RINGER: Rapid Conformer Generation for Macrocycles with Sequence-Conditioned Internal Coordinate Diffusion
CoRR(2023)
摘要
Macrocyclic peptides are an emerging therapeutic modality, yet computational approaches for accurately sampling their diverse 3D ensembles remain challenging due to their conformational diversity and geometric constraints. Here, we introduce RINGER, a diffusion-based transformer model for sequence-conditioned generation of macrocycle structures based on internal coordinates. RINGER provides fast backbone sampling while respecting key structural invariances of cyclic peptides. Through extensive benchmarking and analysis against gold-standard conformer ensembles of cyclic peptides generated with metadynamics, we demonstrate how RINGER generates both high-quality and diverse geometries at a fraction of the computational cost. Our work lays the foundation for improved sampling of cyclic geometries and the development of geometric learning methods for peptides.
更多查看译文
关键词
rapid conformer generation,macrocycles,diffusion,sequence-conditioned
AI 理解论文
溯源树
样例
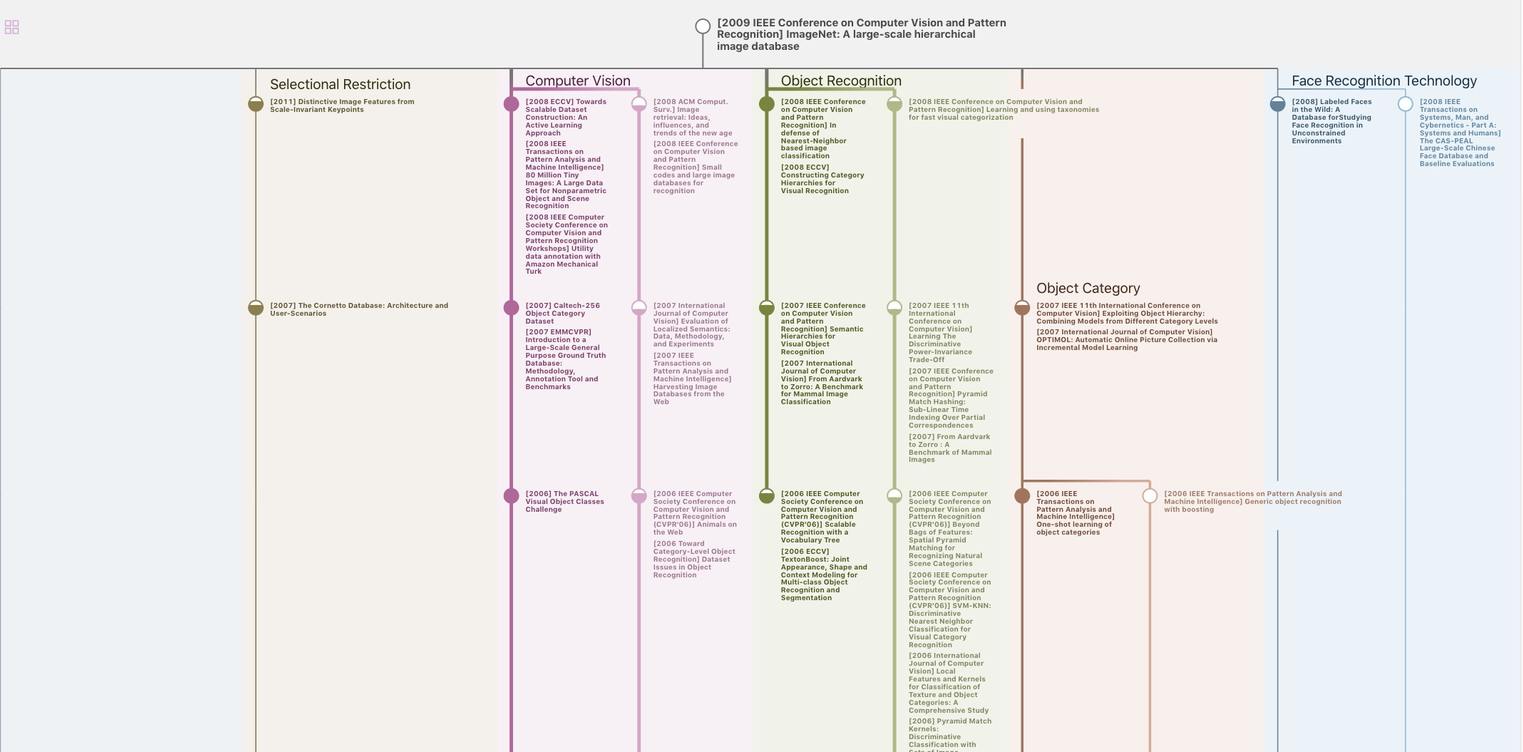
生成溯源树,研究论文发展脉络
Chat Paper
正在生成论文摘要