Loss estimation and design of dc-dc converters using physics- and data-based component models
2023 IEEE Applied Power Electronics Conference and Exposition (APEC)(2023)
摘要
Design optimization of dc-dc converters at the component level can be assisted by machine learning (ML) techniques, where component models are trained using large amounts of component data. This approach enables systematic and effective optimization in terms of efficiency, cost, or size over a large design space. However, more accurate converter loss models require device parameters that are not readily available from component datasheets. This paper aims to combine physics-and data-based component models to address this challenge by generating additional design-oriented parameters that contribute to converter efficiency and can be used by the ML models in the optimization tool. Novel techniques are presented to include loss models due to transistor on-resistance and charge-equivalent capacitance, as well as inductor ac winding and core loss. Each approach is validated on out-of-sample components. The improved loss model is validated on a 48-to-12V, 5A synchronous buck converter prototype and is incorporated into the ML-based optimization tool.
更多查看译文
关键词
Data-driven component models,switched-mode power converters,dc-dc converter design,converter optimization,machine learning
AI 理解论文
溯源树
样例
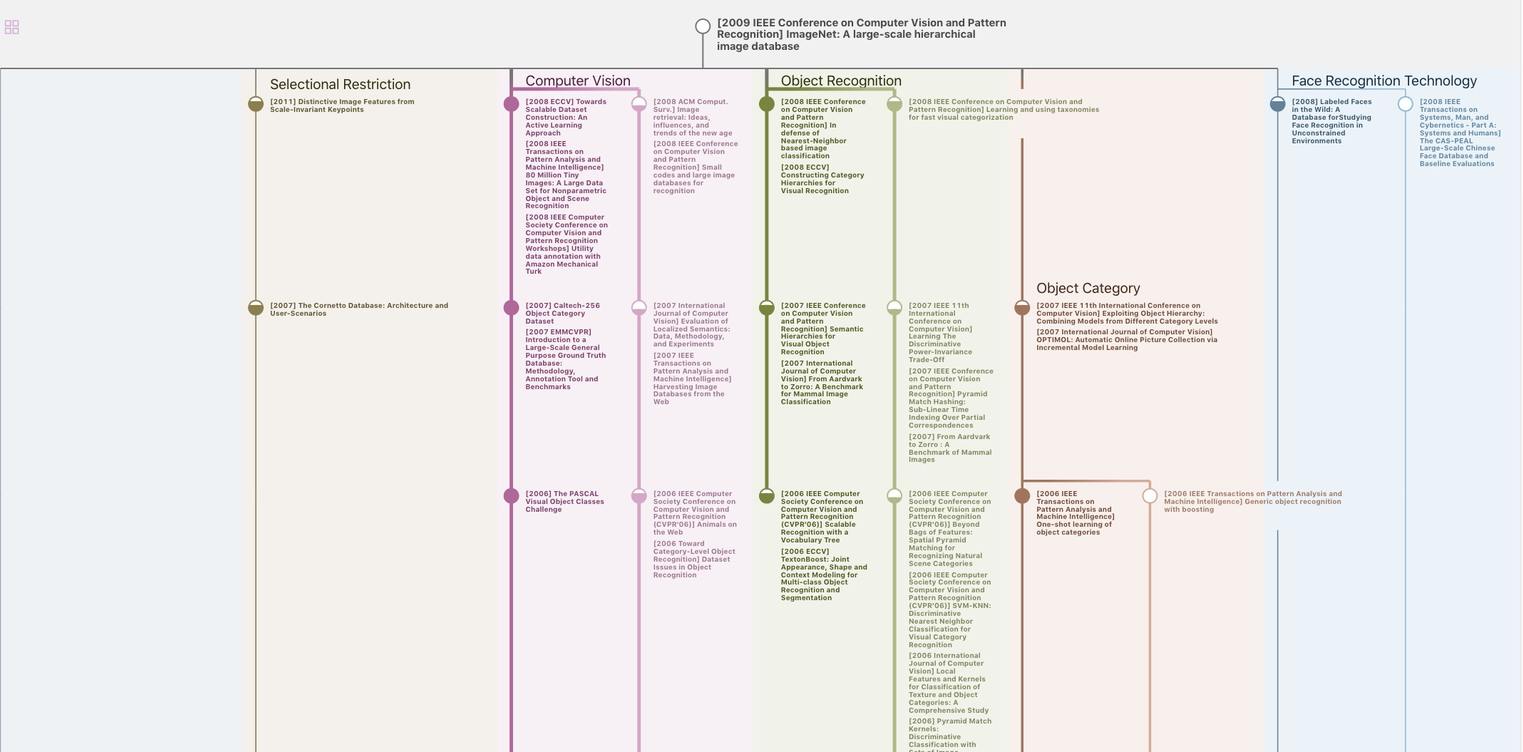
生成溯源树,研究论文发展脉络
Chat Paper
正在生成论文摘要