Hyperspectral Target Detection Based on Low-Rank Background Subspace Learning and Graph Laplacian Regularization
CoRR(2023)
摘要
Hyperspectral target detection is good at finding dim and small objects based on spectral characteristics. However, existing representation-based methods are hindered by the problem of the unknown background dictionary and insufficient utilization of spatial information. To address these issues, this paper proposes an efficient optimizing approach based on low-rank representation (LRR) and graph Laplacian regularization (GLR). Firstly, to obtain a complete and pure background dictionary, we propose a LRR-based background subspace learning method by jointly mining the low-dimensional structure of all pixels. Secondly, to fully exploit local spatial relationships and capture the underlying geometric structure, a local region-based GLR is employed to estimate the coefficients. Finally, the desired detection map is generated by computing the ratio of representation errors from binary hypothesis testing. The experiments conducted on two benchmark datasets validate the effectiveness and superiority of the approach. For reproduction, the accompanying code is available at https://github.com/shendb2022/LRBSL-GLR.
更多查看译文
关键词
graph laplacian regularization,detection,low-rank
AI 理解论文
溯源树
样例
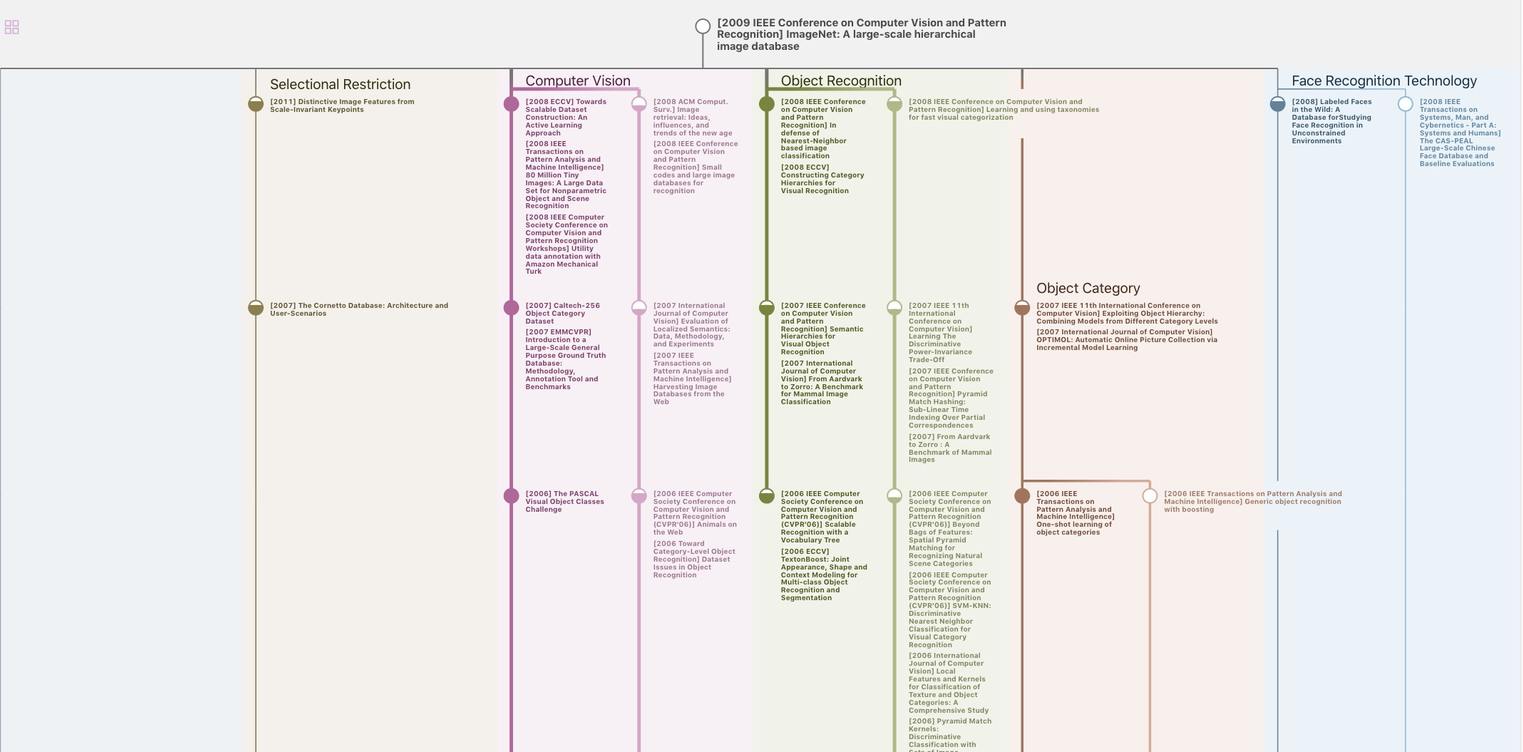
生成溯源树,研究论文发展脉络
Chat Paper
正在生成论文摘要