Data Interpolants -- That's What Discriminators in Higher-order Gradient-regularized GANs Are
CoRR(2023)
摘要
We consider the problem of optimizing the discriminator in generative adversarial networks (GANs) subject to higher-order gradient regularization. We show analytically, via the least-squares (LSGAN) and Wasserstein (WGAN) GAN variants, that the discriminator optimization problem is one of interpolation in $n$-dimensions. The optimal discriminator, derived using variational Calculus, turns out to be the solution to a partial differential equation involving the iterated Laplacian or the polyharmonic operator. The solution is implementable in closed-form via polyharmonic radial basis function (RBF) interpolation. In view of the polyharmonic connection, we refer to the corresponding GANs as Poly-LSGAN and Poly-WGAN. Through experimental validation on multivariate Gaussians, we show that implementing the optimal RBF discriminator in closed-form, with penalty orders $m \approx\lceil \frac{n}{2} \rceil $, results in superior performance, compared to training GAN with arbitrarily chosen discriminator architectures. We employ the Poly-WGAN discriminator to model the latent space distribution of the data with encoder-decoder-based GAN flavors such as Wasserstein autoencoders.
更多查看译文
关键词
gans,data interpolants,discriminators,higher-order,gradient-regularized
AI 理解论文
溯源树
样例
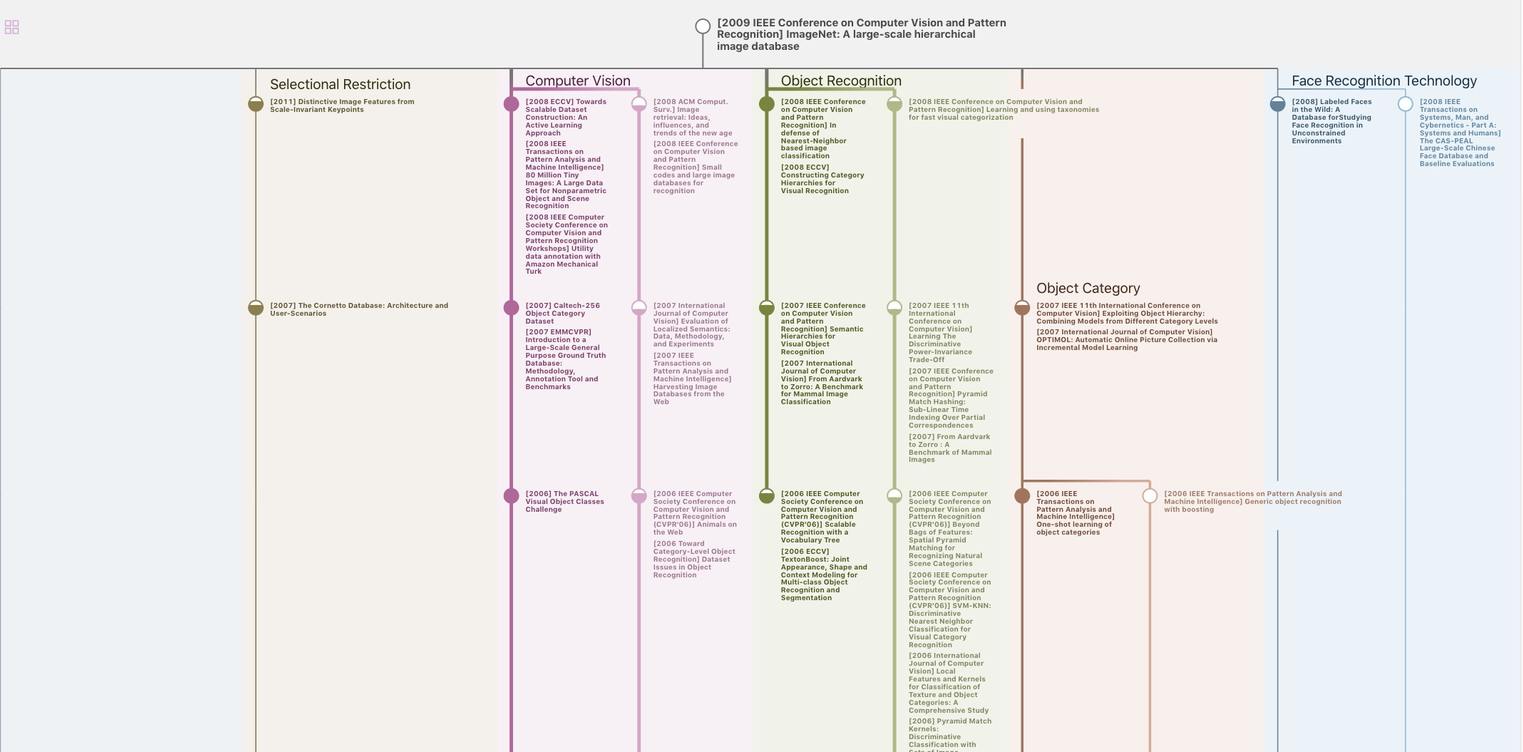
生成溯源树,研究论文发展脉络
Chat Paper
正在生成论文摘要