Gaussian Processes with State-Dependent Noise for Stochastic Control
CoRR(2023)
摘要
This paper considers a stochastic control framework, in which the residual model uncertainty of the dynamical system is learned using a Gaussian Process (GP). In the proposed formulation, the residual model uncertainty consists of a nonlinear function and state-dependent noise. The proposed formulation uses a posterior-GP to approximate the residual model uncertainty and a prior-GP to account for state-dependent noise. The two GPs are interdependent and are thus learned jointly using an iterative algorithm. Theoretical properties of the iterative algorithm are established. Advantages of the proposed state-dependent formulation include (i) faster convergence of the GP estimate to the unknown function as the GP learns which data samples are more trustworthy and (ii) an accurate estimate of state-dependent noise, which can, e.g., be useful for a controller or decision-maker to determine the uncertainty of an action. Simulation studies highlight these two advantages.
更多查看译文
关键词
gaussian processes,stochastic,noise,control,state-dependent
AI 理解论文
溯源树
样例
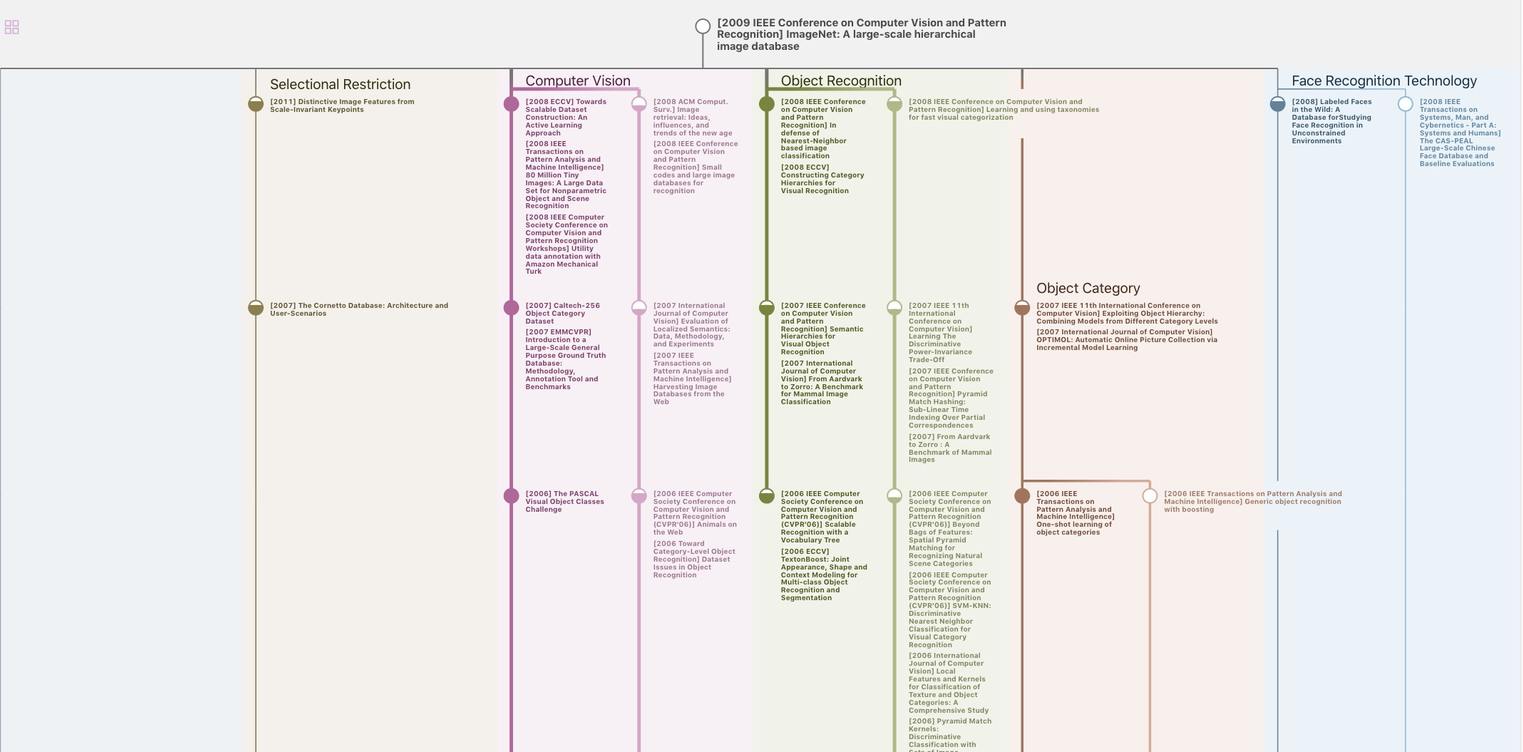
生成溯源树,研究论文发展脉络
Chat Paper
正在生成论文摘要