Representer Point Selection for Explaining Regularized High-dimensional Models
CoRR(2023)
Abstract
We introduce a novel class of sample-based explanations we term high-dimensional representers, that can be used to explain the predictions of a regularized high-dimensional model in terms of importance weights for each of the training samples. Our workhorse is a novel representer theorem for general regularized high-dimensional models, which decomposes the model prediction in terms of contributions from each of the training samples: with positive (negative) values corresponding to positive (negative) impact training samples to the model's prediction. We derive consequences for the canonical instances of $\ell_1$ regularized sparse models, and nuclear norm regularized low-rank models. As a case study, we further investigate the application of low-rank models in the context of collaborative filtering, where we instantiate high-dimensional representers for specific popular classes of models. Finally, we study the empirical performance of our proposed methods on three real-world binary classification datasets and two recommender system datasets. We also showcase the utility of high-dimensional representers in explaining model recommendations.
MoreTranslated text
Key words
models,selection,point,high-dimensional
AI Read Science
Must-Reading Tree
Example
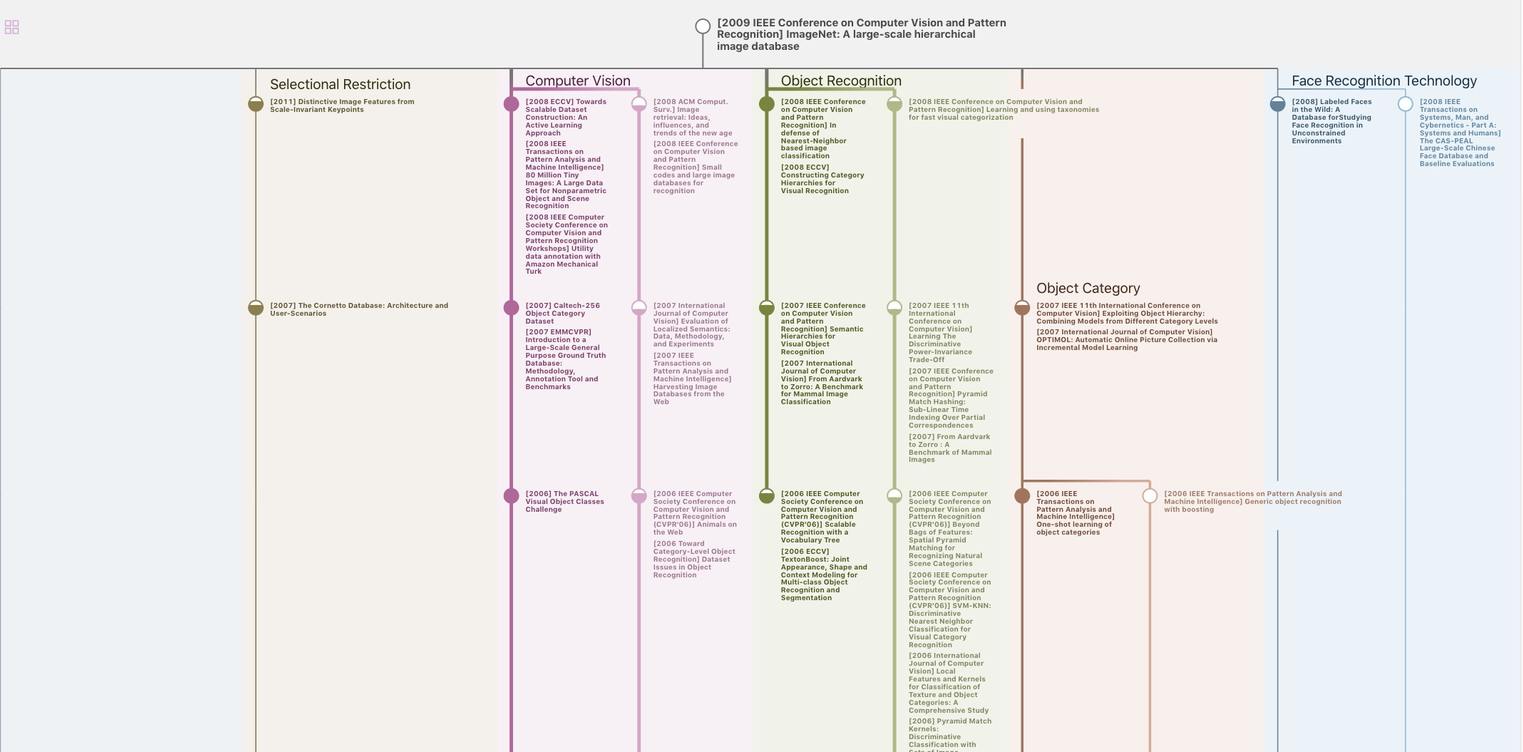
Generate MRT to find the research sequence of this paper
Chat Paper
Summary is being generated by the instructions you defined