An attention-driven nonlinear optimization method for CS-based hyperspectral image reconstruction.
Theor. Comput. Sci.(2023)
摘要
Hyperspectral images contain both spatial and spectral information, which makes it has great advantages in the field of target identification, material determination, etc. However, hyperspectral image acquisition systems usually output massive amounts of data. Compressed sensing (CS) and single dispatchers coded aperture snapshot spectral imaging (SD-CASSI) were proposed to compress the amount of data. These systems usually use numerical optimization methods to do image reconstruction by finding the optimal reconstructed image. This method generates a large number of iterative calculations, which is time-consuming. In this paper, we modeled the image reconstruction for SC-CASSI as a non-linear optimization problem and proved that the number of iterations of the solution process is exponential. We proposed an attention-driven reconstruction algorithm for SD-CASSI systems. The algorithm mainly consists of an initial convolution block and residual attention modules. A channel attention module is also introduced to mine spectrally correlated features in raw signals. Experimental results show that the proposed algorithm is superior to three states-of-art reconstruction algorithms for CS-based systems. The reconstruction speed of the system is also increased by 94% compared with the numerical method. (c) 2023 Elsevier B.V. All rights reserved.
更多查看译文
关键词
Non-linear optimization, Hyperspectral imaging, Image reconstruction, Residual attention, Inverse matrix
AI 理解论文
溯源树
样例
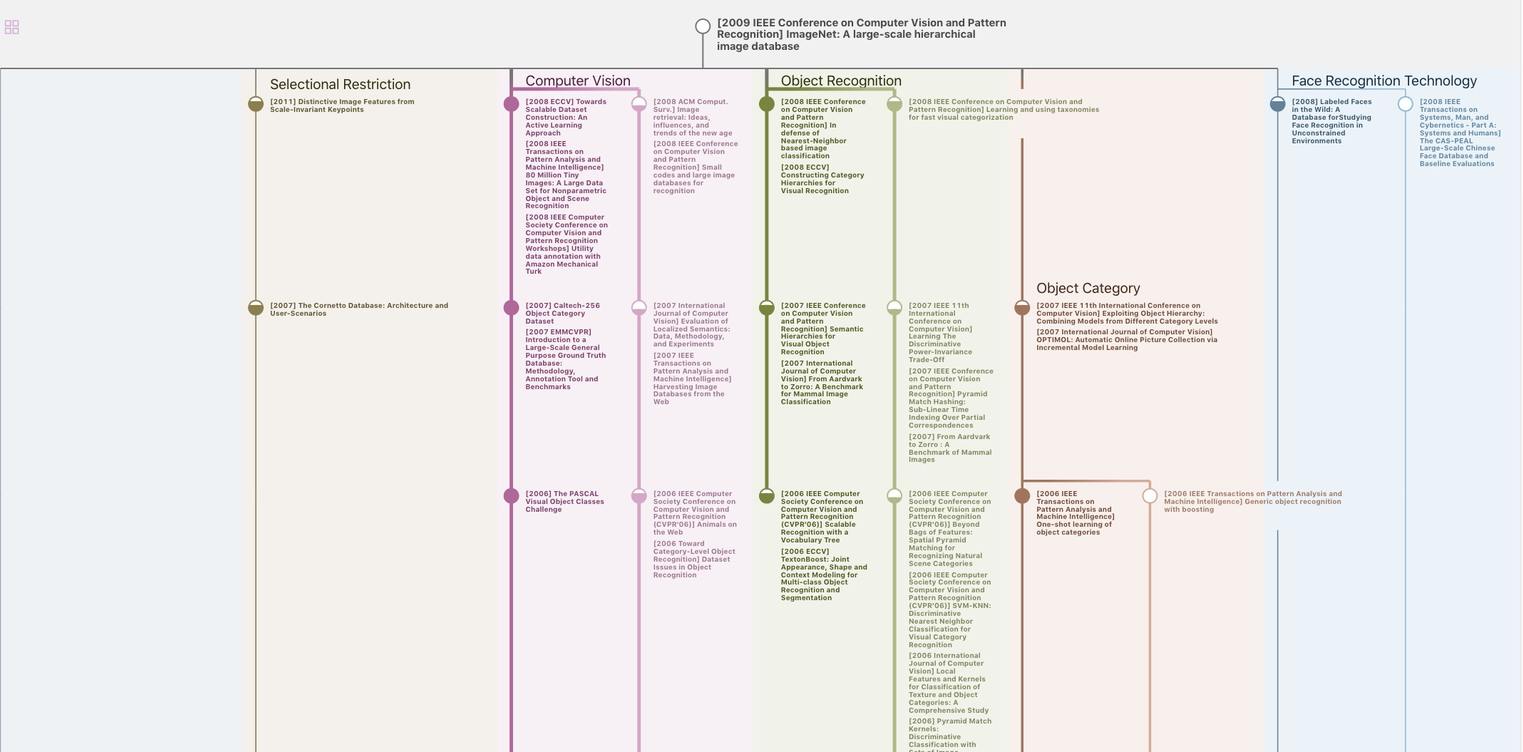
生成溯源树,研究论文发展脉络
Chat Paper
正在生成论文摘要