Sample and Predict Your Latent: Modality-free Sequential Disentanglement via Contrastive Estimation
CoRR(2023)
摘要
Unsupervised disentanglement is a long-standing challenge in representation learning. Recently, self-supervised techniques achieved impressive results in the sequential setting, where data is time-dependent. However, the latter methods employ modality-based data augmentations and random sampling or solve auxiliary tasks. In this work, we propose to avoid that by generating, sampling, and comparing empirical distributions from the underlying variational model. Unlike existing work, we introduce a self-supervised sequential disentanglement framework based on contrastive estimation with no external signals, while using common batch sizes and samples from the latent space itself. In practice, we propose a unified, efficient, and easy-to-code sampling strategy for semantically similar and dissimilar views of the data. We evaluate our approach on video, audio, and time series benchmarks. Our method presents state-of-the-art results in comparison to existing techniques. The code is available at https://github.com/azencot-group/SPYL.
更多查看译文
关键词
latent,sequential,predict,modality-free
AI 理解论文
溯源树
样例
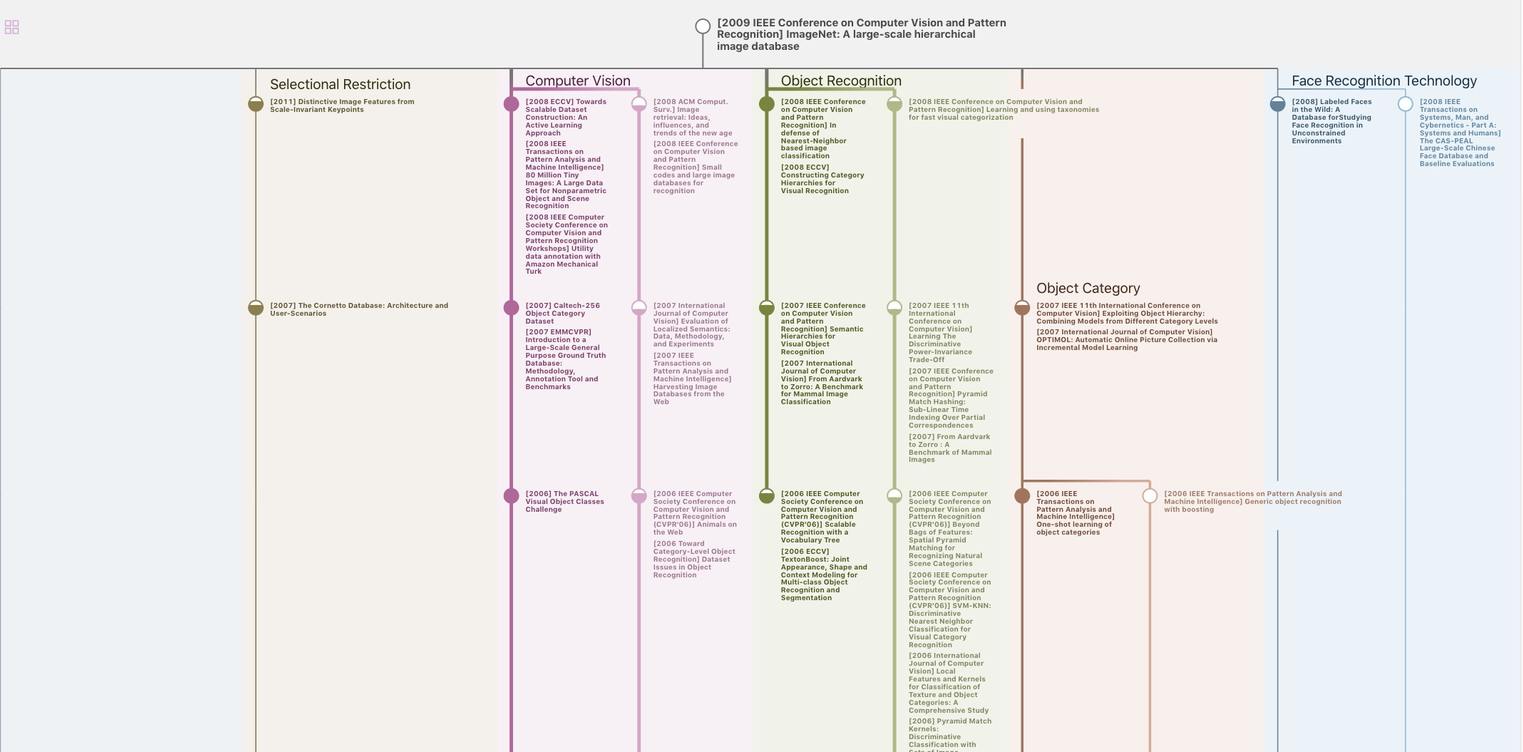
生成溯源树,研究论文发展脉络
Chat Paper
正在生成论文摘要