Proper network randomization is key to assessing social balance
CoRR(2023)
摘要
Studying significant network patterns, known as graphlets (or motifs), has been a popular approach to understand the underlying organizing principles of complex networks. Statistical significance is routinely assessed by comparing to null models that randomize the connections while preserving some key aspects of the data. However, in signed networks, capturing both positive (friendly) and negative (hostile) relations, the results have been controversial and also at odds with the classical theory of structural balance. We show that this is largely due to the fact that large-scale signed networks exhibit a poor correlation between the number of positive and negative ties of each node. As a solution, here we propose a null model based on the maximum entropy framework that preserves both the signed degrees and the network topology (STP randomization). With STP randomization the results change qualitatively and most social networks consistently satisfy strong structural balance, both at the level of triangles and larger graphlets. We propose a potential underlying mechanism of the observed patterns in signed social networks and outline further applications of STP randomization.
更多查看译文
关键词
proper network randomization,balance,social
AI 理解论文
溯源树
样例
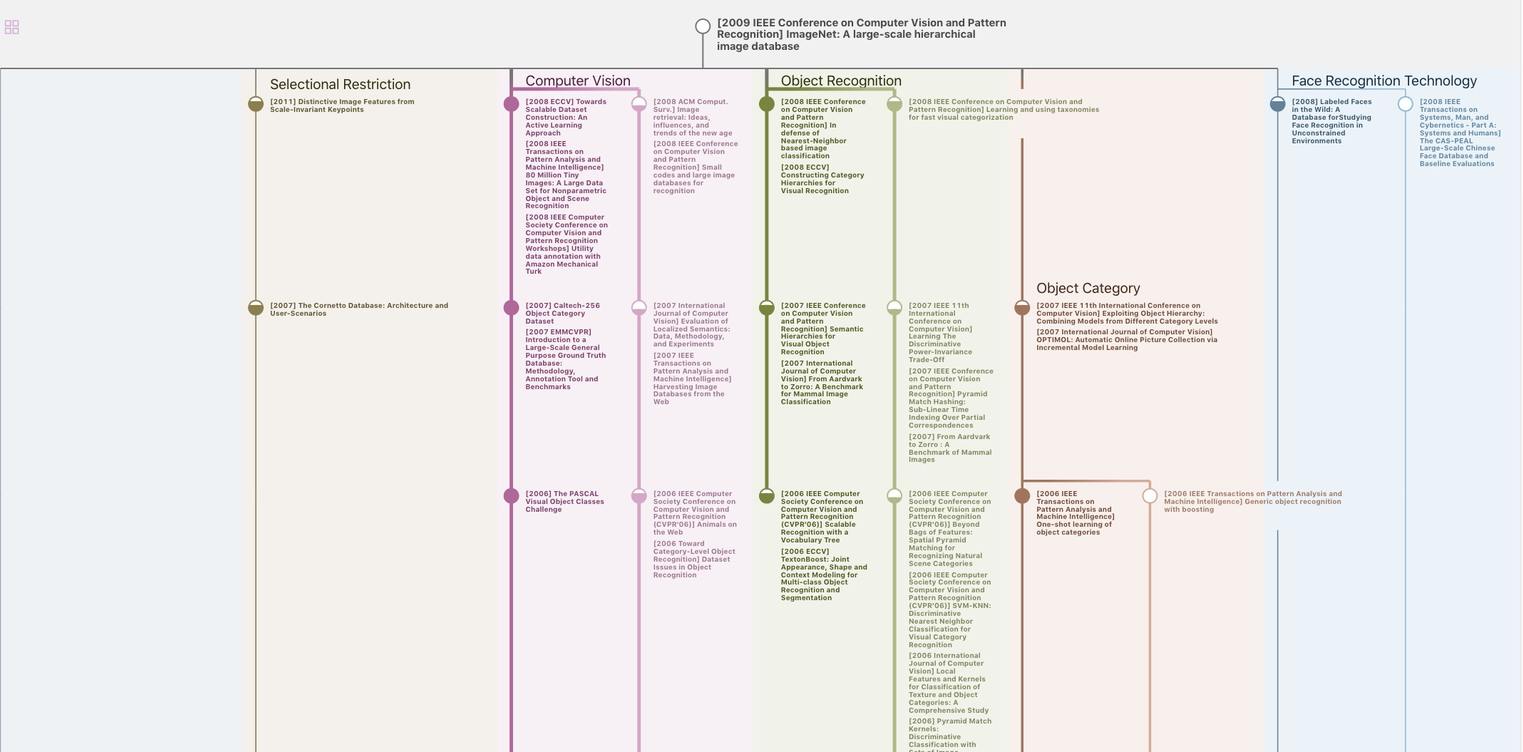
生成溯源树,研究论文发展脉络
Chat Paper
正在生成论文摘要