ContrastNER: Contrastive-based Prompt Tuning for Few-shot NER
CoRR(2023)
摘要
Prompt-based language models have produced encouraging results in numerous applications, including Named Entity Recognition (NER) tasks. NER aims to identify entities in a sentence and provide their types. However, the strong performance of most available NER approaches is heavily dependent on the design of discrete prompts and a verbalizer to map the model-predicted outputs to entity categories, which are complicated undertakings. To address these challenges, we present ContrastNER, a prompt-based NER framework that employs both discrete and continuous tokens in prompts and uses a contrastive learning approach to learn the continuous prompts and forecast entity types. The experimental results demonstrate that ContrastNER obtains competitive performance to the state-of-the-art NER methods in high-resource settings and outperforms the state-of-the-art models in low-resource circumstances without requiring extensive manual prompt engineering and verbalizer design.
更多查看译文
关键词
contrastner,prompt tuning,contrastive-based,few-shot
AI 理解论文
溯源树
样例
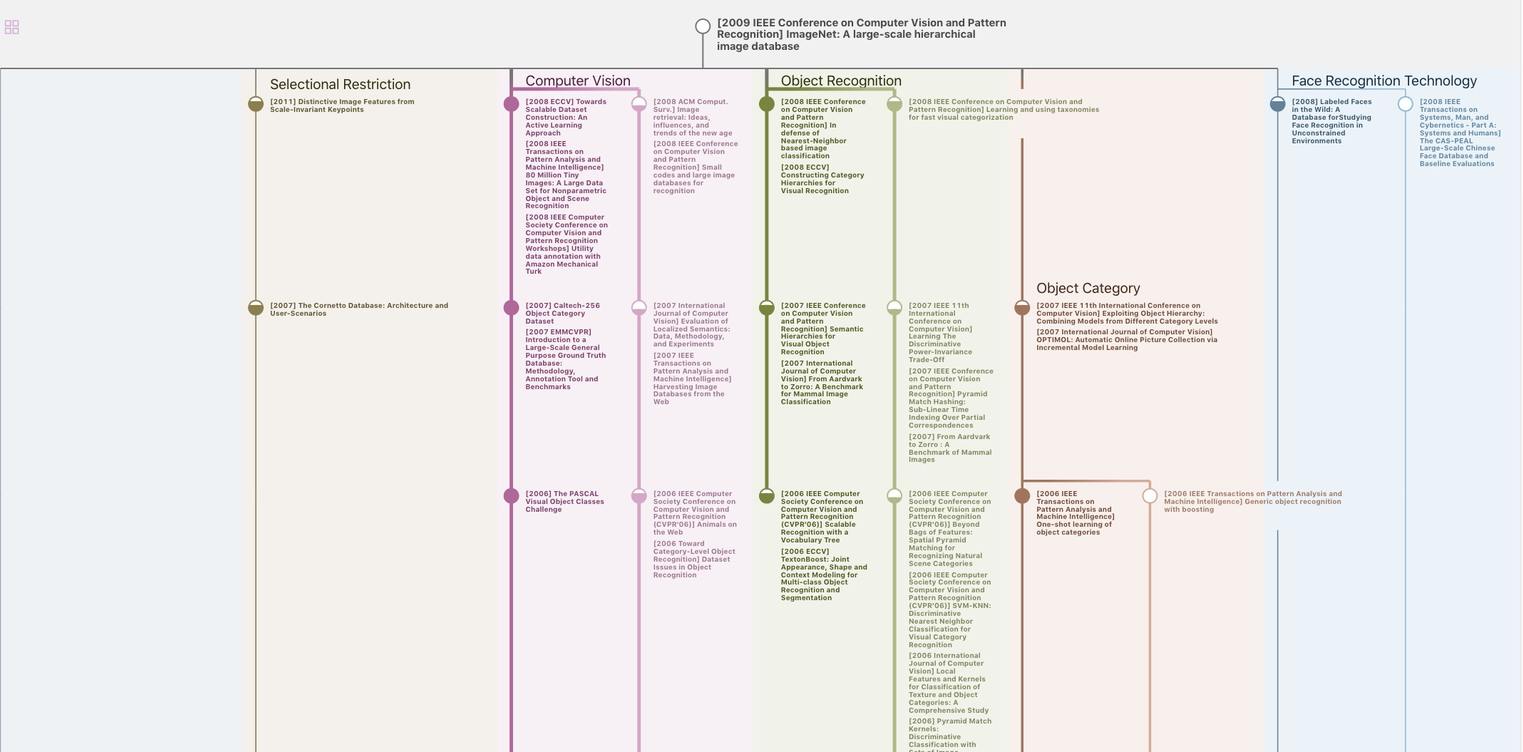
生成溯源树,研究论文发展脉络
Chat Paper
正在生成论文摘要