Multi-Rate Sensor Fusion for Unconstrained Near-Eye Gaze Estimation
ETRA '23: Proceedings of the 2023 Symposium on Eye Tracking Research and Applications(2023)
摘要
The power requirements of video-oculography systems can be prohibitive for high-speed operation on portable devices. Recently, low-power alternatives such as photosensors have been evaluated, providing gaze estimates at high frequency with a trade-off in accuracy and robustness. Potentially, an approach combining slow/high-fidelity and fast/low-fidelity sensors should be able to exploit their complementarity to track fast eye motion accurately and robustly. To foster research on this topic, we introduce OpenSFEDS, a near-eye gaze estimation dataset containing approximately 2M synthetic camera-photosensor image pairs sampled at 500 Hz under varied appearance and camera position. We also formulate the task of sensor fusion for gaze estimation, proposing a deep learning framework consisting in appearance-based encoding and temporal eye-state dynamics. We evaluate several single- and multi-rate fusion baselines on OpenSFEDS, achieving 8.7% error decrease when tracking fast eye movements with a multi-rate approach vs. a gaze forecasting approach operating with a low-speed sensor alone.
更多查看译文
关键词
Gaze estimation,Sensor fusion,Deep learning,Eye tracking,Virtual reality,Photosensors
AI 理解论文
溯源树
样例
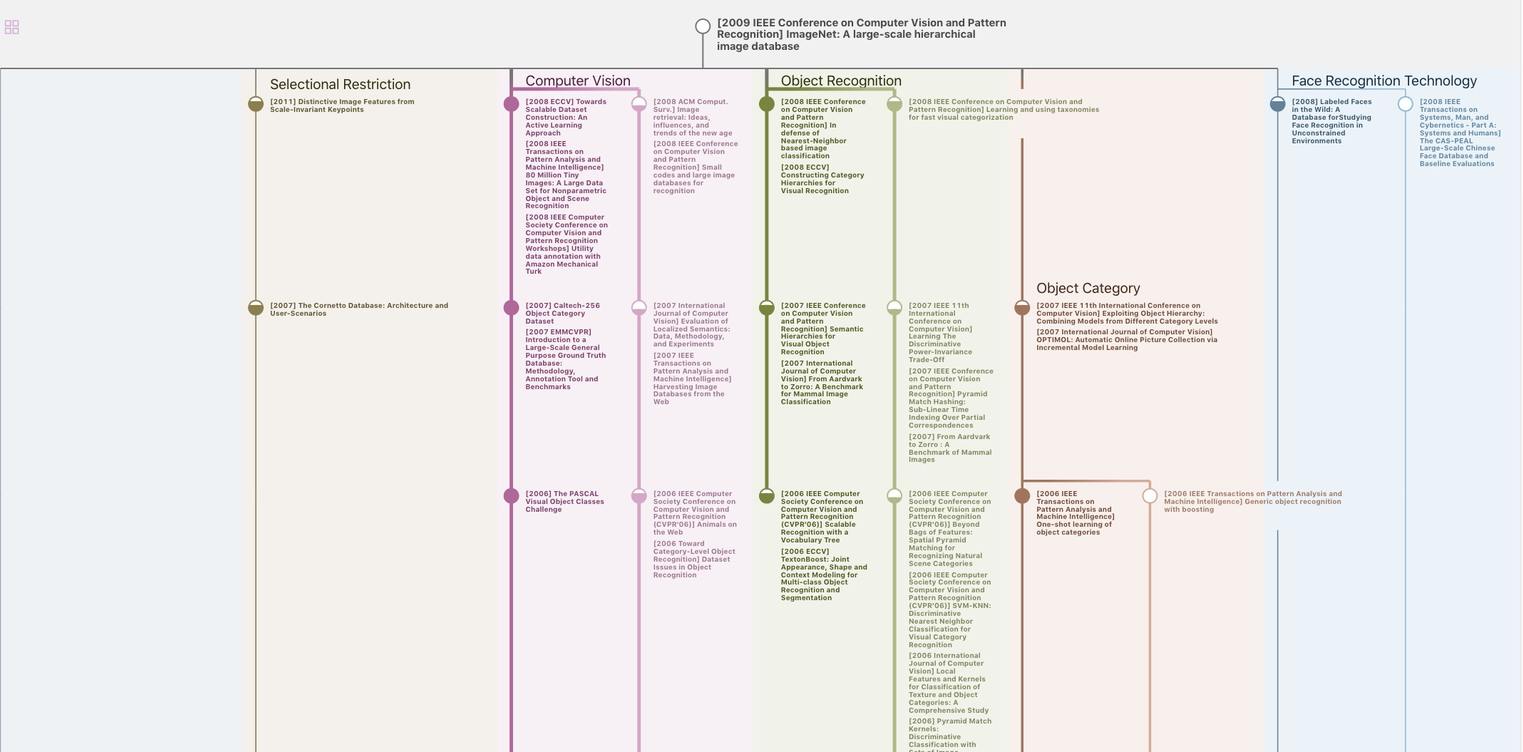
生成溯源树,研究论文发展脉络
Chat Paper
正在生成论文摘要