The Impact of Convolutional Neural Network Parameters in the Binary Classification of Mammograms
2022 24th International Symposium on Symbolic and Numeric Algorithms for Scientific Computing (SYNASC)(2022)
摘要
Breast cancer is the most commonly diagnosed type of cancer. It is essential to classify patients as quickly as possible into groups with a high or low risk of cancer, to provide adequate treatment. This paper aims to address the impact of the parameters of convolutional neural networks in the binary classification of mammograms. In this paper, we treat two types of binary classification, namely: classification between normal and abnormal tissues, respectively classification between benign and malignant tumors. In the analysis, we investigate the correlation and impact of batch size and learning rate in increasing the performance of the proposed model. Following the experiments on the MIAS dataset, we concluded that for the treated problems, it is appropriate to choose a learning rate lower than 0.001. For the classification of tissues (normal/abnormal), we obtained the fact that training the model on a batch size of 32 brings the best results, namely an accuracy of 0.67, and for the classification of tumors (benign/malignant), it is more appropriate to use a batch size of 8, for which we obtained an accuracy of 0.63. For the best results configurations, we continued the experiments by investigating the impact of data augmentation. We have increased the number of training data by applying horizontal flip and rotation operations. Following these attempts, we noticed an improvement only for the tissue classification, for which we obtained an accuracy of 0.70.
更多查看译文
关键词
Breast Cancer,Mammogram Classification,Convolutional Neural Network (CNN)
AI 理解论文
溯源树
样例
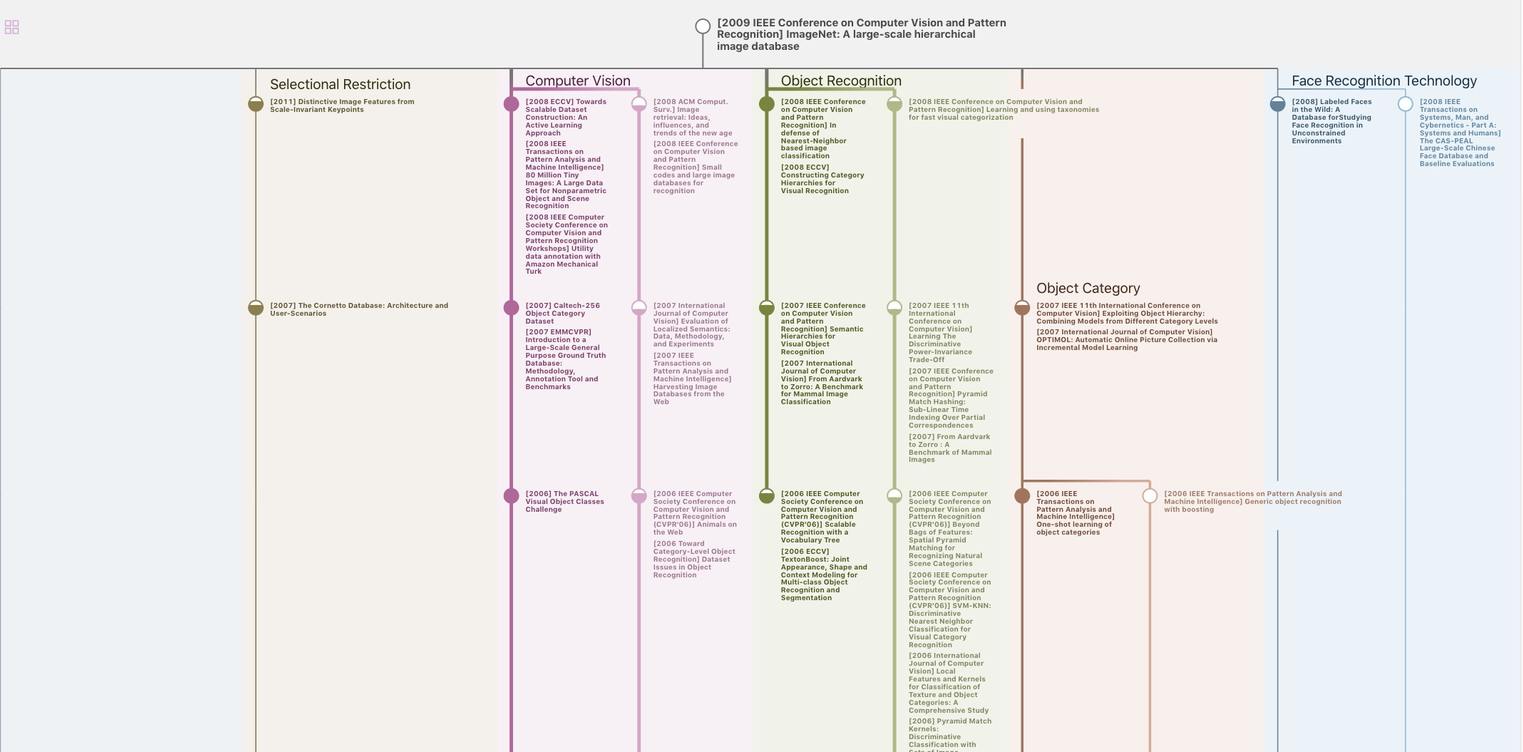
生成溯源树,研究论文发展脉络
Chat Paper
正在生成论文摘要