PESCO: Prompt-enhanced Self Contrastive Learning for Zero-shot Text Classification
PROCEEDINGS OF THE 61ST ANNUAL MEETING OF THE ASSOCIATION FOR COMPUTATIONAL LINGUISTICS (ACL 2023): LONG PAPERS, VOL 1(2023)
Abstract
We present PESCO, a novel contrastive learning framework that substantially improves the performance of zero-shot text classification. We formulate text classification as a neural text matching problem where each document is treated as a query, and the system learns the mapping from each query to the relevant class labels by (1) adding prompts to enhance label matching, and (2) using retrieved labels to enrich the training set in a self-training loop of contrastive learning. PESCO achieves state-of-the-art performance on four benchmark text classification datasets. On DBpedia, we achieve 98.5\% accuracy without any labeled data, which is close to the fully-supervised result. Extensive experiments and analyses show all the components of PESCO are necessary for improving the performance of zero-shot text classification.
MoreTranslated text
Key words
self contrastive learning,text,classification,prompt-enhanced,zero-shot
AI Read Science
Must-Reading Tree
Example
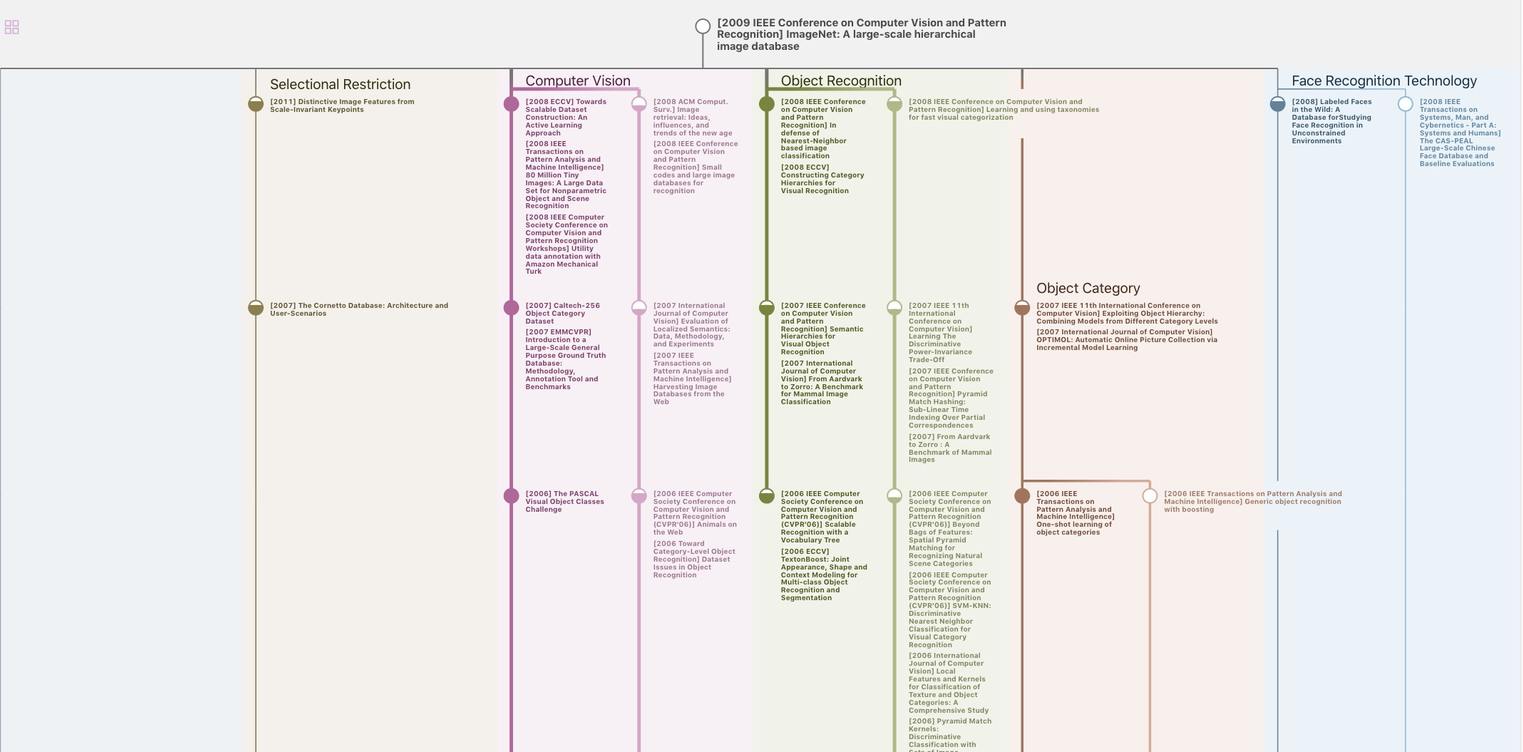
Generate MRT to find the research sequence of this paper
Chat Paper
Summary is being generated by the instructions you defined