DAFTA: Distributed Architecture for Fusion-Transformer training Acceleration.
BiDEDE '23: Proceedings of the International Workshop on Big Data in Emergent Distributed Environments(2023)
摘要
Multi-modal data fusion transformer is a deep learning model that integrates information from multiple modalities, such as text, image, audio, etc., to improve performance in various tasks, especially in the remote sensing domain. Recent efforts leverage hyperspectral imaging (HSI) and LiDAR sensors and their complementary information about the target. Remote sensing image classification is inherently a transductive learning problem, requiring repeated model training (e.g., environment monitoring applications where changes occur on a daily or weekly basis). Hyperspectral data is typically high dimensional and massive, and model training can take an impractically long time (several days to weeks). By reducing training time, it becomes possible to process larger amounts of data and develop more efficient and accurate models. In this paper, we introduce a novel distributed training architecture, DAFTA, which addresses the challenges associated with the processing of large multi-modal remote sensing data. DAFTA is enabled to handle any combination of remote sensing modalities. Additionally, we leverage the similarity of the feature space to optimize the training process and achieve the training with a reduced data set which is equivalent to a complete data set. The proposed approach provides a systematic and efficient method for managing large remote sensing data and enables accurate and timely insights for various applications such as agriculture and infrastructure development. We conduct experiments on two real-world data sets of remotely-sensed images. Our results validate the efficacy of the proposed distributed training approach by achieving a speed-up in the range of 5x without compromising the accuracy, thus making it more practical for real-world applications.
更多查看译文
AI 理解论文
溯源树
样例
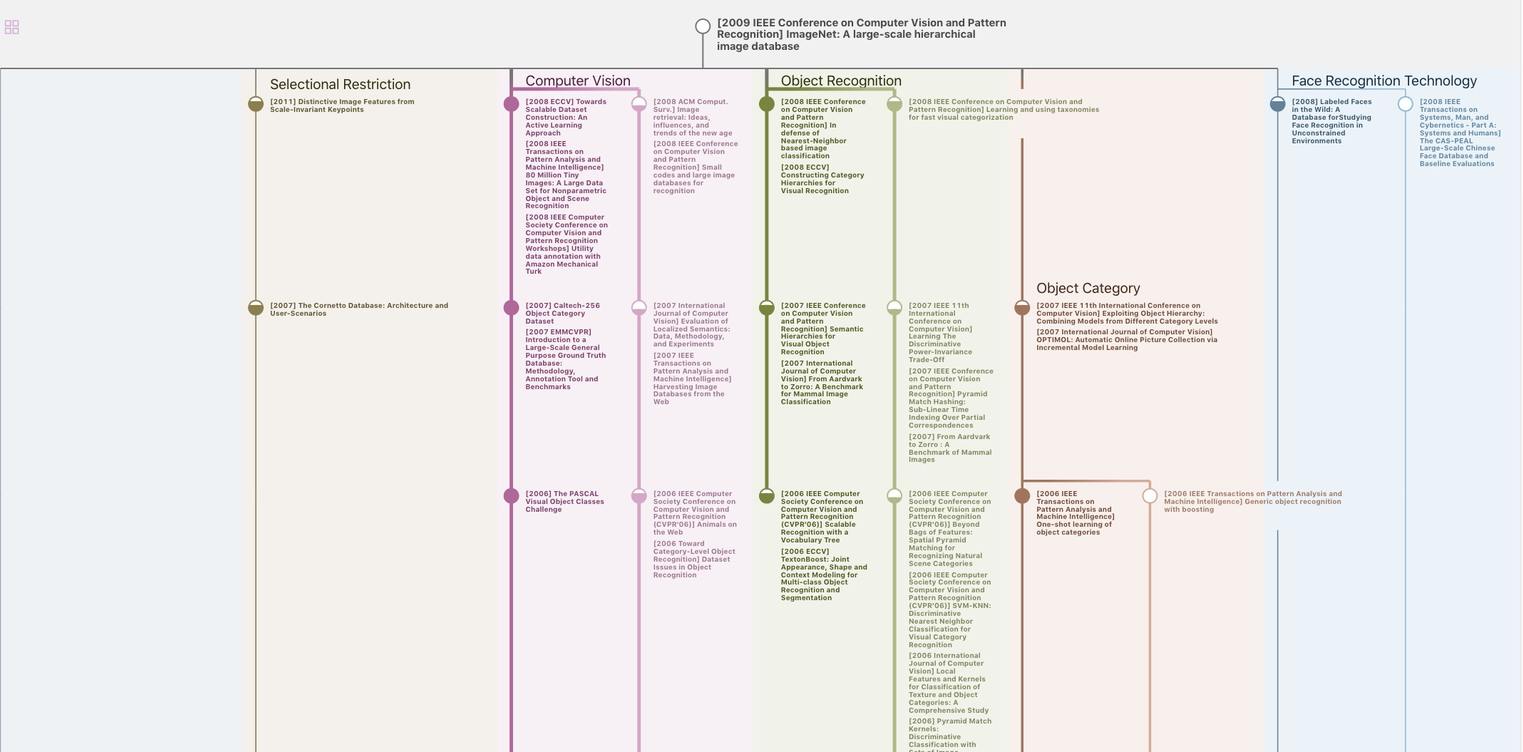
生成溯源树,研究论文发展脉络
Chat Paper
正在生成论文摘要