Lithium-ion Battery RUL Prediction Using ANN and Comparison to Alternative Models
2022 IEEE North Karnataka Subsection Flagship International Conference (NKCon)(2022)
摘要
For successful condition-based maintenance to in-crease reliability and lower total maintenance costs, accurate equipment remaining usable life forecast is essential. With the ever increasing demand of the Electric Vehicles in today's sce-nario It's crucial to have a predictive algorithm for the battery's remaining useful life. This will have a direct impact on customer experience, thus directly affecting the market of the Electric Vehicle. An artificial neural network (ANN) based technique is suggested for more precise remaining useful life prediction of Lithium-Ion batteries subject to condition monitoring. The life expectancy is the result of the ANN model, which uses the capacity attribute as a target against various measurement values as inputs. The suggested ANN technique is compared to other ways in a comparison study, and the results demonstrate that the proposed method has an advantage in terms of more precisely forecasting remaining useful life. The accuracy achieved by us in the using the ANN model is more than 99 percent. This study intends to increase useful life prediction accuracy, which will benefit the electric vehicle industry. The Electric Vehicle being more green than the Fuel Vehicles will also help preserving the environment.
更多查看译文
关键词
Lithium-ion battery (LIB),regression,remaining usable life,critical point (RUL)
AI 理解论文
溯源树
样例
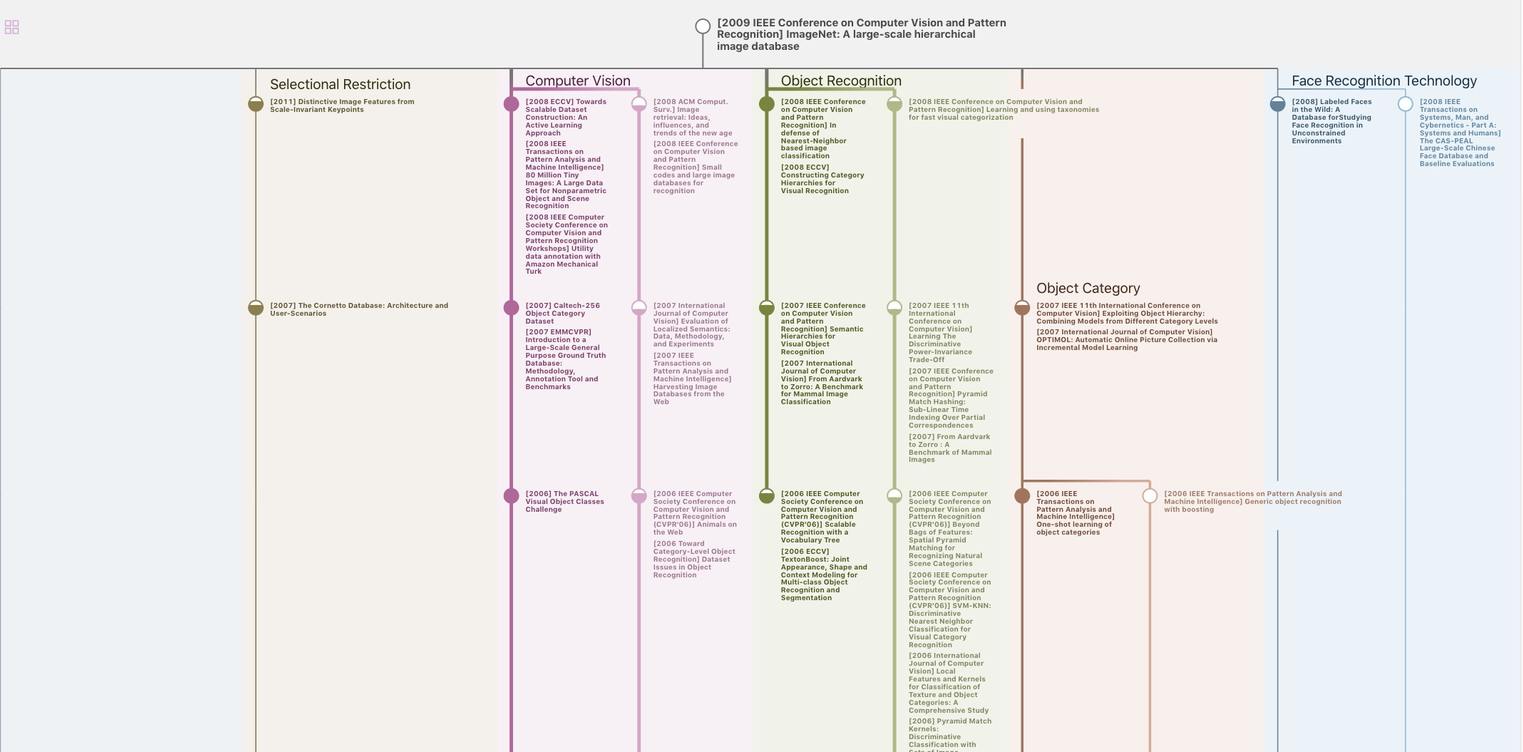
生成溯源树,研究论文发展脉络
Chat Paper
正在生成论文摘要