Robust Influence Maximization under Both Aleatory and Epistemic Uncertainty
ACM transactions on knowledge discovery from data(2023)
摘要
Uncertainty is ubiquitous in almost every real-life optimization problem, which must be effectively managed to get a robust outcome. This is also true for the Influence Maximization (IM) problem, which entails locating a set of influential users within a social network. However, most of the existing IM approaches have overlooked the uncertain factors in finding the optimal solution, which often leads to subpar performance in reality. A few recent studies have considered only the epistemic uncertainty (i.e., arises from the imprecise data), while ignoring completely the aleatory uncertainty (i.e., arises from natural or physical variability). In this article, we propose a formulation and a novel algorithm for the Robust Influence Maximization (RIM) problem under both types of uncertainties. First, we develop a robust influence spread function under aleatory uncertainty that, in contrast to the existing IM theory, is no longer monotone and submodular. Thereafter, we expand our RIM formulation to incorporate epistemic uncertainty aiming to maximize the robust ratio between the selected worst-case solution and the best-case optimal solution, adopting a conservative approach. Furthermore, using a chance-constraint-based method, we investigated feasibility robustness by accounting for the uncertainties related to constraint functions. Finally, an Evolutionary Algorithm (named EA-RIM) is designed to solve the proposed formulation of the RIM problem. Experimental evaluation results on four empirical datasets show that our proposed formulation and algorithm are more effective in dealing with uncertainties and finding an optimal solution for the RIM problem.
更多查看译文
关键词
Uncertainty modeling,Robust InfluenceMaximization,evolutionary computation,social network analysis
AI 理解论文
溯源树
样例
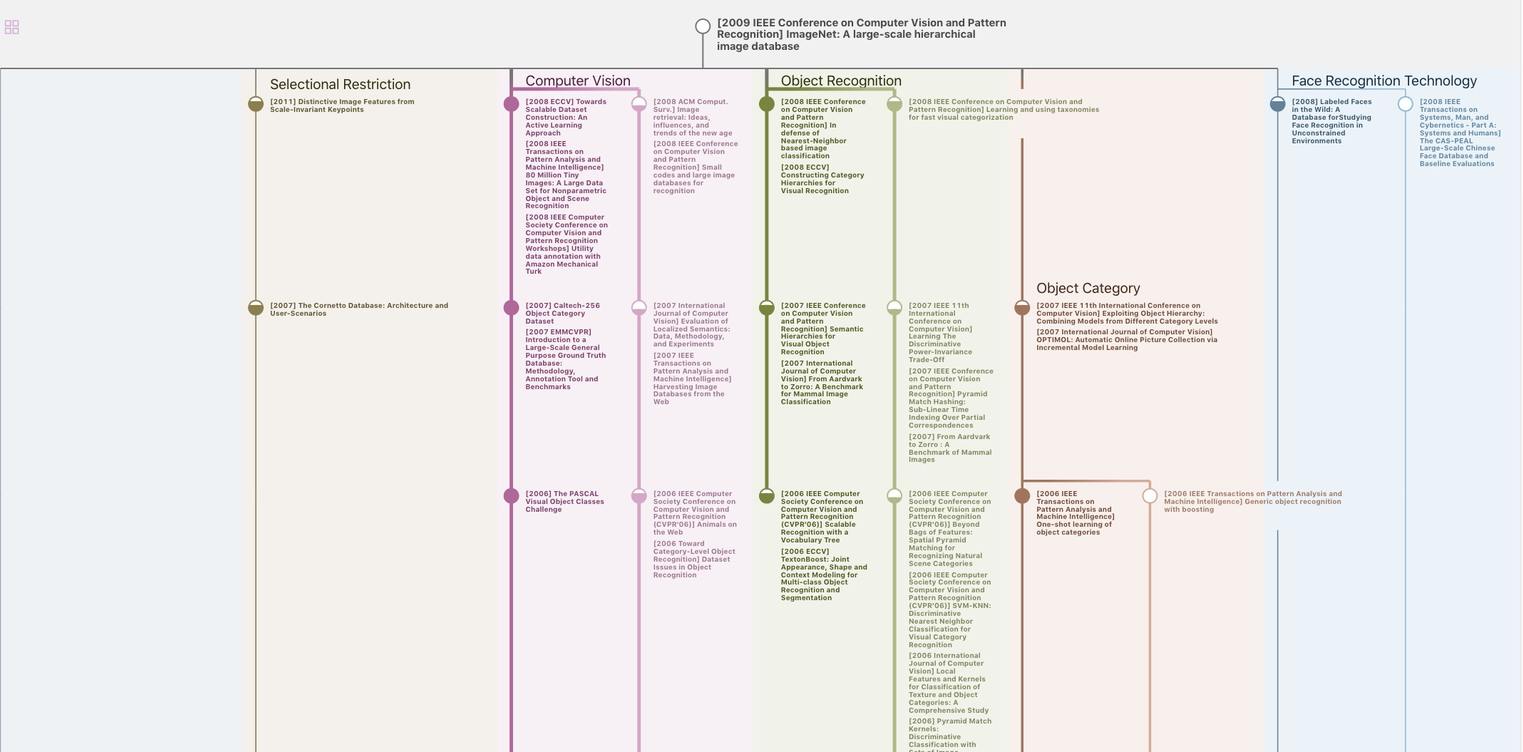
生成溯源树,研究论文发展脉络
Chat Paper
正在生成论文摘要