Explainability Techniques for Chemical Language Models
CoRR(2023)
摘要
Explainability techniques are crucial in gaining insights into the reasons behind the predictions of deep learning models, which have not yet been applied to chemical language models. We propose an explainable AI technique that attributes the importance of individual atoms towards the predictions made by these models. Our method backpropagates the relevance information towards the chemical input string and visualizes the importance of individual atoms. We focus on self-attention Transformers operating on molecular string representations and leverage a pretrained encoder for finetuning. We showcase the method by predicting and visualizing solubility in water and organic solvents. We achieve competitive model performance while obtaining interpretable predictions, which we use to inspect the pretrained model.
更多查看译文
关键词
chemical,models,language
AI 理解论文
溯源树
样例
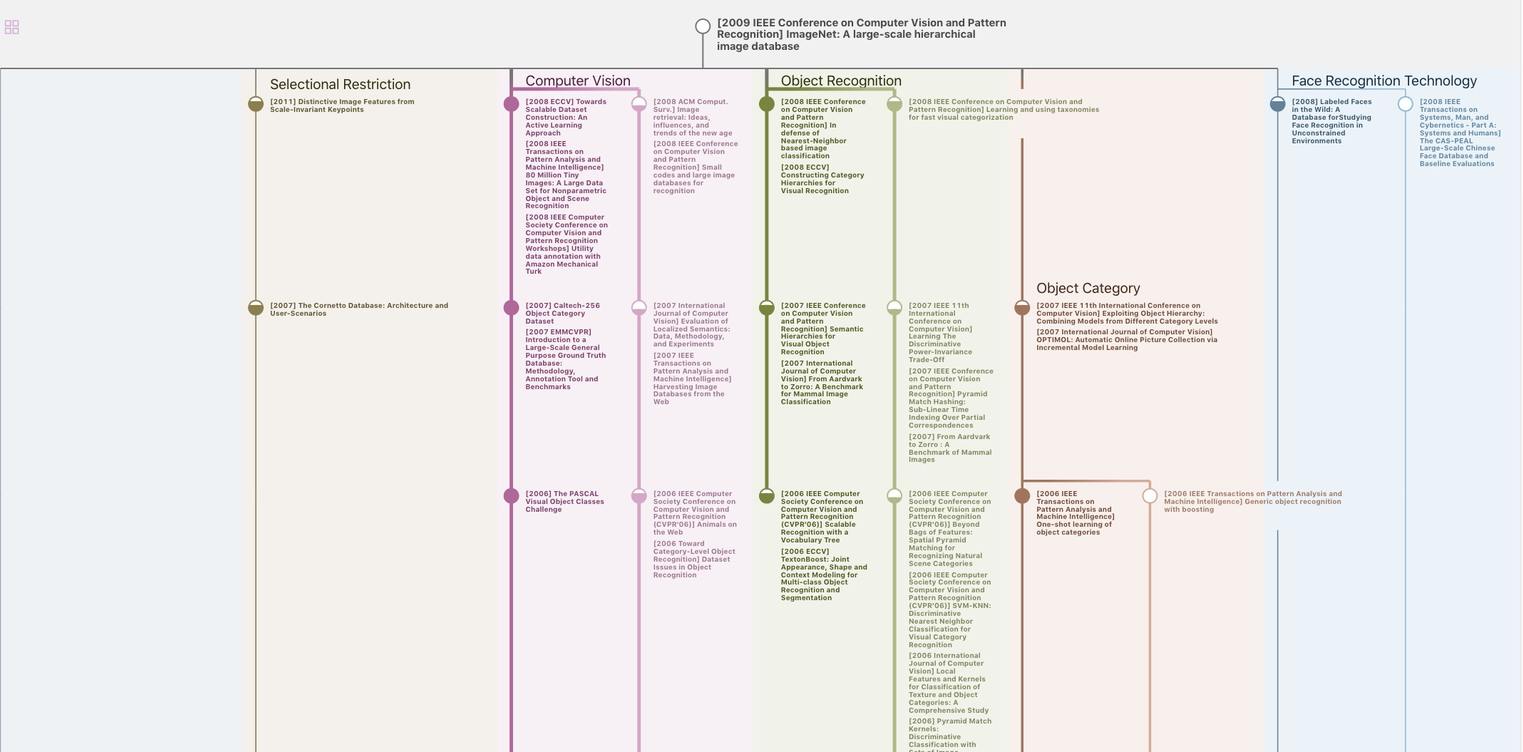
生成溯源树,研究论文发展脉络
Chat Paper
正在生成论文摘要