Robust Extraction of Thermal Observables from State Sampling and Real-Time Dynamics on Quantum Computers
QUANTUM(2023)
摘要
Simulating properties of quantum materials is one of the most promising applications of quantum computation, both near-and long-term. While real-time dynamics can be straightforwardly implemented, the finite temperature ensemble involves non-unitary operators that render an implementation on a near-term quantum computer extremely challenging. Recently, Lu, Banuls and Cirac, PRX Quantum 2, 020321 (2021) suggested a "timeseries quantum Monte Carlo method" which circumvents this problem by calculating finite temperature properties from Monte Carlo sampling of easily preparable states, where the Boltzmann weights are extracted from real -time quantum simulations via Wick's rotation. In this paper, we address the challenges associated with the practical applications of this method, using the two-dimensional transverse field Ising model as a testbed. We demonstrate that estimating Boltzmann weights via Wick's rotation is very sensitive to time-domain truncation and statistical shot noise. To alleviate this problem, we introduce a technique that imposes constraints on the density of states, most notably its non-negativity, and show that this way, we can reliably extract Boltzmann weights from noisy time series. In addition, we show how to reduce the statistical errors of Monte Carlo sampling via a reweighted version of the Wolff cluster algorithm. Our work enables the implementation of the time-series algorithm on present-day quantum computers to study finite temperature properties of many-body quantum systems.
更多查看译文
关键词
thermal observables,state sampling,quantum,robust extraction,real-time
AI 理解论文
溯源树
样例
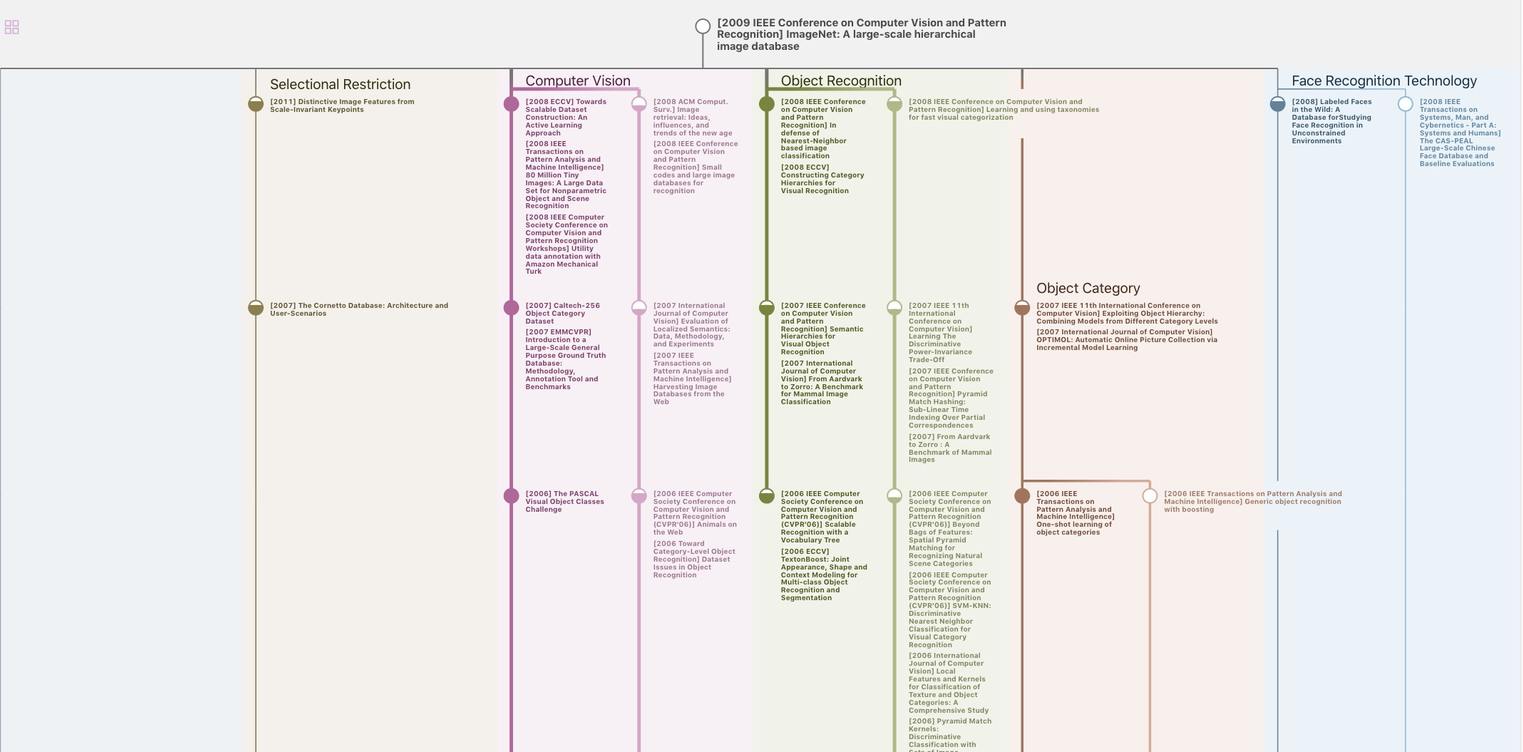
生成溯源树,研究论文发展脉络
Chat Paper
正在生成论文摘要