Multi-Modal Interaction-Aware Motion Prediction at Unsignalized Intersections
IEEE TRANSACTIONS ON INTELLIGENT VEHICLES(2023)
摘要
Autonomous vehicle technologies have evolved quickly over the last few years, with safety being one of the key requirements for their full deployment. However, ensuring their safety while navigating through highly interactive and complex scenarios remains a critical challenge. To tackle this problem, intention estimation and motion prediction are fundamental. In this work, a method to infer the intentions, based on a Dynamic Bayesian Network (DBN), and predict the motion, using Markov Chains, of the nearby vehicles at unsignalized intersections is proposed. This approach considers all possible corridors of the surrounding traffic participants and takes into account their interactions to infer the probabilities of stopping or crossing the intersection, as well as the probability of being in each of the possible navigable corridors. To achieve this, the DBN is used to model the relationships between the observed states and the unobserved intentions of the nearby agents. The Markov Chain model, obtained from a kinematic model, is used to predict the future motions of the vehicles, taking into account their current state, their inferred intentions, and the uncertainty associated with the prediction. The resulting multi-modal motion predictions are sent to the ego vehicle to navigate through the scene. The proposed method is evaluated in 6 real situations extracted from publicly available datasets and is compared with a model-based and a learn-based baseline models. The results showed that the proposed method outperformed both baselines in terms of accuracy considering the metrics ADE and FDE.
更多查看译文
关键词
Autonomous vehicles,motion prediction,intention-detection,interaction-aware,intersection
AI 理解论文
溯源树
样例
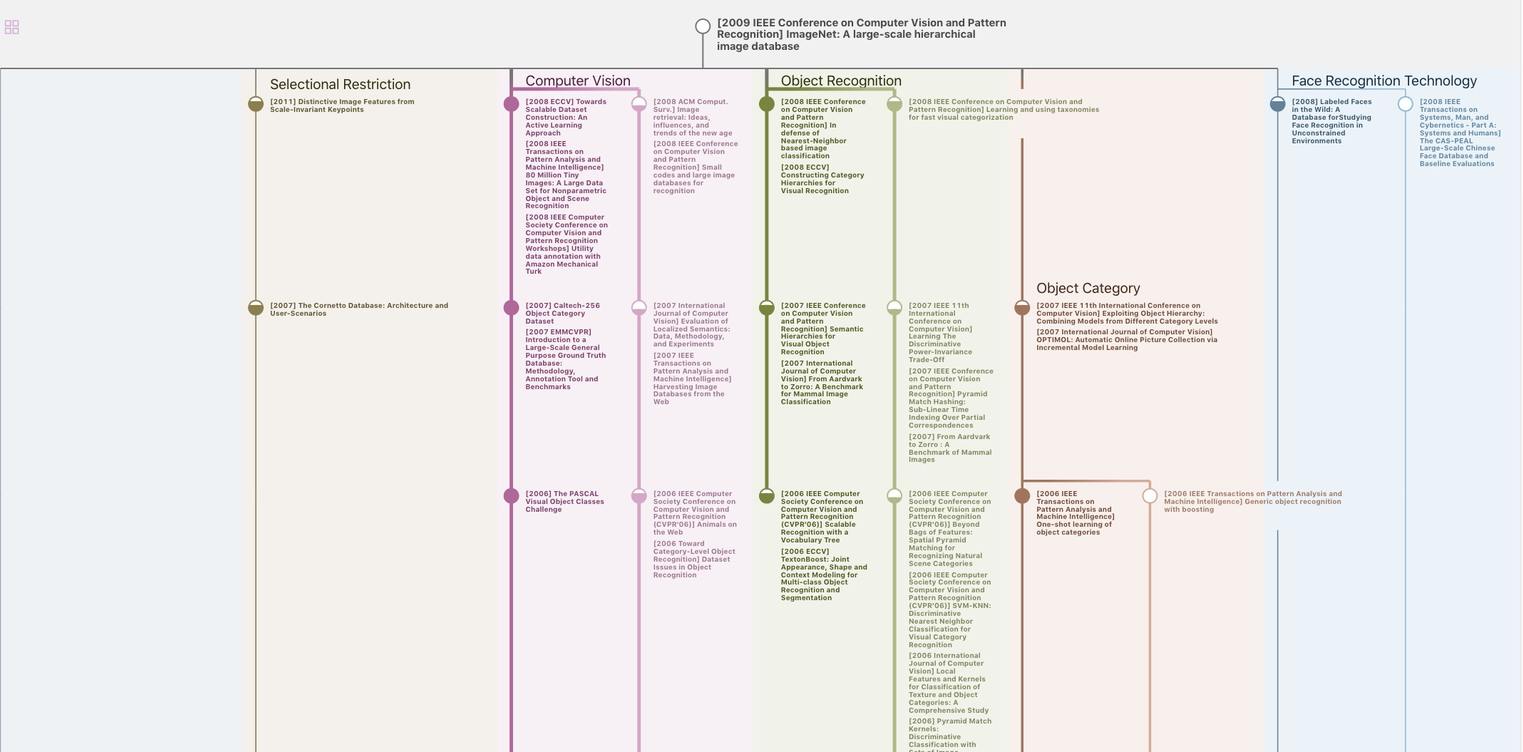
生成溯源树,研究论文发展脉络
Chat Paper
正在生成论文摘要