Learning Causal Graphs via Monotone Triangular Transport Maps
CoRR(2023)
摘要
We study the problem of causal structure learning from data using optimal transport (OT). Specifically, we first provide a constraint-based method which builds upon lower-triangular monotone parametric transport maps to design conditional independence tests which are agnostic to the noise distribution. We provide an algorithm for causal discovery up to Markov Equivalence with no assumptions on the structural equations/noise distributions, which allows for settings with latent variables. Our approach also extends to score-based causal discovery by providing a novel means for defining scores. This allows us to uniquely recover the causal graph under additional identifiability and structural assumptions, such as additive noise or post-nonlinear models. We provide experimental results to compare the proposed approach with the state of the art on both synthetic and real-world datasets.
更多查看译文
关键词
causal graphs,monotone triangular transport maps,learning
AI 理解论文
溯源树
样例
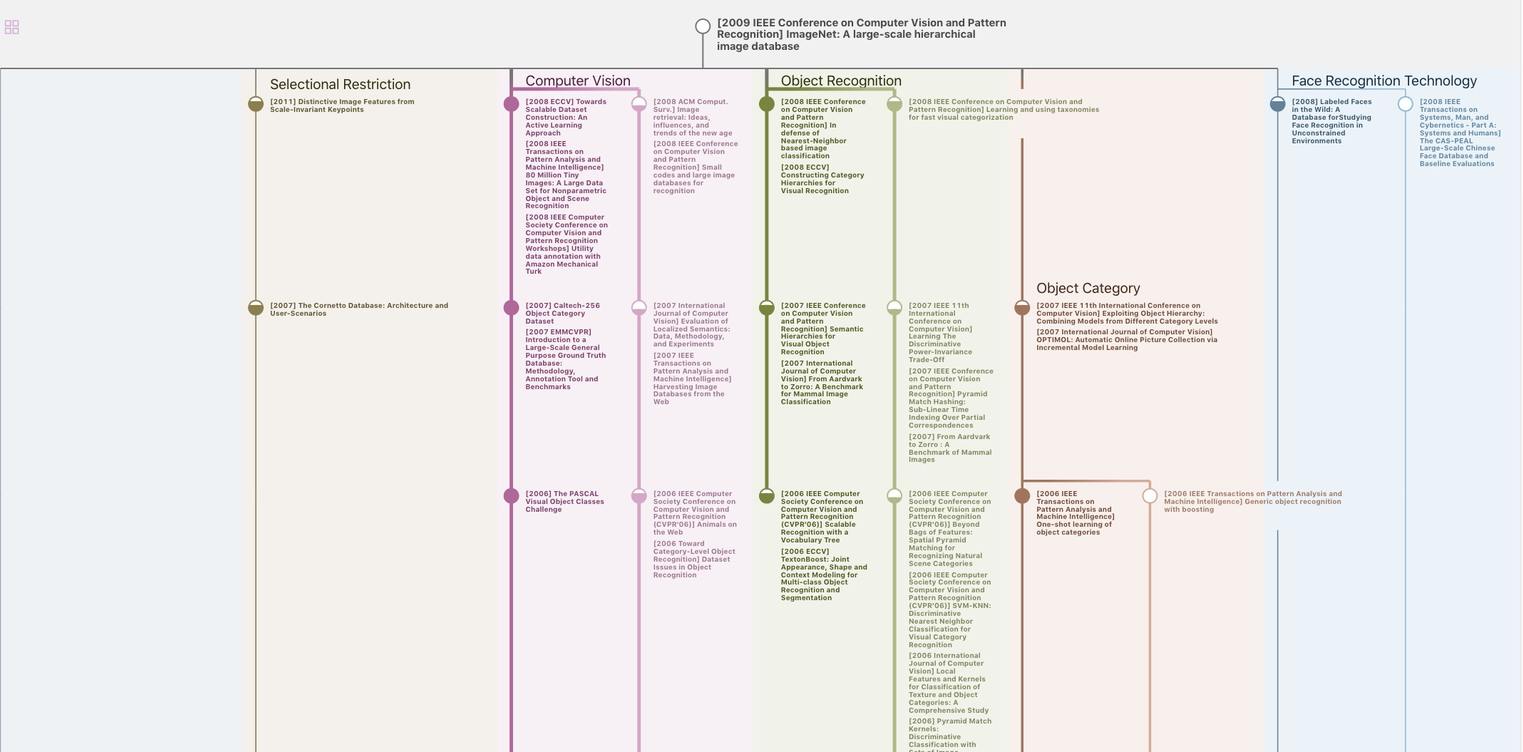
生成溯源树,研究论文发展脉络
Chat Paper
正在生成论文摘要