Optimal Scheduling of Electric Vehicle Charging with Deep Reinforcement Learning Considering End Users Flexibility
13th Mediterranean Conference on Power Generation, Transmission, Distribution and Energy Conversion (MEDPOWER 2022)(2022)
摘要
The rapid growth of decentralized energy resources and especially Electric Vehicles (EV), that are expected to increase sharply over the next decade, will put further stress on existing power distribution networks, increasing the need for higher system reliability and flexibility. In an attempt to avoid unnecessary network investments and to increase the controllability over distribution networks, network operators develop demand response (DR) programs that incentivize end users to shift their consumption in return for financial or other benefits. Artificial intelligence (AI) methods are in the research forefront for residential load scheduling applications, mainly due to their high accuracy, high computational speed and lower dependence on the physical characteristics of the models under development. The aim of this work is to identify households' EV cost-reducing charging policy under a Time-of-Use tariff scheme, with the use of Deep Reinforcement Learning, and more specifically Deep Q-Networks (DQN). A novel end users flexibility potential reward is inferred from historical data analysis, where households with solar power generation have been used to train and test the designed algorithm. The suggested DQN EV charging policy can lead to more than 20 users electricity bills.
更多查看译文
关键词
artificial intelligence methods,data analysis,deep Q-networks,deep reinforcement learning,demand response programs,electric vehicle charging,electricity bills,energy resources,power distribution networks,solar power generation,system reliability,time-of-use tariff scheme
AI 理解论文
溯源树
样例
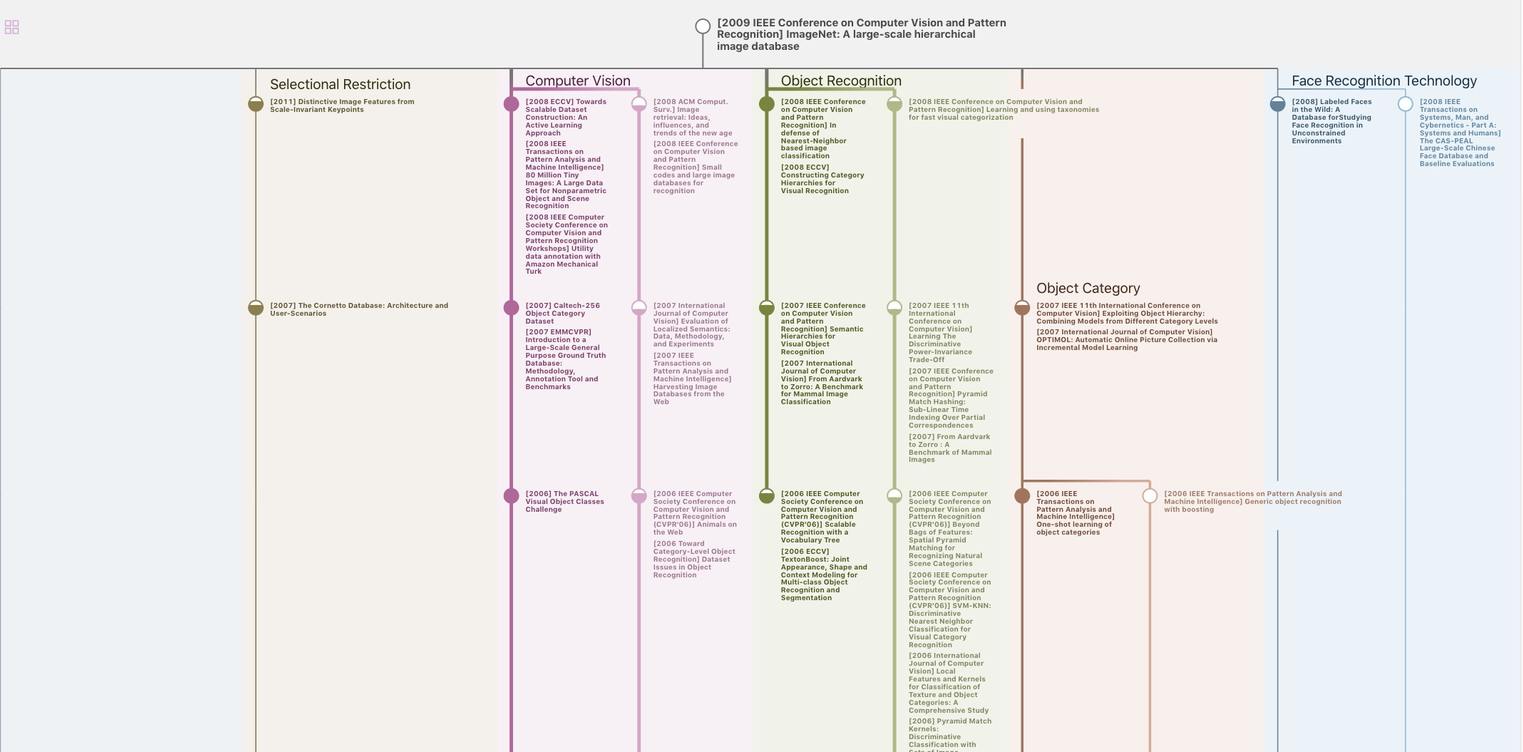
生成溯源树,研究论文发展脉络
Chat Paper
正在生成论文摘要