DynStatF: An Efficient Feature Fusion Strategy for LiDAR 3D Object Detection
CoRR(2023)
摘要
Augmenting LiDAR input with multiple previous frames provides richer semantic information and thus boosts performance in 3D object detection, However, crowded point clouds in multi-frames can hurt the precise position information due to the motion blur and inaccurate point projection. In this work, we propose a novel feature fusion strategy, DynStaF (Dynamic-Static Fusion), which enhances the rich semantic information provided by the multi-frame (dynamic branch) with the accurate location information from the current single-frame (static branch). To effectively extract and aggregate complimentary features, DynStaF contains two modules, Neighborhood Cross Attention (NCA) and Dynamic-Static Interaction (DSI), operating through a dual pathway architecture. NCA takes the features in the static branch as queries and the features in the dynamic branch as keys (values). When computing the attention, we address the sparsity of point clouds and take only neighborhood positions into consideration. NCA fuses two features at different feature map scales, followed by DSI providing the comprehensive interaction. To analyze our proposed strategy DynStaF, we conduct extensive experiments on the nuScenes dataset. On the test set, DynStaF increases the performance of PointPillars in NDS by a large margin from 57.7% to 61.6%. When combined with CenterPoint, our framework achieves 61.0% mAP and 67.7% NDS, leading to state-of-the-art performance without bells and whistles.
更多查看译文
关键词
accurate location information,complimentary features,comprehensive interaction,crowded point clouds,different feature map scales,DSI,dual pathway architecture,dynamic branch,Dynamic-Static Fusion,Dynamic-Static Interaction,DynStatF,efficient feature fusion strategy,inaccurate point projection,LiDAR 3D object detection,LiDAR input,motion blur,multiframes,multiple previous frames,NCA fuses,Neighborhood Cross Attention,neighborhood positions,precise position information,rich semantic information,richer semantic information,static branch,strategy DynStaF
AI 理解论文
溯源树
样例
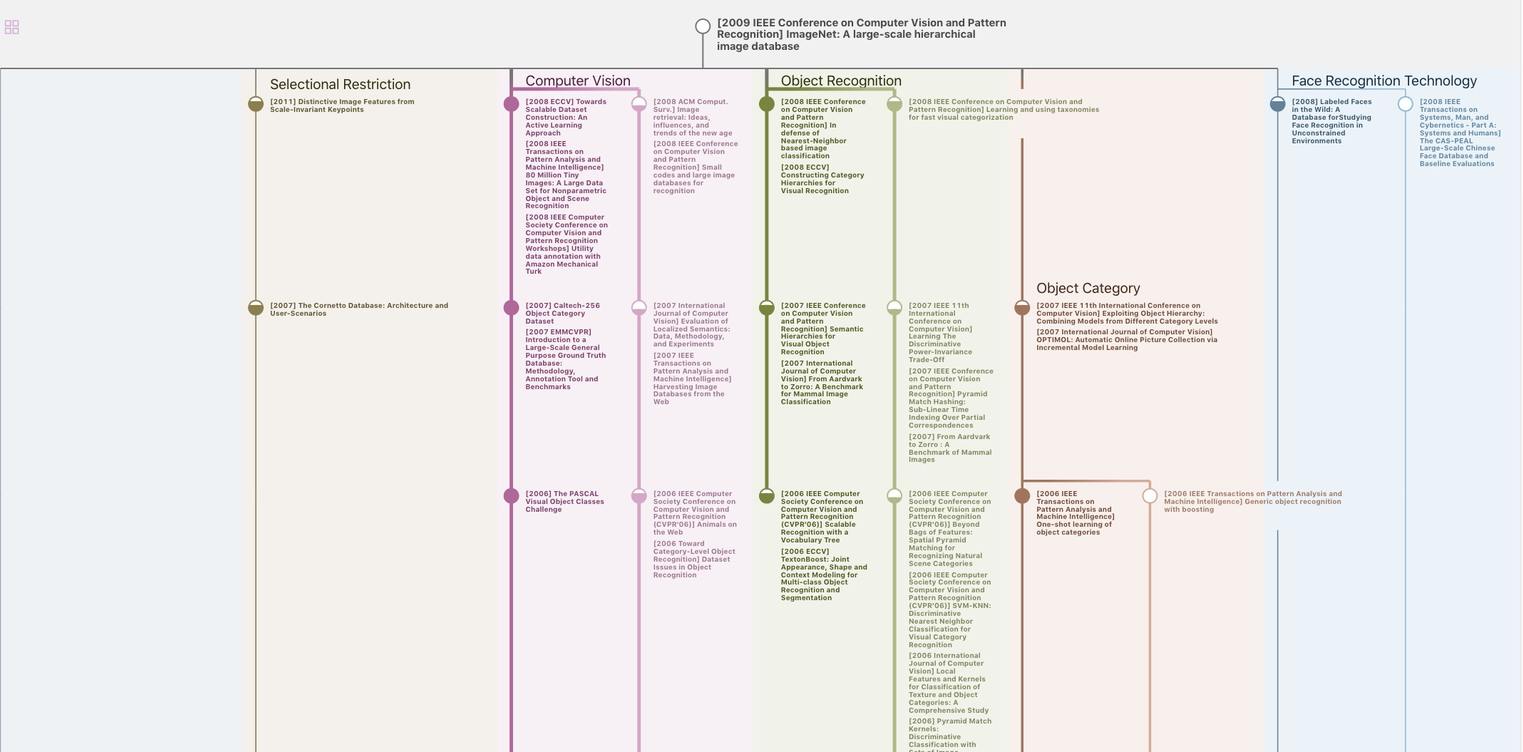
生成溯源树,研究论文发展脉络
Chat Paper
正在生成论文摘要