Using Hypothesis-Led Machine Learning and Hierarchical Cluster Analysis to Identify Disease Pathways Prior to Dementia: Longitudinal Cohort Study
Journal of Medical Internet Research(2023)
摘要
Background: Dementia development is a complex process in which the occurrence and sequential relationships of different diseases or conditions may construct specific patterns leading to incident dementia.Objective: This study aimed to identify patterns of disease or symptom clusters and their sequences prior to incident dementia using a novel approach incorporating machine learning methods. Methods: Using Taiwan's National Health Insurance Research Database, data from 15,700 older people with dementia and 15,700 nondementia controls matched on age, sex, and index year (n=10,466, 67% for the training data set and n=5234, 33% for the testing data set) were retrieved for analysis. Using machine learning methods to capture specific hierarchical disease triplet clusters prior to dementia, we designed a study algorithm with four steps: (1) data preprocessing, (2) disease or symptom pathway selection, (3) model construction and optimization, and (4) data visualization. Results: Among 15,700 identified older people with dementia, 10,466 and 5234 subjects were randomly assigned to the training and testing data sets, and 6215 hierarchical disease triplet clusters with positive correlations with dementia onset were identified. We subsequently generated 19,438 features to construct prediction models, and the model with the best performance was support vector machine (SVM) with the by-group LASSO (least absolute shrinkage and selection operator) regression method (total corresponding features=2513; accuracy=0.615; sensitivity=0.607; specificity=0.622; positive predictive value=0.612; negative predictive value=0.619; area under the curve=0.639). In total, this study captured 49 hierarchical disease triplet clusters related to dementia development, and the most characteristic patterns leading to incident dementia started with cardiovascular conditions (mainly hypertension), cerebrovascular disease, mobility disorders, or infections, followed by neuropsychiatric conditions.Conclusions: Dementia development in the real world is an intricate process involving various diseases or conditions, their co-occurrence, and sequential relationships. Using a machine learning approach, we identified 49 hierarchical disease triplet clusters with leading roles (cardio-or cerebrovascular disease) and supporting roles (mental conditions, locomotion difficulties, infections, and nonspecific neurological conditions) in dementia development. Further studies using data from other countries are needed to validate the prediction algorithms for dementia development, allowing the development of comprehensive strategies to prevent or care for dementia in the real world.
更多查看译文
关键词
dementia,machine learning,cluster analysis,disease,condition,symptoms,data,data set,cardiovascular,neuropsychiatric,infection,mobility,mental conditions,development
AI 理解论文
溯源树
样例
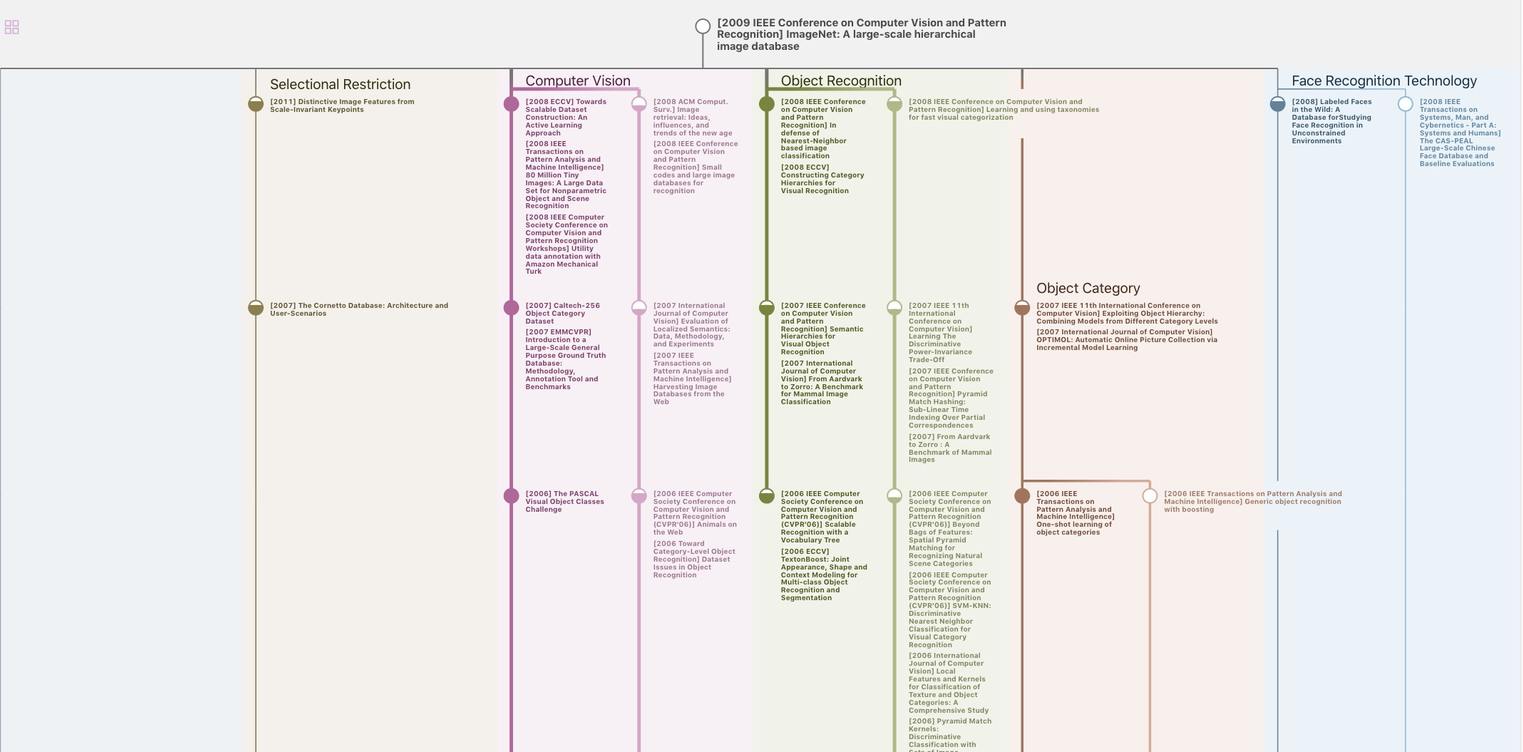
生成溯源树,研究论文发展脉络
Chat Paper
正在生成论文摘要