Single Image Dehazing via Fusion of Multilevel Attention Network for Vision-Based Measurement Applications.
IEEE Trans. Instrum. Meas.(2023)
摘要
Nowadays, researchers use vision-based measurement tools to record, detect, and monitor an atmospheric phenomenon called haze. It impedes the proper functioning of many outdoor industrial systems, such as autonomous driving, surveillance, satellite imagery, and so on. Conventional visibility restoration methods cannot accurately recover image quality due to inaccurate estimations of haze thickness and the presence of color-cast effects. Deep neural networks are evolving due to their ability to directly dehaze images from hazy scenes. Therefore, a unique attention-based end-to-end dehazing network named oval-net has been proposed in this study to restore clear images from its counterpart without employing the atmospheric scattering model. The oval-net is an encoder-decoder architecture that uses spatial and channel attention at each stage to focus on dominant and significant information while avoiding the transmission of irrelevant information from the encoder to the decoder, allowing quicker convergence. The proposed approach outperforms seven state-of-the-art algorithms in quantitative and qualitative assessments of a variety of synthetic and real-world hazy images, proving its effectiveness for vision-based industrial systems.
更多查看译文
关键词
Atmospheric modeling, Image restoration, Image color analysis, Atmospheric measurements, Feature extraction, Information filters, Discrete wavelet transforms, Channel attention (CA), encoder-decoder, image dehazing, spatial attention (SA), UNet
AI 理解论文
溯源树
样例
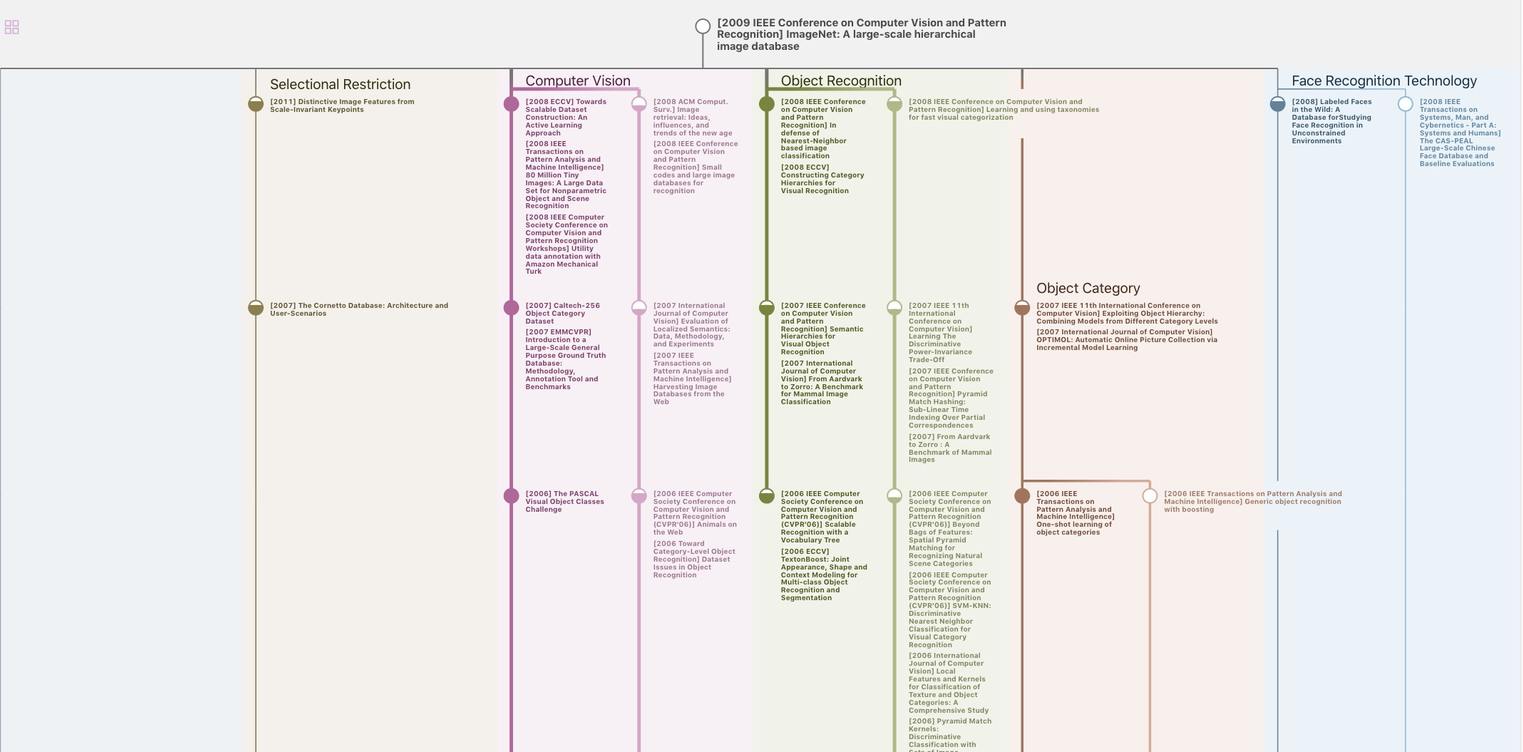
生成溯源树,研究论文发展脉络
Chat Paper
正在生成论文摘要