Offline Meta Reinforcement Learning with In-Distribution Online Adaptation
CoRR(2023)
摘要
Recent offline meta-reinforcement learning (meta-RL) methods typically utilize task-dependent behavior policies (e.g., training RL agents on each individual task) to collect a multi-task dataset. However, these methods always require extra information for fast adaptation, such as offline context for testing tasks. To address this problem, we first formally characterize a unique challenge in offline meta-RL: transition-reward distribution shift between offline datasets and online adaptation. Our theory finds that out-of-distribution adaptation episodes may lead to unreliable policy evaluation and that online adaptation with in-distribution episodes can ensure adaptation performance guarantee. Based on these theoretical insights, we propose a novel adaptation framework, called In-Distribution online Adaptation with uncertainty Quantification (IDAQ), which generates in-distribution context using a given uncertainty quantification and performs effective task belief inference to address new tasks. We find a return-based uncertainty quantification for IDAQ that performs effectively. Experiments show that IDAQ achieves state-of-the-art performance on the Meta-World ML1 benchmark compared to baselines with/without offline adaptation.
更多查看译文
关键词
offline,adaptation,online
AI 理解论文
溯源树
样例
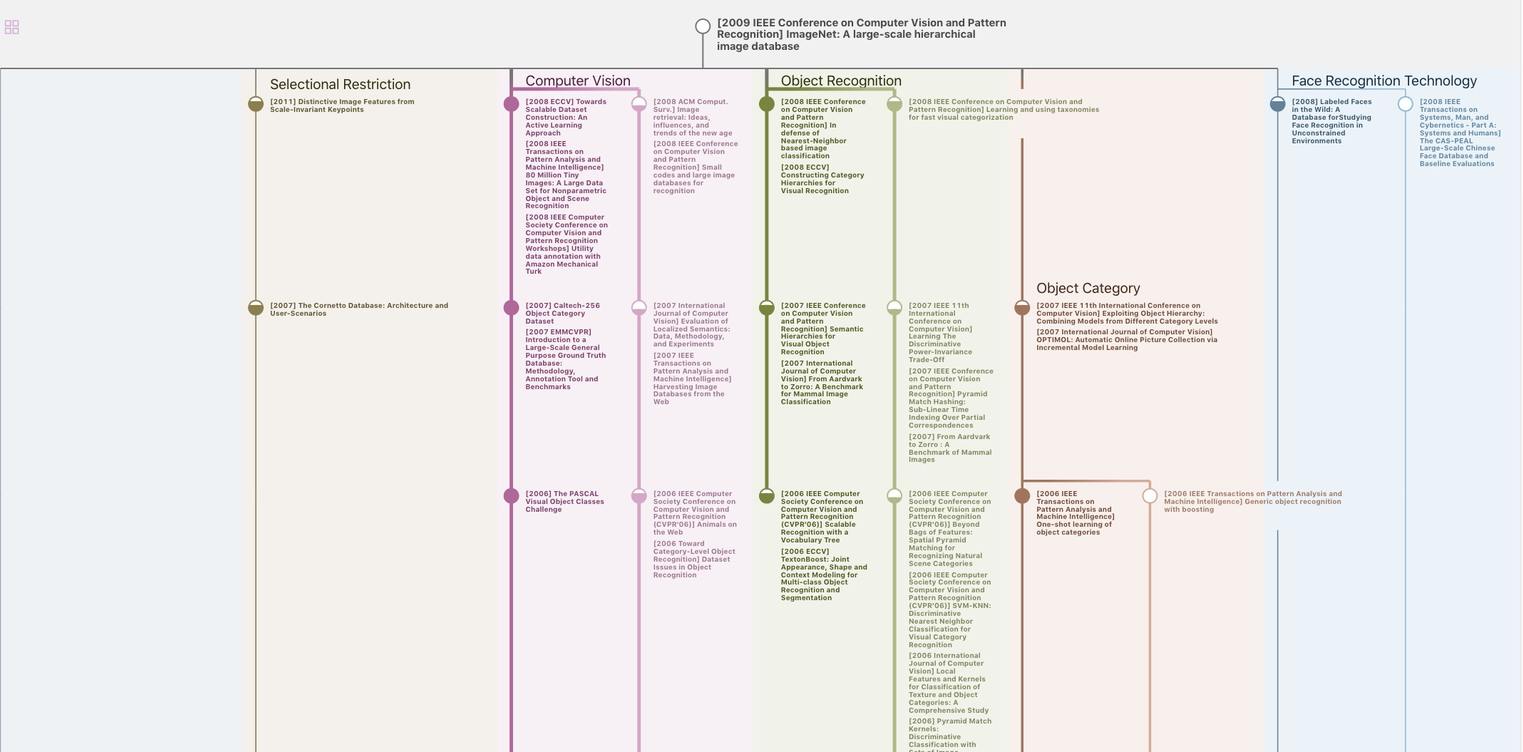
生成溯源树,研究论文发展脉络
Chat Paper
正在生成论文摘要