BRIGHT: Bi-level Feature Representation of Image Collections using Groups of Hash Tables
CoRR(2023)
摘要
We present BRIGHT, a bi-levelfeature representation for an imagecollection, consisting of a per-image latent space on top of a multi-scale feature grid space. Our representation is learned by an autoencoder to encode images intocontinuouskey codes, which are used to retrieve features fromgroups of multi-resolution hashtables. Our key codes and hash tables are trained together continuously with well-defined gradient flows, leading to high usage of the hash table entries and improved generative modeling compared to discrete Vector Quantization (VQ). Differently from existing continuous representations such as KL-regularized latent codes, our key codes are strictly bounded in scale and variance. Overall, feature encoding by BRIGHT is compact, efficient to train, and enables generative modeling over the image codes using state-of-the-art generators such as latent diffusion models(LDMs). Experimental results show that our method achieves comparable recon-struction results to VQ methods while having a smaller and more efficient decoder network. By applying LDM over our key code space, we achieve state-of-the-art performance on image synthesis on the LSUN-Church and human-face datasets.
更多查看译文
关键词
image collections,feature,representation,bi-level
AI 理解论文
溯源树
样例
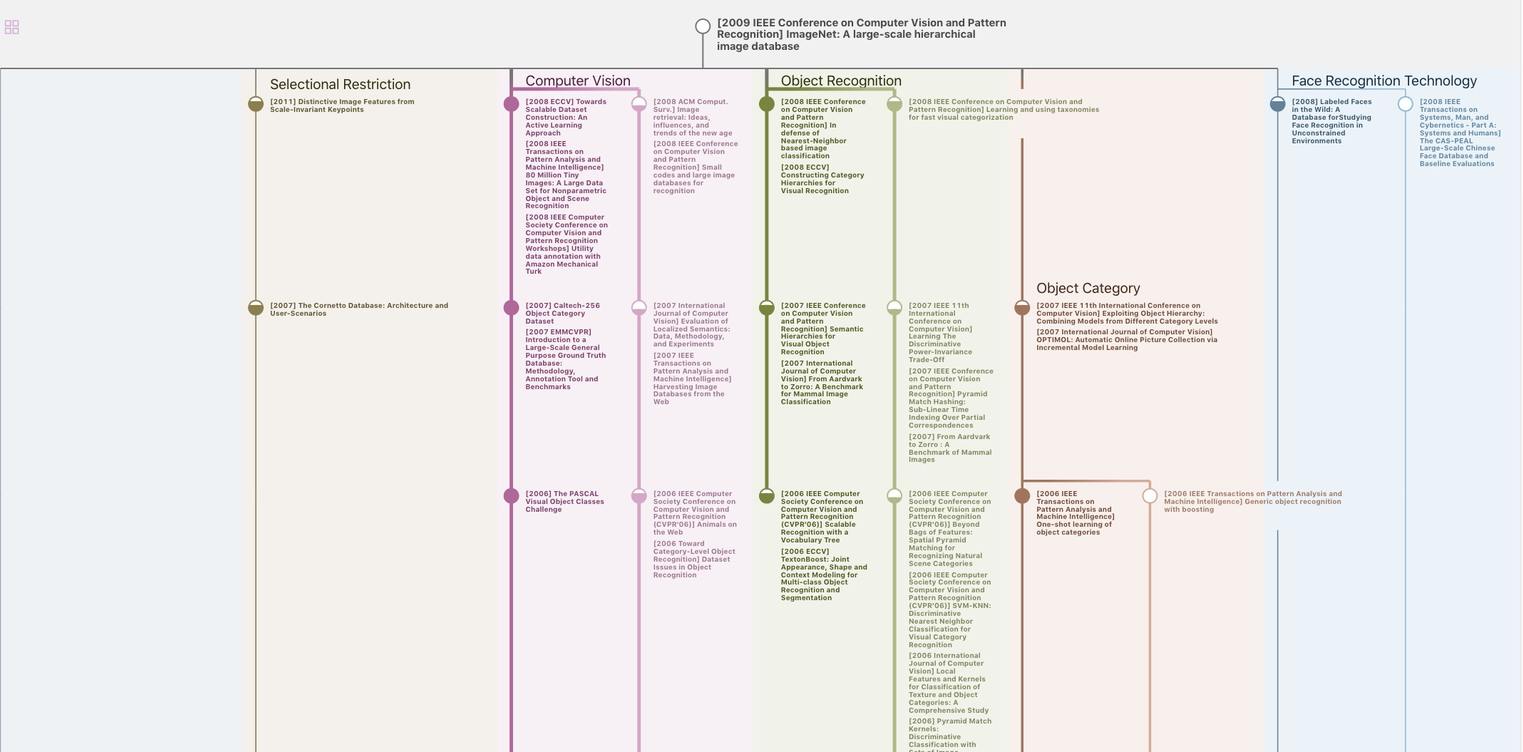
生成溯源树,研究论文发展脉络
Chat Paper
正在生成论文摘要