Improving Model Learning by Inferring Separating Sequences from Traces
2023 IEEE International Conference on Software Testing, Verification and Validation Workshops (ICSTW)(2023)
摘要
Models that can represent the behavior of systems, such as a Finite State Machine (FSM), are crucial for software development and maintenance as they serve as a base for several automated activities like testing, verification, validation, and refinement of systems. Contrasting their importance and value, models are usually complex and costly to obtain. Model inference algorithms can help with this task. In this paper, we propose a method to improve the learning process of FSMs by inferring separating sequences from traces and using them in characterization sets. We conducted a case study to assess the impact of the proposed method on an FSM learning algorithm called hW-inference. We observed that the proposed method was capable of improving by 24% the learning process.
更多查看译文
关键词
model learning,FSM,characterization sets,and separating sequences
AI 理解论文
溯源树
样例
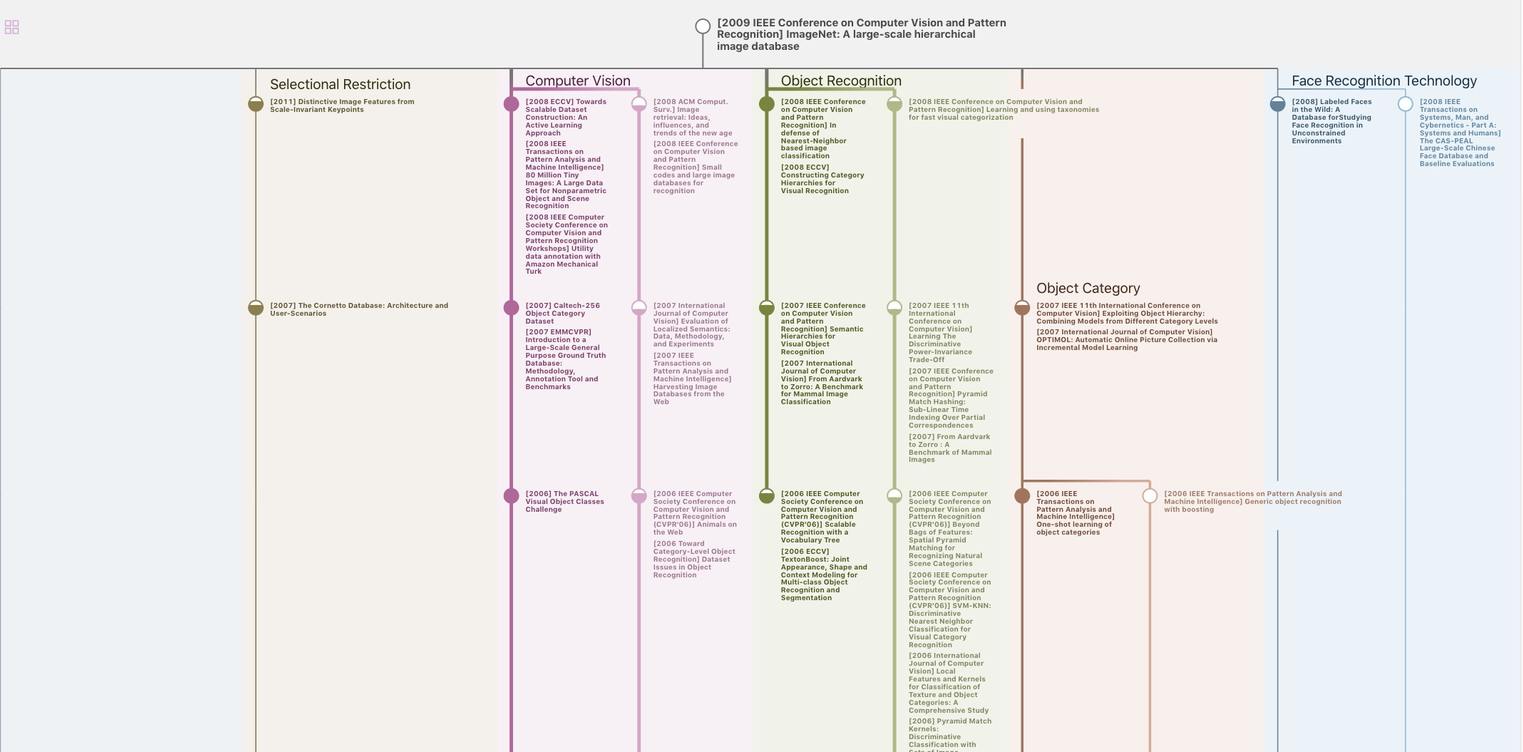
生成溯源树,研究论文发展脉络
Chat Paper
正在生成论文摘要