RHFedMTL: Resource-Aware Hierarchical Federated Multi-Task Learning
IEEE Internet of Things Journal(2023)
Abstract
The rapid development of artificial intelligence (AI) over massive applications including Internet-of-things on cellular network raises the concern of technical challenges such as privacy, heterogeneity and resource efficiency. Federated learning is an effective way to enable AI over massive distributed nodes with security. However, conventional works mostly focus on learning a single global model for a unique task across the network, and are generally less competent to handle multi-task learning (MTL) scenarios with stragglers at the expense of acceptable computation and communication cost. Meanwhile, it is challenging to ensure the privacy while maintain a coupled multi-task learning across multiple base stations (BSs) and terminals. In this paper, inspired by the natural cloud-BS-terminal hierarchy of cellular works, we provide a viable resource-aware hierarchical federated MTL (RHFedMTL) solution to meet the heterogeneity of tasks, by solving different tasks within the BSs and aggregating the multi-task result in the cloud without compromising the privacy. Specifically, a primal-dual method has been leveraged to effectively transform the coupled MTL into some local optimization sub-problems within BSs. Furthermore, compared with existing methods to reduce resource cost by simply changing the aggregation frequency, we dive into the intricate relationship between resource consumption and learning accuracy, and develop a resource-aware learning strategy for local terminals and BSs to meet the resource budget. Extensive simulation results demonstrate the effectiveness and superiority of RHFedMTL in terms of improving the learning accuracy and boosting the convergence rate.
MoreTranslated text
Key words
Federated Learning,Multi-Task Learning,Mobile Edge Computing,Artificial intelligence (AI)
AI Read Science
Must-Reading Tree
Example
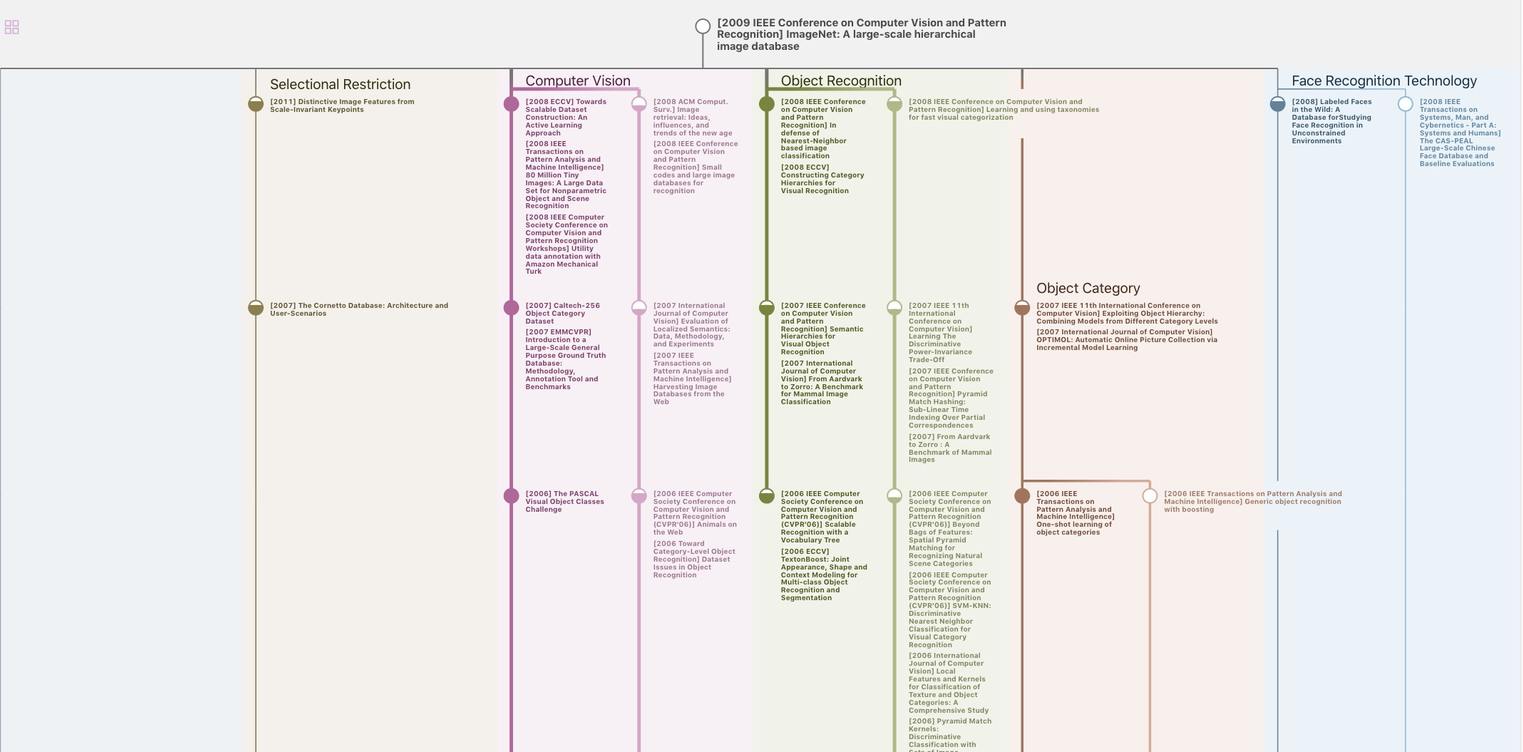
Generate MRT to find the research sequence of this paper
Chat Paper
Summary is being generated by the instructions you defined