A Learning-based Nonlinear Model Predictive Controller for a Real Go-Kart based on Black-box Dynamics Modeling through Gaussian Processes
CoRR(2023)
摘要
Lately, Nonlinear Model Predictive Control (NMPC)has been successfully applied to (semi-) autonomous driving problems and has proven to be a very promising technique. However, accurate control models for real vehicles could require costly and time-demanding specific measurements. To address this problem, the exploitation of system data to complement or derive the prediction model of the NMPC has been explored, employing learning dynamics approaches within Learning-based NMPC (LbNMPC). Its application to the automotive field has focused on discrete grey-box modeling, in which a nominal dynamics model is enhanced by the data-driven component. In this manuscript, we present an LbNMPC controller for a real go-kart based on a continuous black-box model of the accelerations obtained by Gaussian Processes. We show the effectiveness of the proposed approach by testing the controller on a real go-kart vehicle, highlighting the approximation steps required to get an exploitable GP model on a real-time application.
更多查看译文
关键词
nonlinear model predictive controller,gaussian processes,dynamics,learning-based,go-kart,black-box
AI 理解论文
溯源树
样例
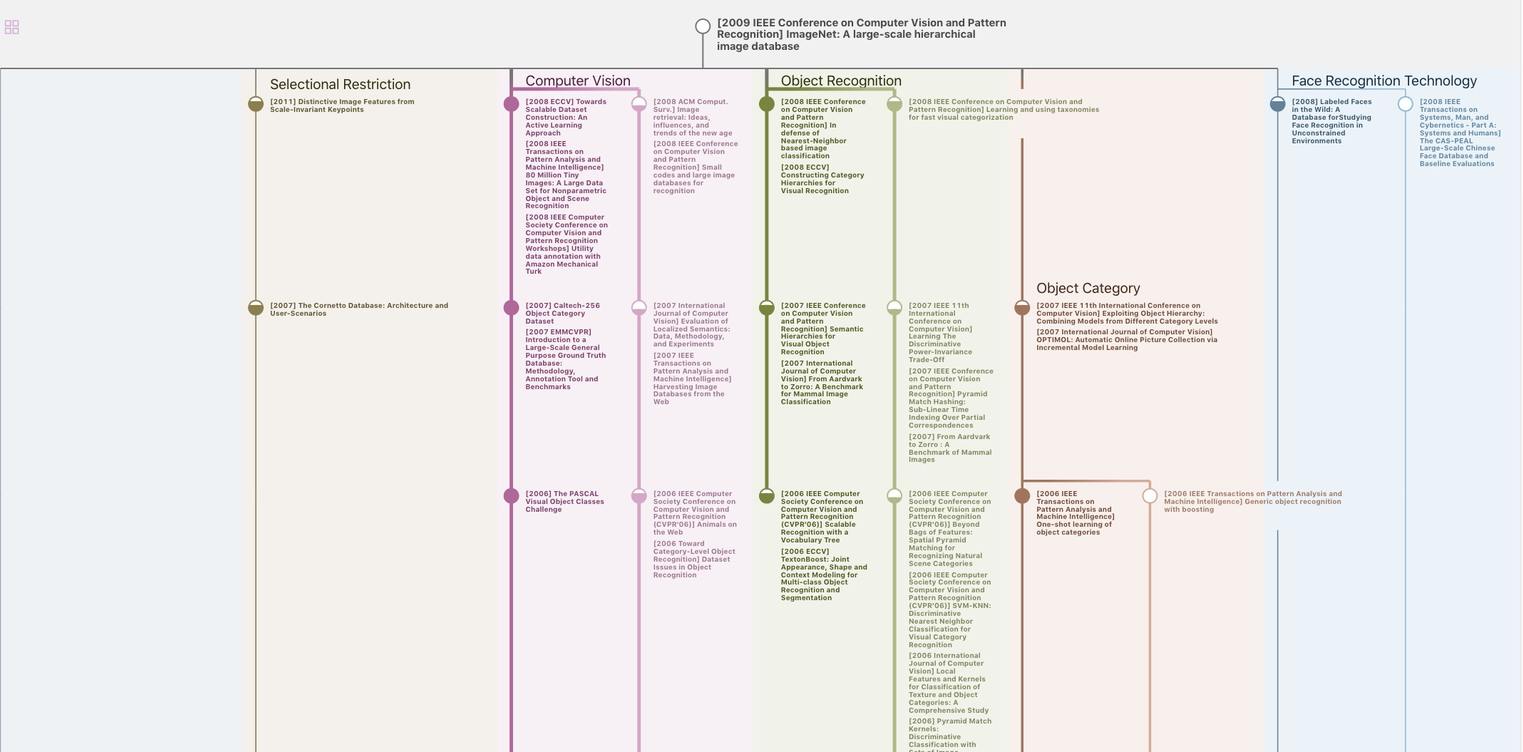
生成溯源树,研究论文发展脉络
Chat Paper
正在生成论文摘要