Feature Selection Optimization for Heart Rate Variability
2023 11th International Symposium on Digital Forensics and Security (ISDFS)(2023)
摘要
Feature selection optimization has gained attention since it helps extract good insights about a given subject. It is commonly used to reduce the dimensionality of the problem and to identify the best combination of features with aim of improving the prediction or classification model performance. Thereby, the present study intended to determine the best subset of features to classify the drowsiness state, into awake and drowsy, considering the heart rate variability. This approach is a gap in the literature since the studies available only classify the drowsy state without understanding which features have more impact on the transition from awake to drowsy. Single-objective optimization was performed using the genetic algorithm, with the purpose of maximizing accuracy, F1 score, and recall. Different machine learning algorithms were computed, and comparisons were made. Therefore, logistic regression and the extra tree algorithms had the best results for all the evaluation metrics. The best results were achieved with fewer heart rate variability features than initially introduced. With the proposed methodology it was possible to identify 13 features that occurred most to classify the drowsiness state. Where from the time, frequency, and nonlinear domains there were 6, 5, and 2 features, respectively. As future work, time series must be implemented to verify if it is possible to predict the drowsiness state and visualize trends and seasonality.
更多查看译文
关键词
artificial intelligence,drowsiness at the wheel,heart rate variability,optimization,accuracy,recall
AI 理解论文
溯源树
样例
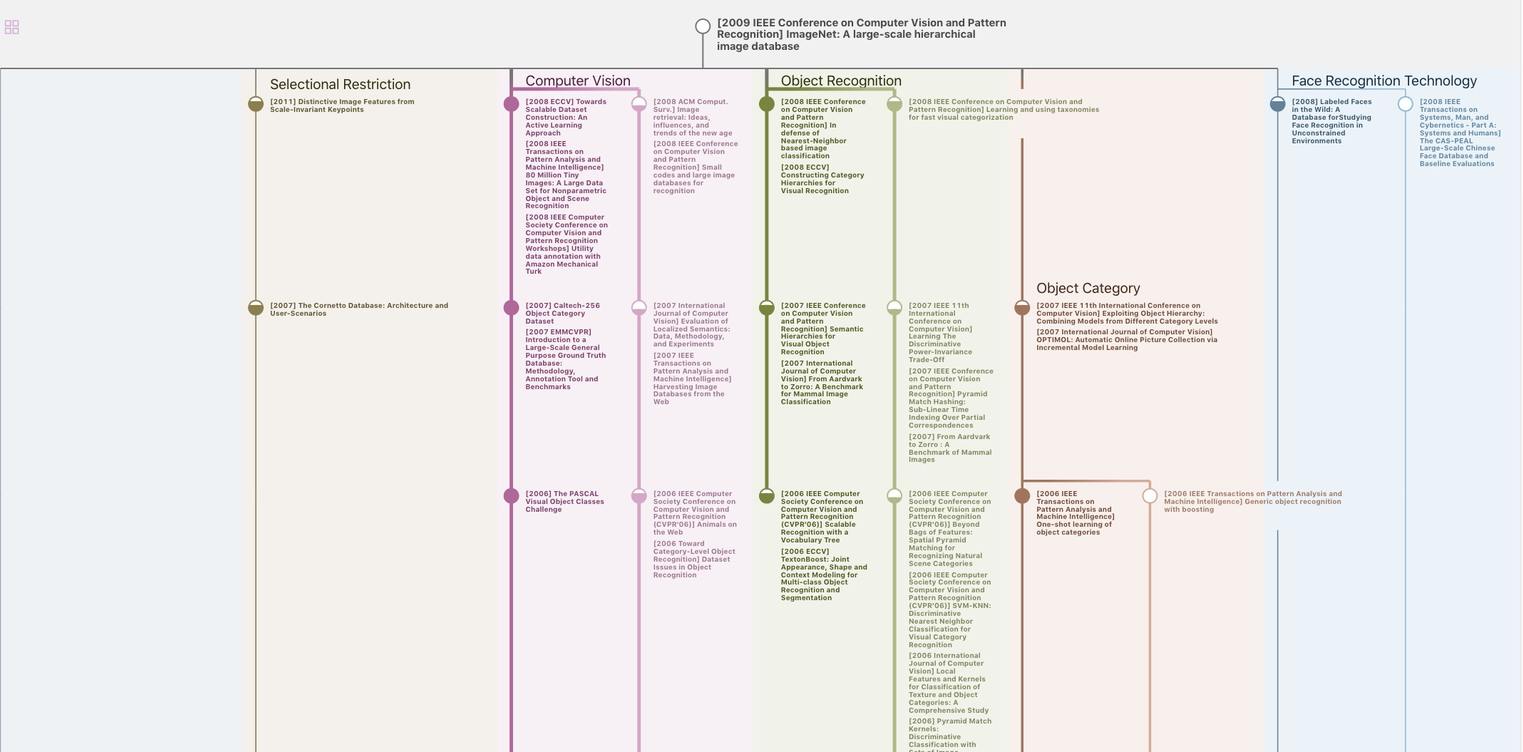
生成溯源树,研究论文发展脉络
Chat Paper
正在生成论文摘要