Representation Reliability and Its Impact on Downstream Tasks
CoRR(2023)
摘要
Self-supervised pre-trained models extract general-purpose representations from data, and quantifying how reliable they are is crucial because many downstream models use these representations as input for their own tasks. To this end, we first introduce a formal definition of representation reliability: the representation for a given test input is considered to be reliable if the downstream models built on top of that representation can consistently generate accurate predictions for that test point. It is desired to estimate the representation reliability without knowing the downstream tasks a priori. We provide a negative result showing that existing frameworks for uncertainty quantification in supervised learning are not suitable for this purpose. As an alternative, we propose an ensemble-based method for quantifying representation reliability, based on the concept of neighborhood consistency in the representation spaces across various pre-trained models. More specifically, the key insight is to use shared neighboring points as anchors to align different representation spaces. We demonstrate through comprehensive numerical experiments that our method is capable of predicting representation reliability with high accuracy.
更多查看译文
关键词
reliability
AI 理解论文
溯源树
样例
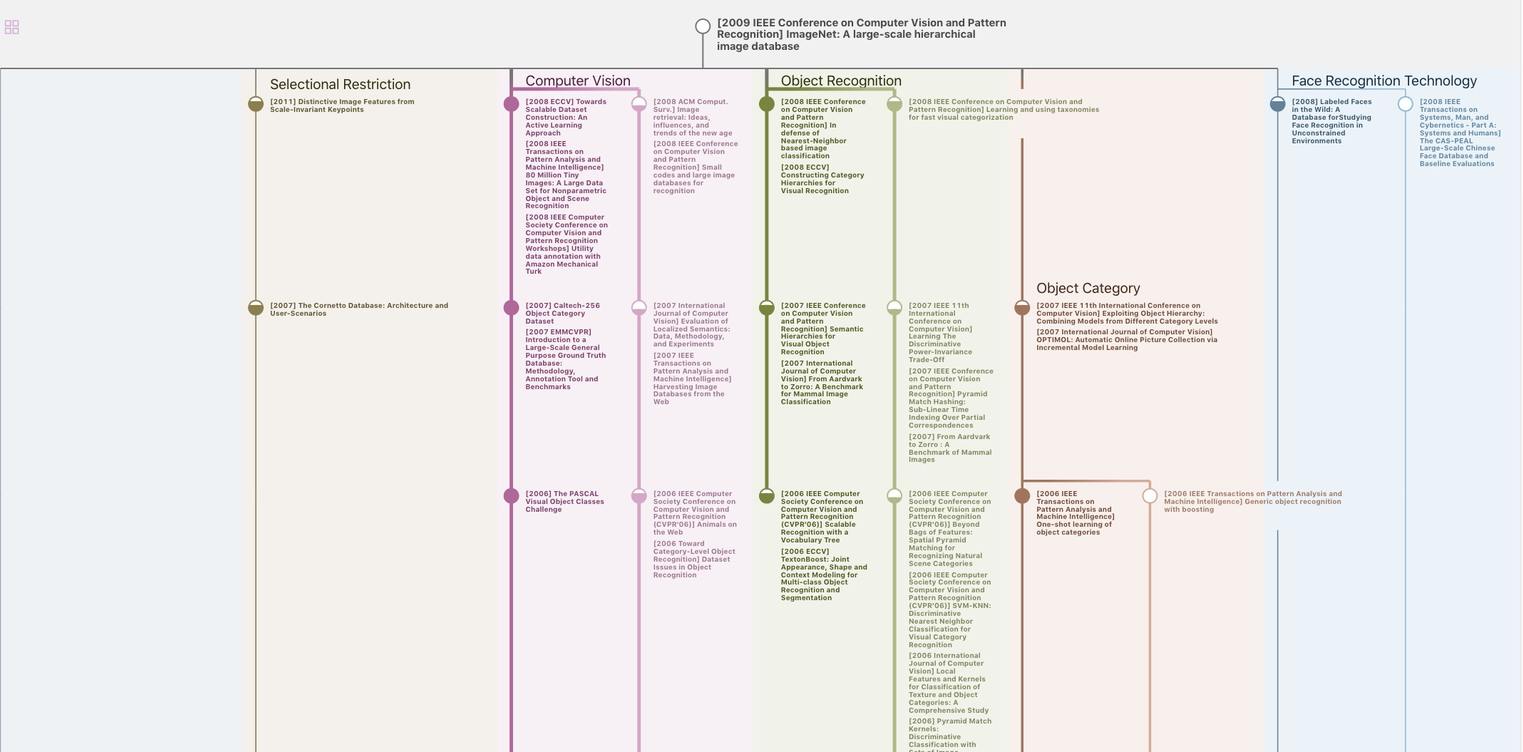
生成溯源树,研究论文发展脉络
Chat Paper
正在生成论文摘要