Collaborative Multi-Agent Heterogeneous Multi-Armed Bandits
CoRR(2023)
摘要
The study of collaborative multi-agent bandits has attracted significant attention recently. In light of this, we initiate the study of a new collaborative setting, consisting of $N$ agents such that each agent is learning one of $M$ stochastic multi-armed bandits to minimize their group cumulative regret. We develop decentralized algorithms which facilitate collaboration between the agents under two scenarios. We characterize the performance of these algorithms by deriving the per agent cumulative regret and group regret upper bounds. We also prove lower bounds for the group regret in this setting, which demonstrates the near-optimal behavior of the proposed algorithms.
更多查看译文
关键词
multi-agent,multi-armed
AI 理解论文
溯源树
样例
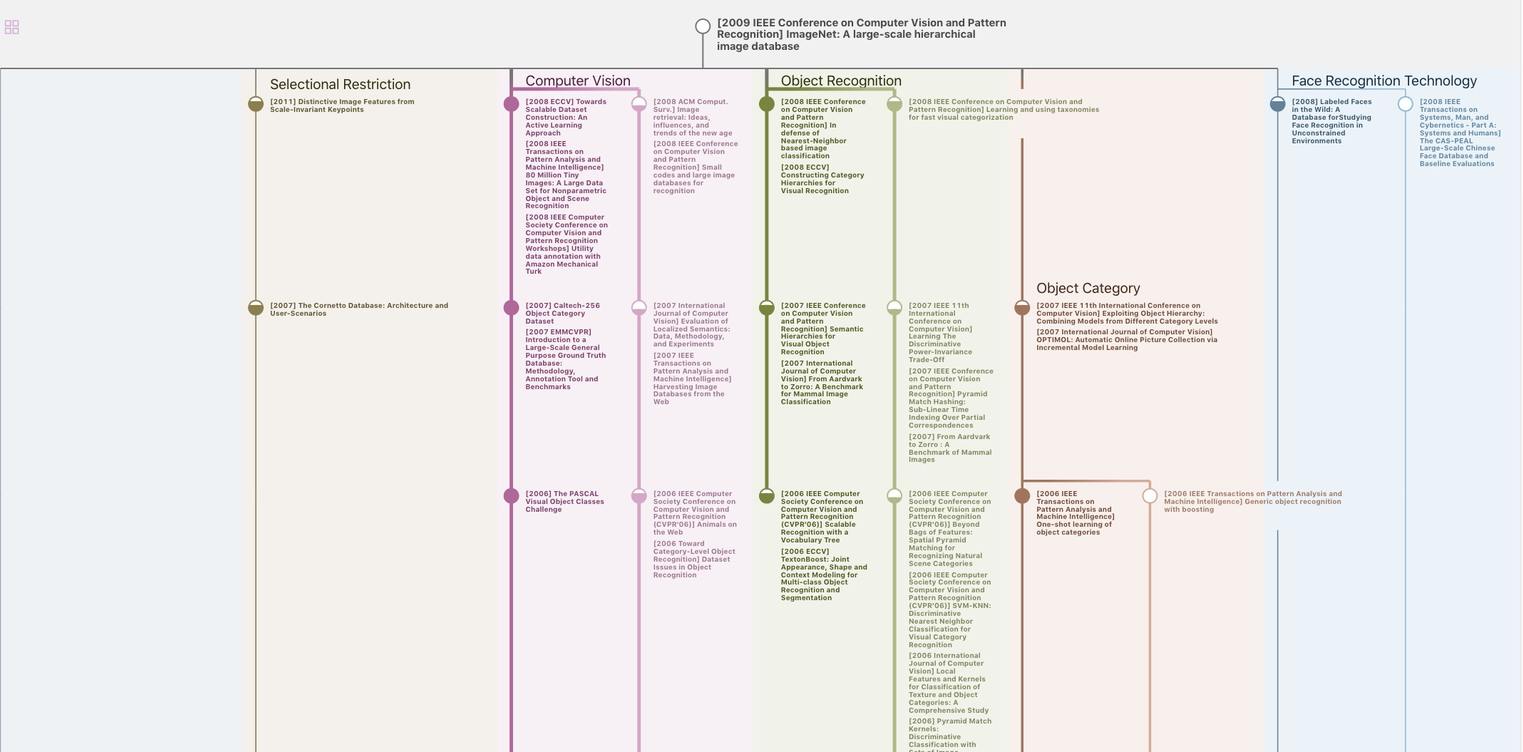
生成溯源树,研究论文发展脉络
Chat Paper
正在生成论文摘要