Causal discovery for time series with constraint-based model and PMIME measure
CoRR(2023)
摘要
Causality defines the relationship between cause and effect. In multivariate time series field, this notion allows to characterize the links between several time series considering temporal lags. These phenomena are particularly important in medicine to analyze the effect of a drug for example, in manufacturing to detect the causes of an anomaly in a complex system or in social sciences... Most of the time, studying these complex systems is made through correlation only. But correlation can lead to spurious relationships. To circumvent this problem, we present in this paper a novel approach for discovering causality in time series data that combines a causal discovery algorithm with an information theoretic-based measure. Hence the proposed method allows inferring both linear and non-linear relationships and building the underlying causal graph. We evaluate the performance of our approach on several simulated data sets, showing promising results.
更多查看译文
关键词
causal discovery,time series,constraint-based
AI 理解论文
溯源树
样例
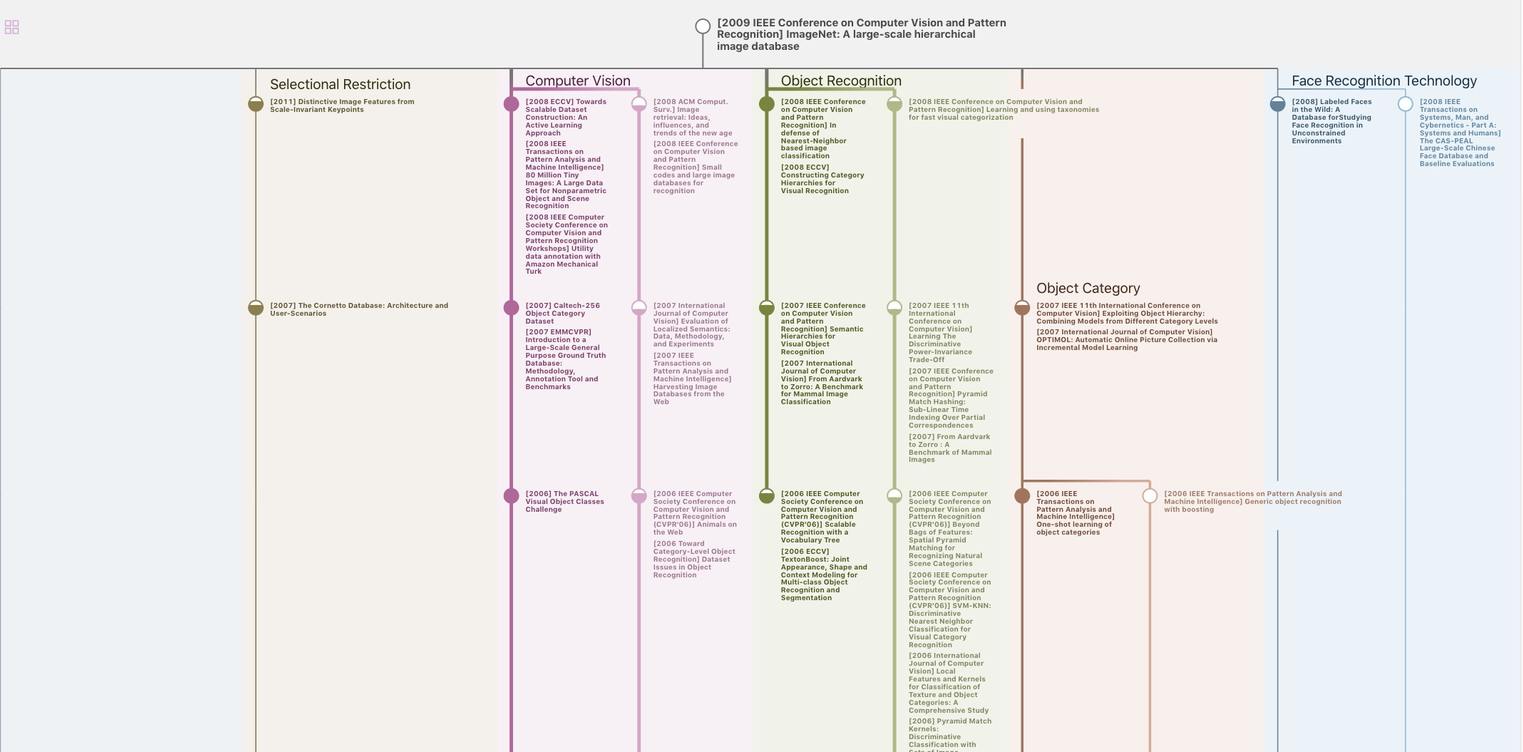
生成溯源树,研究论文发展脉络
Chat Paper
正在生成论文摘要