Empirical Sufficiency Lower Bounds for Language Modeling with Locally-Bootstrapped Semantic Structures
CoRR(2023)
摘要
In this work we build upon negative results from an attempt at language modeling with predicted semantic structure, in order to establish empirical lower bounds on what could have made the attempt successful. More specifically, we design a concise binary vector representation of semantic structure at the lexical level and evaluate in-depth how good an incremental tagger needs to be in order to achieve better-than-baseline performance with an end-to-end semantic-bootstrapping language model. We envision such a system as consisting of a (pretrained) sequential-neural component and a hierarchical-symbolic component working together to generate text with low surprisal and high linguistic interpretability. We find that (a) dimensionality of the semantic vector representation can be dramatically reduced without losing its main advantages and (b) lower bounds on prediction quality cannot be established via a single score alone, but need to take the distributions of signal and noise into account.
更多查看译文
关键词
language modeling,empirical sufficiency lower bounds,locally-bootstrapped
AI 理解论文
溯源树
样例
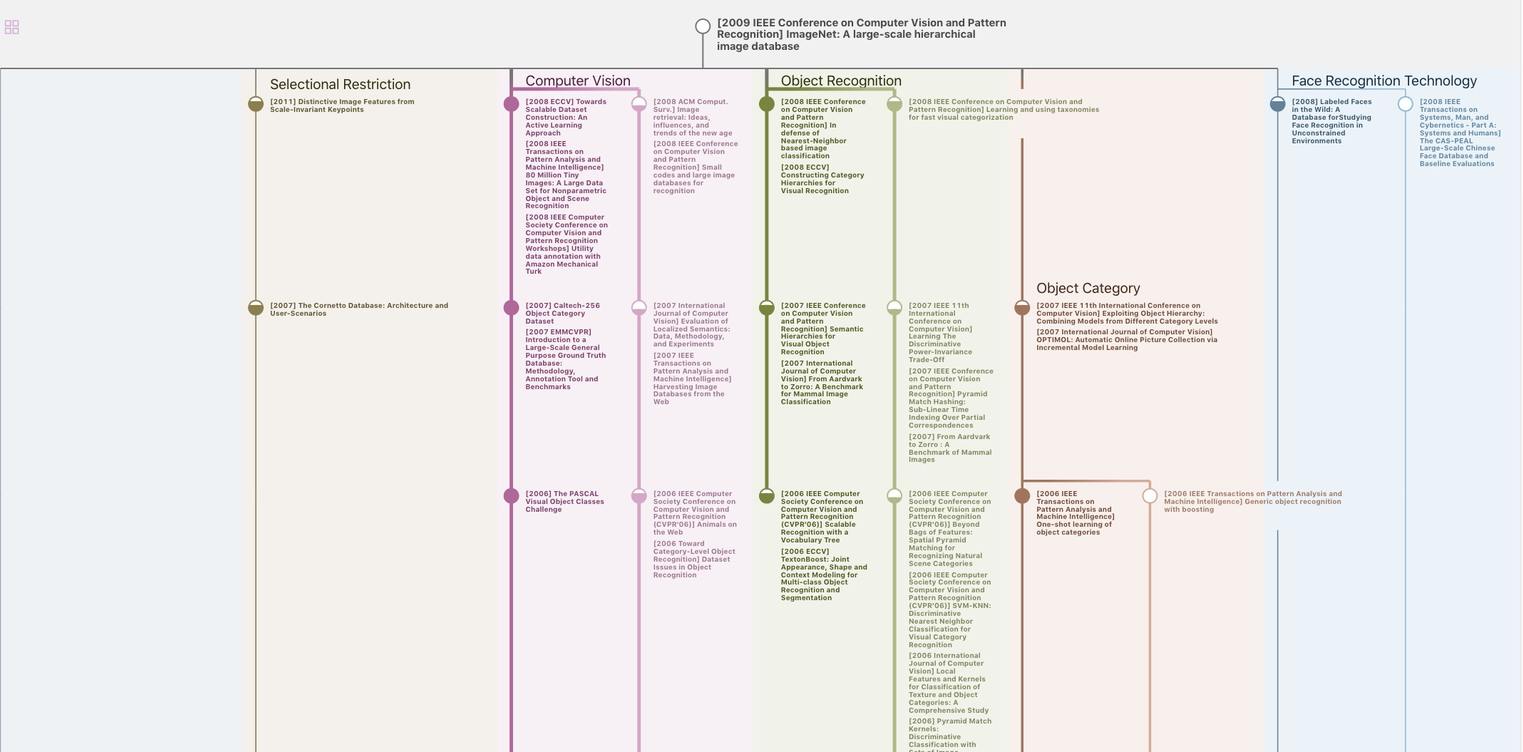
生成溯源树,研究论文发展脉络
Chat Paper
正在生成论文摘要