ByteSized32: A Corpus and Challenge Task for Generating Task-Specific World Models Expressed as Text Games.
CoRR(2023)
摘要
In this work we examine the ability of language models to generate explicit world models of scientific and common-sense reasoning tasks by framing this as a problem of generating text-based games. To support this, we introduce ByteSized32, a corpus of 32 highly-templated text games written in Python totaling 24k lines of code, each centered around a particular task, and paired with a set of 16 unseen text game specifications for evaluation. We propose a suite of automatic and manual metrics for assessing simulation validity, compliance with task specifications, playability, winnability, and alignment with the physical world. In a single-shot evaluation of GPT-4 on this simulation-as-code-generation task, we find it capable of producing runnable games in 27% of cases, highlighting the difficulty of this challenge task. We discuss areas of future improvement, including GPT-4's apparent capacity to perform well at simulating near canonical task solutions, with performance dropping off as simulations include distractors or deviate from canonical solutions in the action space.
更多查看译文
关键词
world models,challenge task,corpus,task-specific
AI 理解论文
溯源树
样例
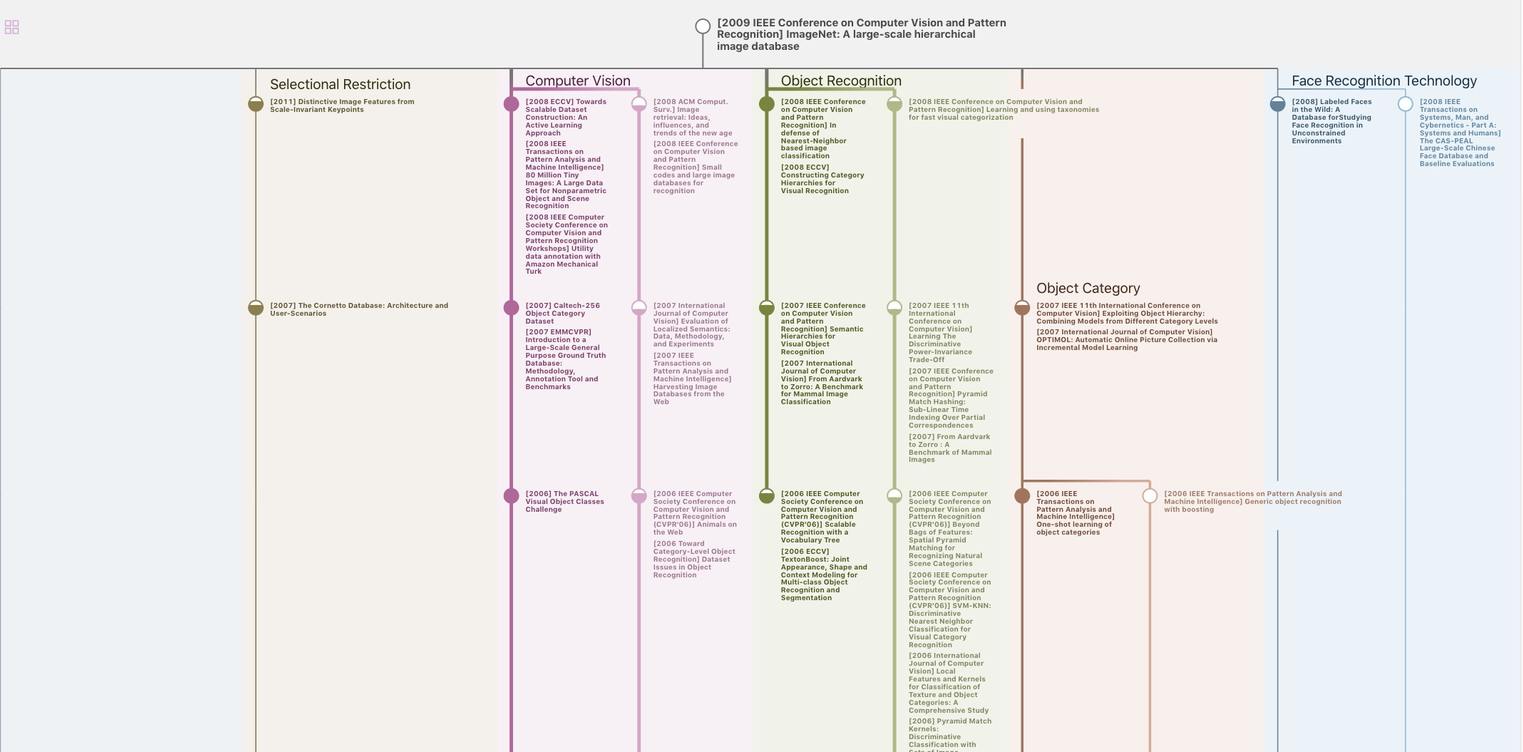
生成溯源树,研究论文发展脉络
Chat Paper
正在生成论文摘要