Differentially Private Low-dimensional Synthetic Data from High-dimensional Datasets
CoRR(2023)
摘要
Differentially private synthetic data provide a powerful mechanism to enable
data analysis while protecting sensitive information about individuals.
However, when the data lie in a high-dimensional space, the accuracy of the
synthetic data suffers from the curse of dimensionality. In this paper, we
propose a differentially private algorithm to generate low-dimensional
synthetic data efficiently from a high-dimensional dataset with a utility
guarantee with respect to the Wasserstein distance. A key step of our algorithm
is a private principal component analysis (PCA) procedure with a near-optimal
accuracy bound that circumvents the curse of dimensionality. Unlike the
standard perturbation analysis, our analysis of private PCA works without
assuming the spectral gap for the covariance matrix.
更多查看译文
关键词
data,low-dimensional,high-dimensional
AI 理解论文
溯源树
样例
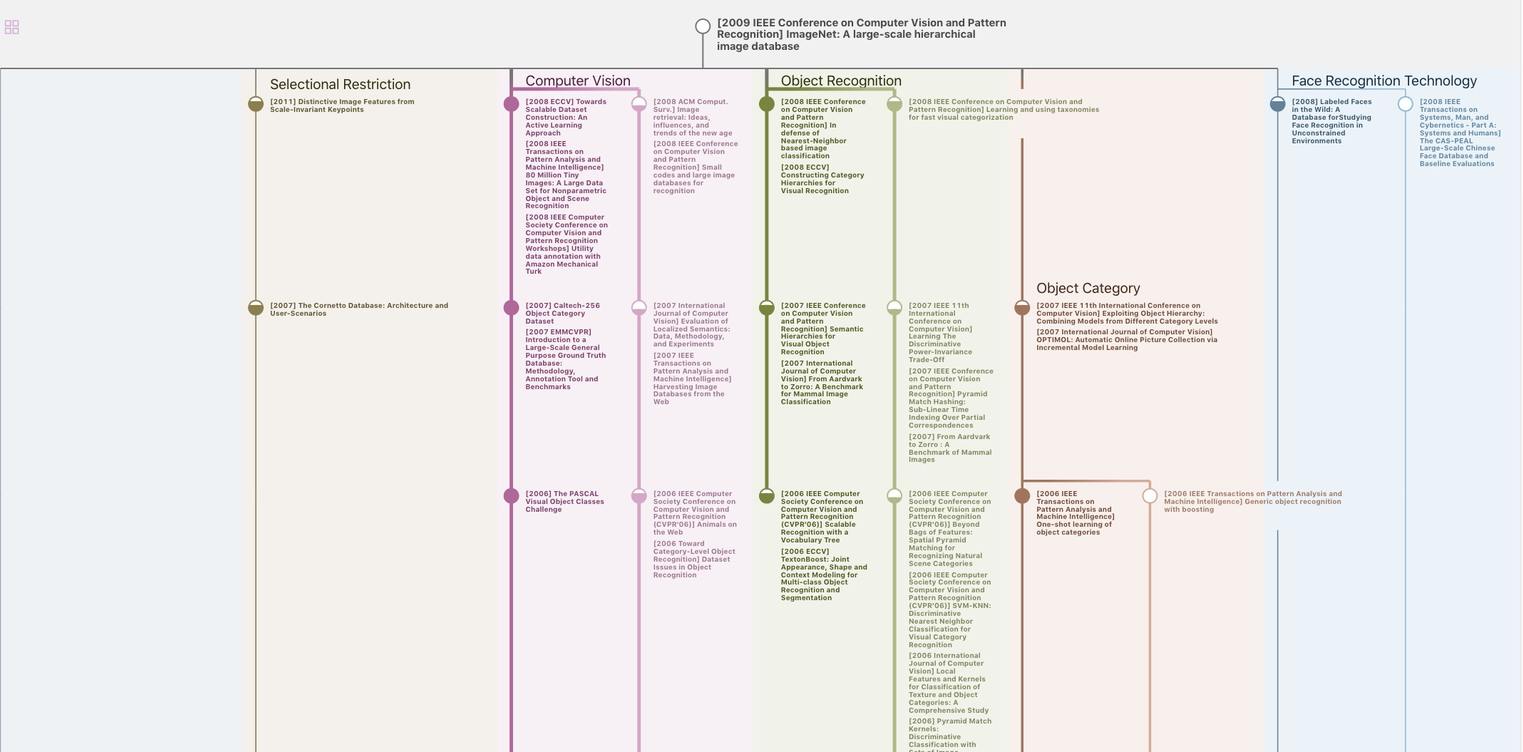
生成溯源树,研究论文发展脉络
Chat Paper
正在生成论文摘要