A Traffic Parameter Extraction Model Using Small Vehicle Detection and Tracking in Low-Brightness Aerial Images
Sustainability(2023)
摘要
It is still a challenge to detect small-size vehicles from a drone perspective, particularly under low-brightness conditions. In this context, a YOLOX-IM-DeepSort model was proposed, which improved the object detection performance in low-brightness conditions accurately and efficiently. At the stage of object detection, this model incorporates the data enhancement algorithm as well as an ultra-lightweight subspace attention module, and optimizes the number of detection heads and the loss function. Then, the ablation experiment was conducted and the analysis results showed that the YOLOX-IM model has better mAP than the baseline model YOLOX-s for multi-scale object detection. At the stage of object tracking, the DeepSort object-tracking algorithm is connected to the YOLOX-IM model, which can extract vehicle classification data, vehicle trajectory, and vehicle speed. Then, the VisDrone2021 dataset was adopted to verify the object-detection and tracking performance of the proposed model, and comparison experiment results showed that the average vehicle detection accuracy is 85.00% and the average vehicle tracking accuracy is 71.30% at various brightness levels, both of which are better than those of CenterNet, YOLOv3, FasterR-CNN, and CascadeR-CNN. Next, a field experiment using an in-vehicle global navigation satellite system and a DJI Phantom 4 RTK drone was conducted in Tianjin, China, and 12 control experimental scenarios with different drone flight heights and vehicle speeds were designed to analyze the effect of drone flight altitude on speed extraction accuracy. Finally, the conclusions and discussions were presented.
更多查看译文
关键词
traffic information and control,vehicle detection and tracking,unmanned aerial vehicles,low brightness images
AI 理解论文
溯源树
样例
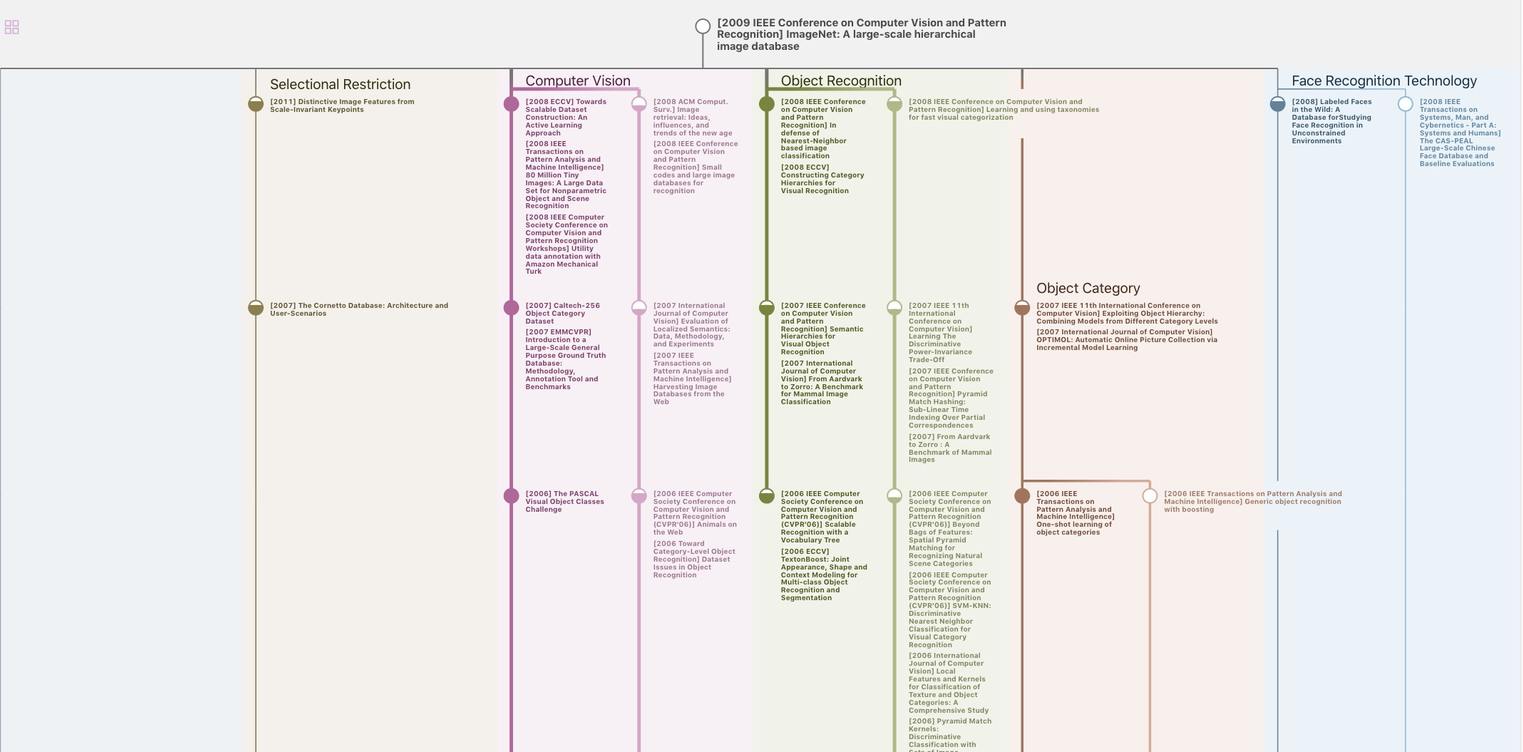
生成溯源树,研究论文发展脉络
Chat Paper
正在生成论文摘要