#REVAL: a semantic evaluation framework for hashtag recommendation
CoRR(2023)
摘要
Automatic evaluation of hashtag recommendation models is a fundamental task in many online social network systems. In the traditional evaluation method, the recommended hashtags from an algorithm are firstly compared with the ground truth hashtags for exact correspondences. The number of exact matches is then used to calculate the hit rate, hit ratio, precision, recall, or F1-score. This way of evaluating hashtag similarities is inadequate as it ignores the semantic correlation between the recommended and ground truth hashtags. To tackle this problem, we propose a novel semantic evaluation framework for hashtag recommendation, called #REval. This framework includes an internal module referred to as BERTag, which automatically learns the hashtag embeddings. We investigate on how the #REval framework performs under different word embedding methods and different numbers of synonyms and hashtags in the recommendation using our proposed #REval-hit-ratio measure. Our experiments of the proposed framework on three large datasets show that #REval gave more meaningful hashtag synonyms for hashtag recommendation evaluation. Our analysis also highlights the sensitivity of the framework to the word embedding technique, with #REval based on BERTag more superior over #REval based on FastText and Word2Vec.
更多查看译文
关键词
#reval,semantic evaluation framework,recommendation
AI 理解论文
溯源树
样例
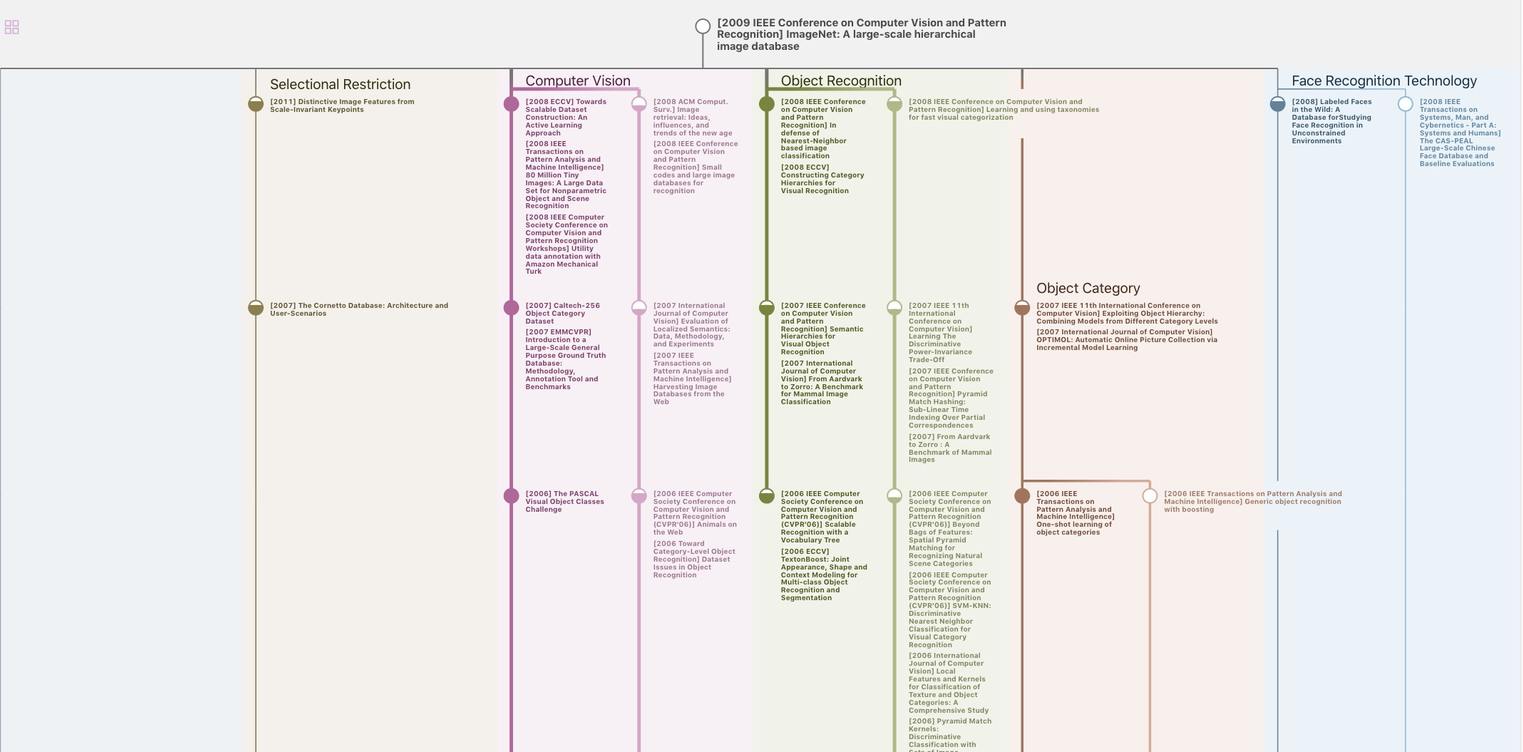
生成溯源树,研究论文发展脉络
Chat Paper
正在生成论文摘要