M4: Multi-generator, Multi-domain, and Multi-lingual Black-Box Machine-Generated Text Detection.
Computing Research Repository (CoRR)(2024)
Mohamed bin Zayed Univ Artificial Intelligence | Tech Univ Darmstadt
- Pretraining has recently greatly promoted the development of natural language processing (NLP)
- We show that M6 outperforms the baselines in multimodal downstream tasks, and the large M6 with 10 parameters can reach a better performance
- We propose a method called M6 that is able to process information of multiple modalities and perform both single-modal and cross-modal understanding and generation
- The model is scaled to large model with 10 billion parameters with sophisticated deployment, and the 10 -parameter M6-large is the largest pretrained model in Chinese
- Experimental results show that our proposed M6 outperforms the baseline in a number of downstream tasks concerning both single modality and multiple modalities We will continue the pretraining of extremely large models by increasing data to explore the limit of its performance
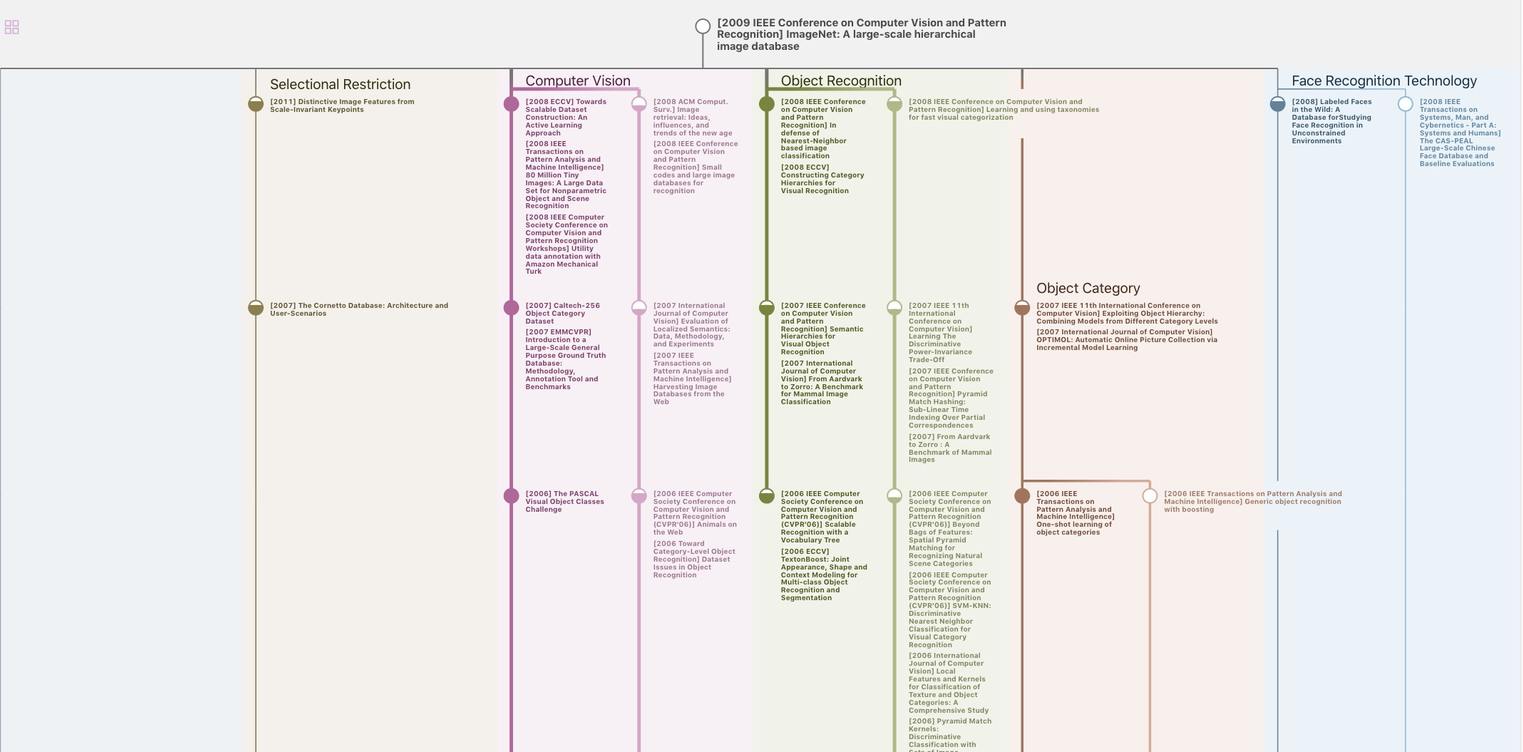
DNA-GPT: Divergent N-Gram Analysis for Training-Free Detection of GPT-Generated Text
被引用31
NBIAS: A Natural Language Processing Framework for Bias Identification in Text
被引用6
TOPFORMER: Topology-Aware Authorship Attribution of Deepfake Texts with Diverse Writing Styles
被引用1
Unlocking Bias Detection: Leveraging Transformer-Based Models for Content Analysis
被引用0
IMGTB: A Framework for Machine-Generated Text Detection Benchmarking
被引用1
TextMachina: Seamless Generation of Machine-Generated Text Datasets
被引用0
被引用0
被引用1
LLM-Detector: Improving AI-Generated Chinese Text Detection with Open-Source LLM Instruction Tuning
被引用1
M4GT-Bench: Evaluation Benchmark for Black-Box Machine-Generated Text Detection
被引用1
Stumbling Blocks: Stress Testing the Robustness of Machine-Generated Text Detectors under Attacks
被引用0
被引用0
Whose LLM is It Anyway? Linguistic Comparison and LLM Attribution for GPT-3.5, GPT-4 and Bard
被引用0
被引用0
A Survey of AI-generated Text Forensic Systems: Detection, Attribution, and Characterization
被引用2
Securing Large Language Models: Threats, Vulnerabilities and Responsible Practices
被引用2
被引用0
被引用0
Multilingual Large Language Model: A Survey of Resources, Taxonomy and Frontiers
被引用2
PetKaz at SemEval-2024 Task 8: Can Linguistics Capture the Specifics of LLM-generated Text?
被引用0