Semantic Segmentation with Bidirectional Language Models Improves Long-form ASR
CoRR(2023)
摘要
We propose a method of segmenting long-form speech by separating semantically complete sentences within the utterance. This prevents the ASR decoder from needlessly processing faraway context while also preventing it from missing relevant context within the current sentence. Semantically complete sentence boundaries are typically demarcated by punctuation in written text; but unfortunately, spoken real-world utterances rarely contain punctuation. We address this limitation by distilling punctuation knowledge from a bidirectional teacher language model (LM) trained on written, punctuated text. We compare our segmenter, which is distilled from the LM teacher, against a segmenter distilled from a acoustic-pause-based teacher used in other works, on a streaming ASR pipeline. The pipeline with our segmenter achieves a 3.2% relative WER gain along with a 60 ms median end-of-segment latency reduction on a YouTube captioning task.
更多查看译文
关键词
bidirectional language models,asr,segmentation,long-form
AI 理解论文
溯源树
样例
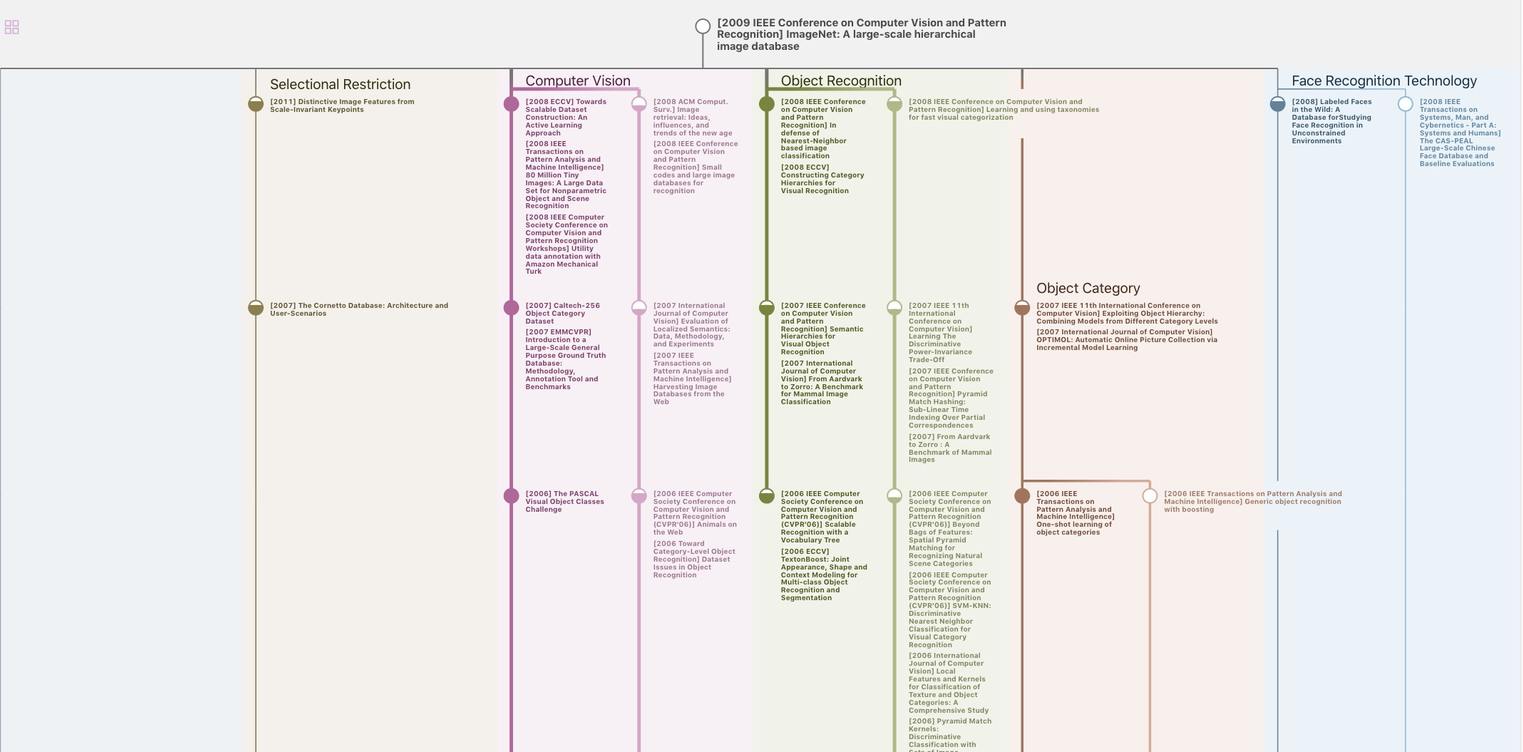
生成溯源树,研究论文发展脉络
Chat Paper
正在生成论文摘要