Reduced Precision Floating-Point Optimization for Deep Neural Network On-Device Learning on Microcontrollers
FUTURE GENERATION COMPUTER SYSTEMS-THE INTERNATIONAL JOURNAL OF ESCIENCE(2023)
摘要
Enabling On-Device Learning (ODL) for Ultra-Low-Power Micro-Controller Units (MCUs) is a key step for post-deployment adaptation and fine-tuning of Deep Neural Network (DNN) models in future TinyML applications. This paper tackles this challenge by introducing a novel reduced precision optimization technique for ODL primitives on MCU-class devices, leveraging the State-of-Art advancements in RISC-V RV32 architectures with support for vectorized 16-bit floating-point (FP16) Single-Instruction Multiple-Data (SIMD) operations. Our approach for the Forward and Backward steps of the Back-Propagation training algorithm is composed of specialized shape transform operators and Matrix Multiplication (MM) kernels, accelerated with parallelization and loop unrolling. When evaluated on a single training step of a 2D Convolution layer, the SIMD-optimized FP16 primitives result up to 1.72× faster than the FP32 baseline on a RISC-V-based 8+1-core MCU. An average computing efficiency of 3.11 Multiply and Accumulate operations per clock cycle (MAC/clk) and 0.81 MAC/clk is measured for the end-to-end training tasks of a ResNet8 and a DS-CNN for Image Classification and Keyword Spotting, respectively – requiring 17.1 ms and 6.4 ms on the target platform to compute a training step on a single sample. Overall, our approach results more than two orders of magnitude faster than existing ODL software frameworks for single-core MCUs and outperforms by 1.6× previous FP32 parallel implementations on a Continual Learning setup.
更多查看译文
关键词
Parallel computing,Computer architecture,Open source software,Open architecture platforms,Deep learning
AI 理解论文
溯源树
样例
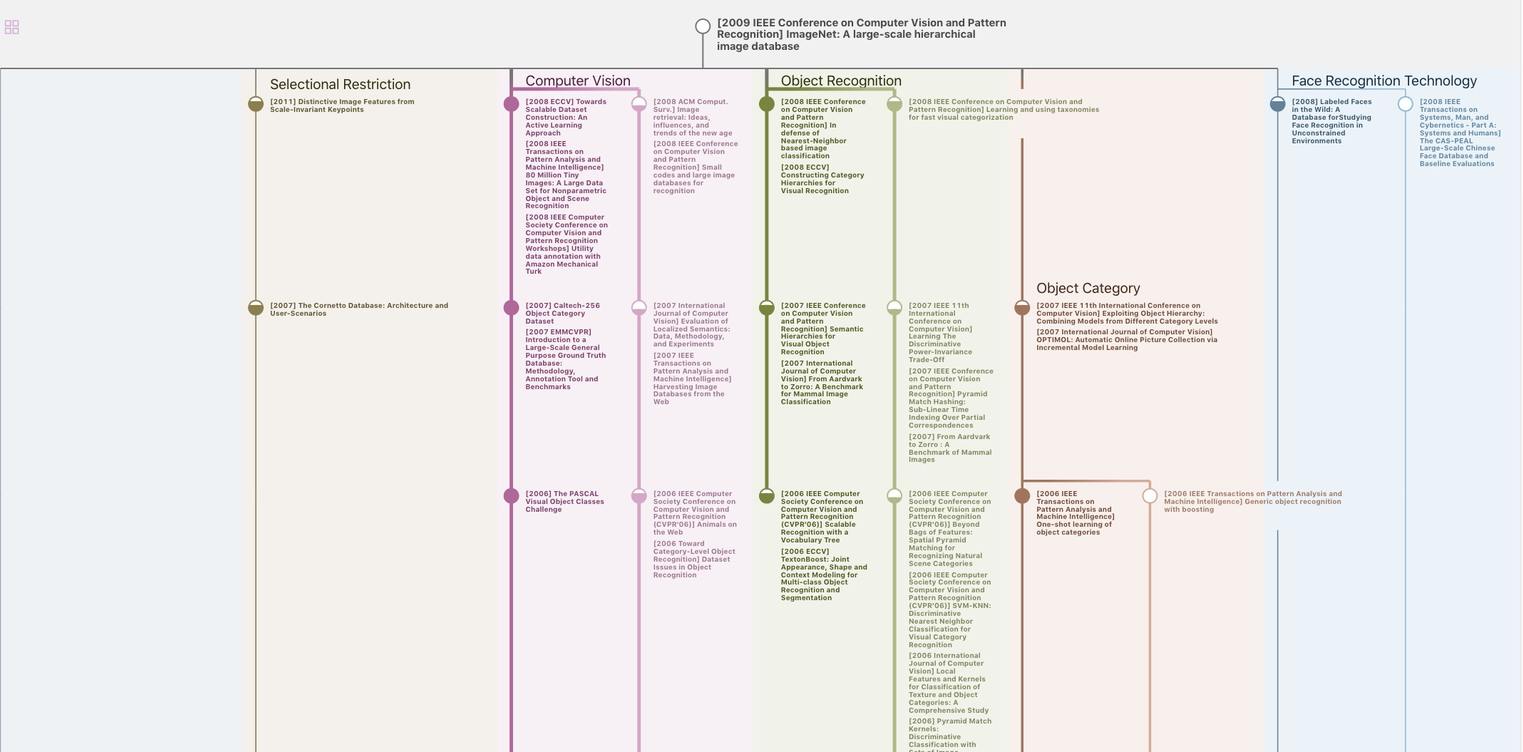
生成溯源树,研究论文发展脉络
Chat Paper
正在生成论文摘要