An AI-Ready Multiplex Staining Dataset for Reproducible and Accurate Characterization of Tumor Immune Microenvironment
MEDICAL IMAGE COMPUTING AND COMPUTER ASSISTED INTERVENTION, MICCAI 2023, PT VI(2023)
摘要
We introduce a new AI-ready computational pathology dataset containing restained and co-registered digitized images from eight head-and-neck squamous cell carcinoma patients. Specifically, the same tumor sections were stained with the expensive multiplex immunofluorescence (mIF) assay first and then restained with cheaper multiplex immunohistochemistry (mIHC). This is a first public dataset that demonstrates the equivalence of these two staining methods which in turn allows several use cases; due to the equivalence, our cheaper mIHC staining protocol can offset the need for expensive mIF staining/scanning which requires highly-skilled lab technicians. As opposed to subjective and error-prone immune cell annotations from individual pathologists (disagreement > 50%) to drive SOTA deep learning approaches, this dataset provides objective immune and tumor cell annotations via mIF/mIHC restaining for more reproducible and accurate characterization of tumor immune microenvironment (e.g. for immunotherapy). We demonstrate the effectiveness of this dataset in three use cases: (1) IHC quantification of CD3/CD8 tumor-infiltrating lymphocytes via style transfer, (2) virtual translation of cheap mIHC stains to more expensive mIF stains, and (3) virtual tumor/immune cellular phenotyping on standard hematoxylin images. The dataset is available at https://github. com/nadeemlab/DeepLIIF.
更多查看译文
关键词
multiplex immuofluorescence,multiplex immunohistochemistry,tumor microenvironment,virtual stain-to-stain translation
AI 理解论文
溯源树
样例
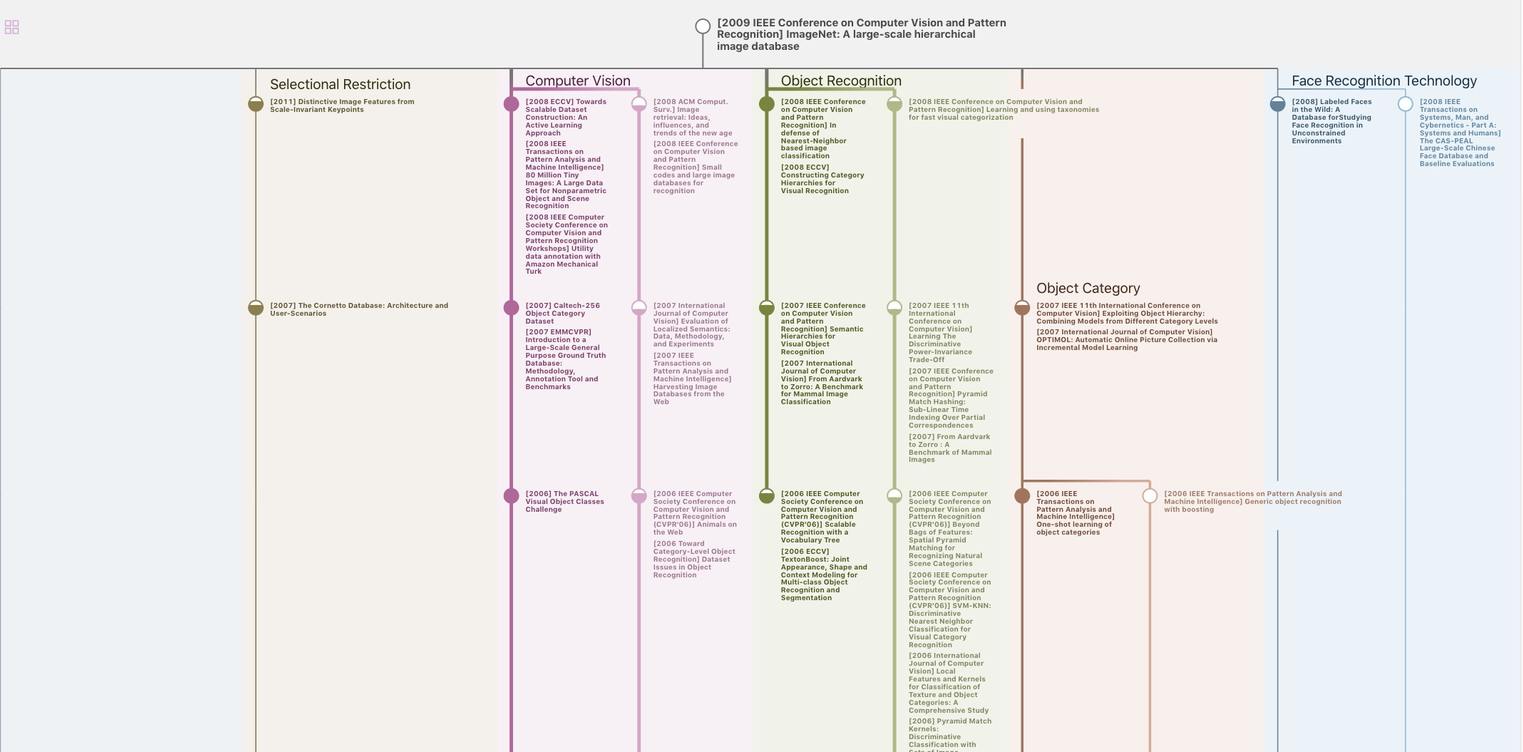
生成溯源树,研究论文发展脉络
Chat Paper
正在生成论文摘要