In-Context Demonstration Selection with Cross Entropy Difference.
CoRR(2023)
摘要
Large language models (LLMs) can use in-context demonstrations to improve performance on zero-shot tasks. However, selecting the best in-context examples is challenging because model performance can vary widely depending on the selected examples. We present a cross-entropy difference (CED) method for selecting in-context demonstrations. Our method is based on the observation that the effectiveness of in-context demonstrations negatively correlates with the perplexity of the test example by a language model that was finetuned on that demonstration. We utilize parameter efficient finetuning to train small models on training data that are used for computing the cross-entropy difference between a test example and every candidate in-context demonstration. This metric is used to rank and select in-context demonstrations independently for each test input. We evaluate our method on a mix-domain dataset that combines 8 benchmarks, representing 4 text generation tasks, showing that CED for in-context demonstration selection can improve performance for a variety of LLMs.
更多查看译文
关键词
demonstration,entropy,in-context
AI 理解论文
溯源树
样例
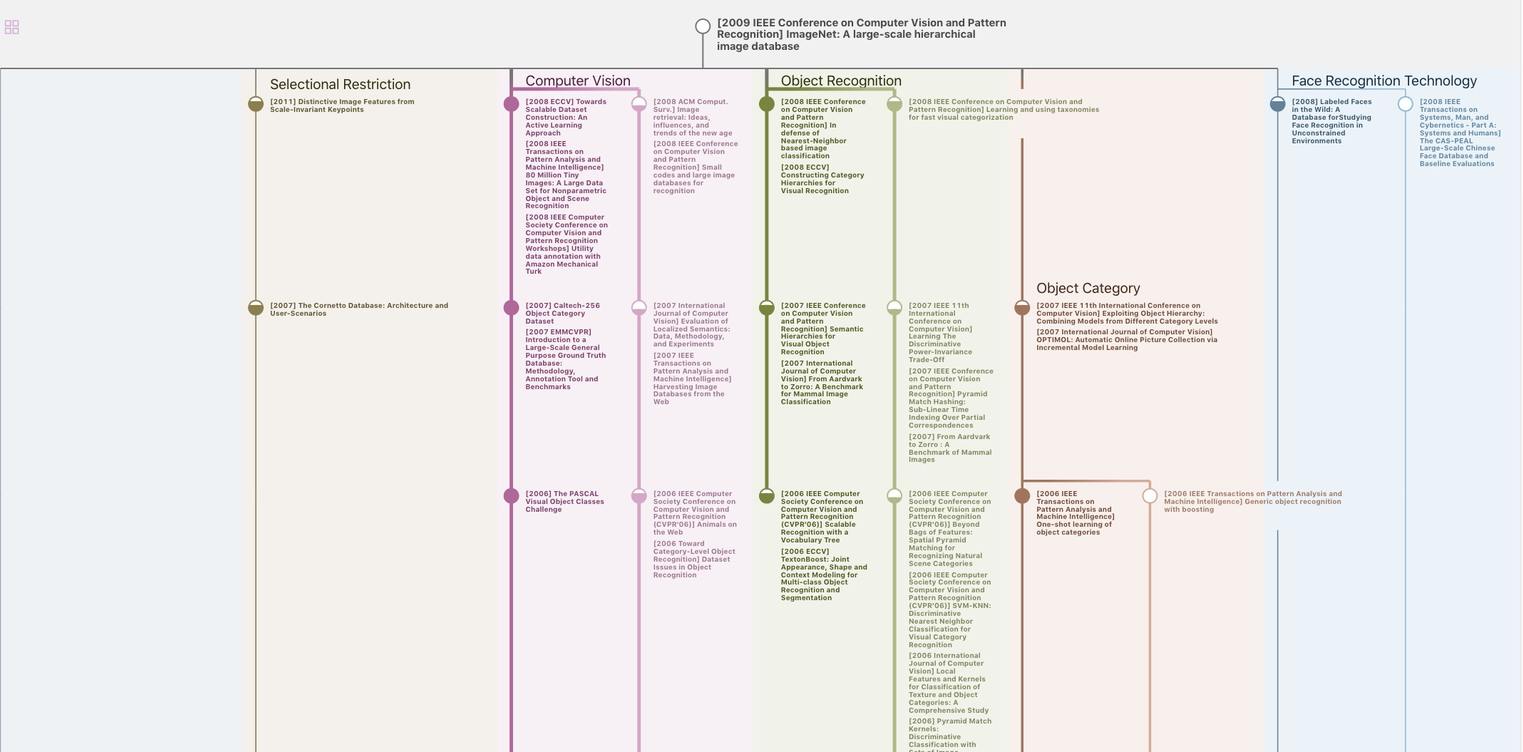
生成溯源树,研究论文发展脉络
Chat Paper
正在生成论文摘要