GPT4Graph: Can Large Language Models Understand Graph Structured Data ? An Empirical Evaluation and Benchmarking
CoRR(2023)
摘要
Large language models~(LLM) like ChatGPT have become indispensable to artificial general intelligence~(AGI), demonstrating excellent performance in various natural language processing tasks. In the real world, graph data is ubiquitous and an essential part of AGI and prevails in domains like social network analysis, bioinformatics and recommender systems. The training corpus of large language models often includes some algorithmic components, which allows them to achieve certain effects on some graph data-related problems. However, there is still little research on their performance on a broader range of graph-structured data. In this study, we conduct an extensive investigation to assess the proficiency of LLMs in comprehending graph data, employing a diverse range of structural and semantic-related tasks. Our analysis encompasses 10 distinct tasks that evaluate the LLMs' capabilities in graph understanding. Through our study, we not only uncover the current limitations of language models in comprehending graph structures and performing associated reasoning tasks but also emphasize the necessity for further advancements and novel approaches to enhance their graph processing capabilities. Our findings contribute valuable insights towards bridging the gap between language models and graph understanding, paving the way for more effective graph mining and knowledge extraction.
更多查看译文
关键词
language,empirical evaluation
AI 理解论文
溯源树
样例
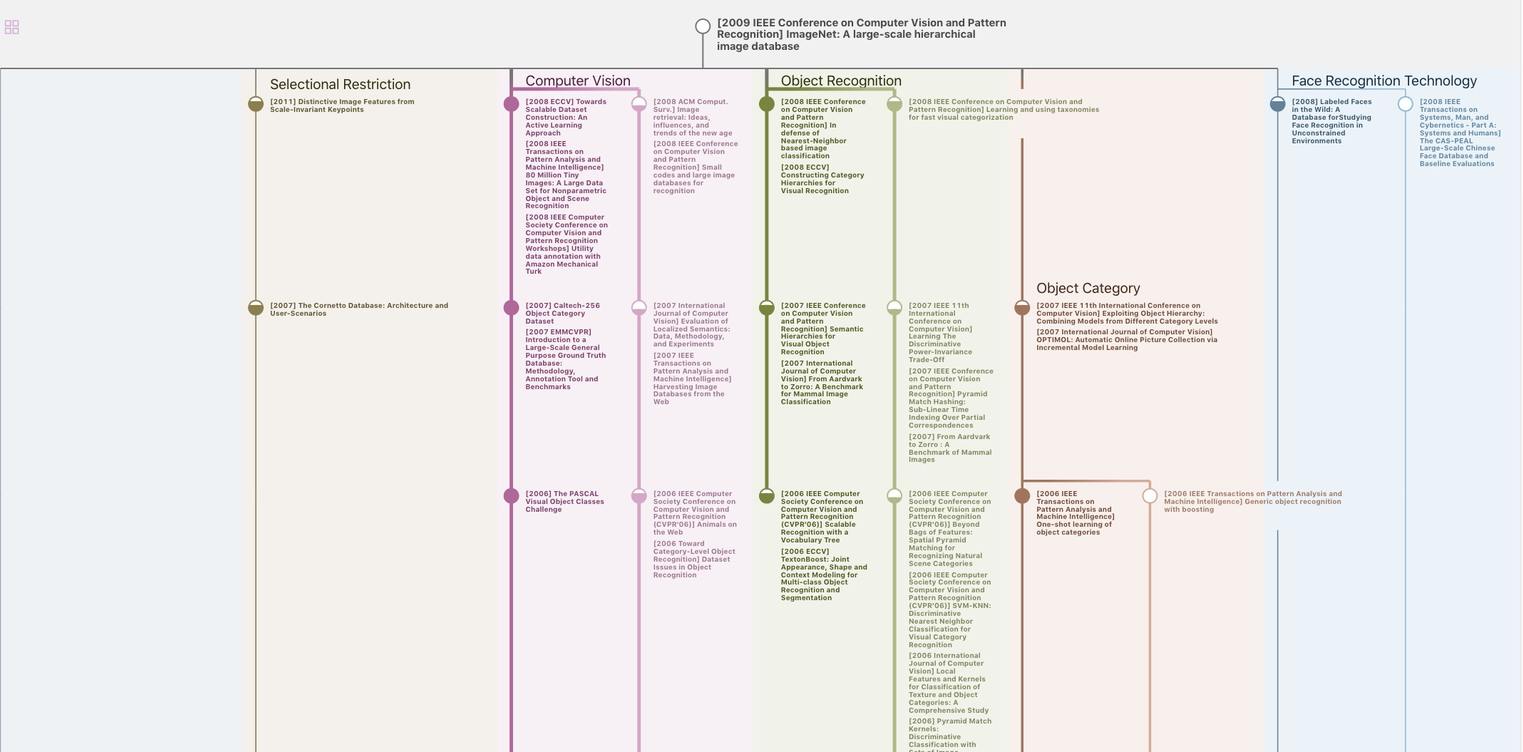
生成溯源树,研究论文发展脉络
Chat Paper
正在生成论文摘要