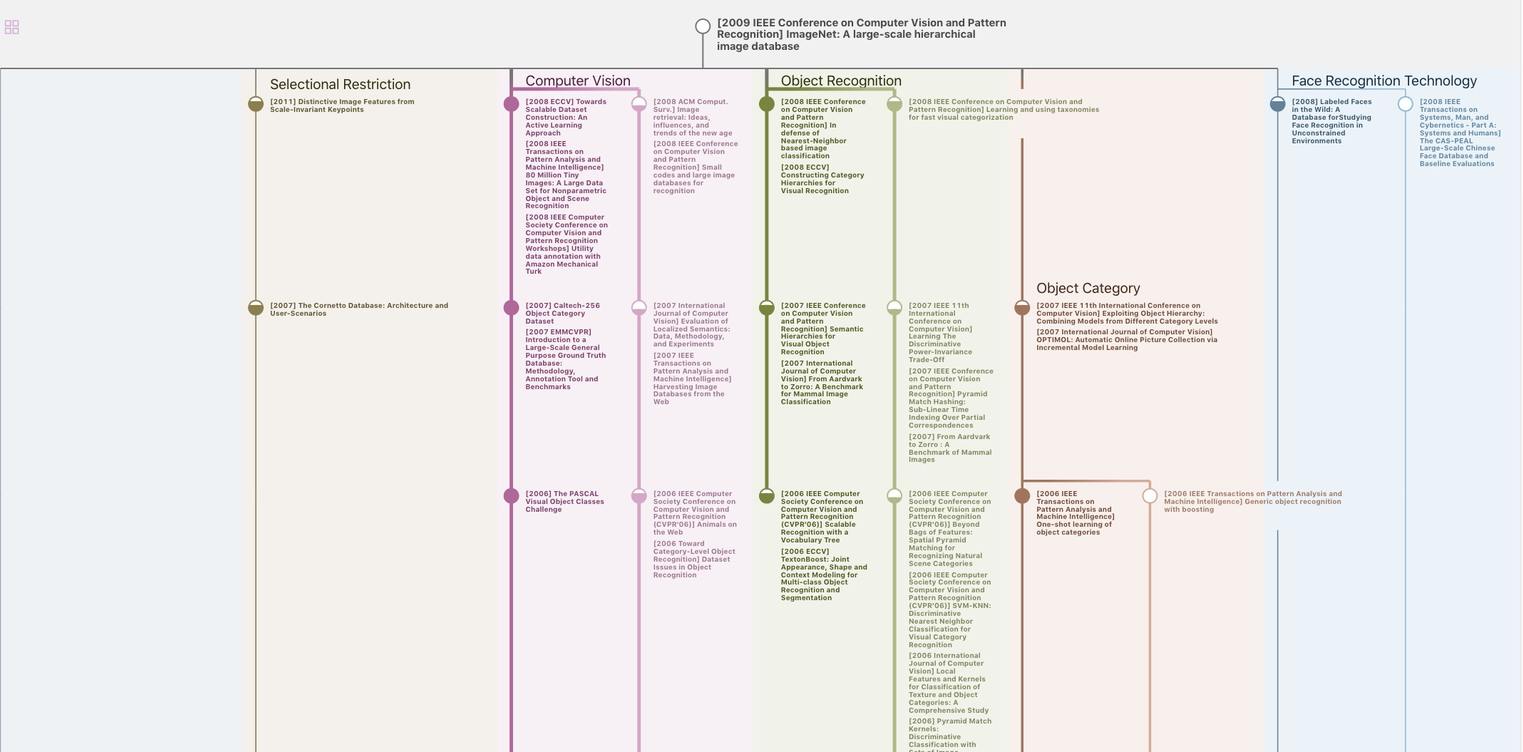
生成溯源树,研究论文发展脉络
Chat Paper
正在生成论文摘要