Classification of Gastric Lesions Using Gabor Block Local Binary Patterns.
Comput. Syst. Sci. Eng.(2023)
摘要
The identification of cancer tissues in Gastroenterology imaging poses novel challenges to the computer vision community in designing generic decision support systems. This generic nature demands the image descriptors to be invariant to illumination gradients, scaling, homogeneous illumination, and rotation. In this article, we devise a novel feature extraction methodology, which explores the effectiveness of Gabor filters coupled with Block Local Binary Patterns in designing such descriptors. We effectively exploit the illumination invariance properties of Block Local Binary Patterns and the inherent capability of convolutional neural networks to construct novel rotation, scale and illumination invariant features. The invariance characteristics of the proposed Gabor Block Local Binary Patterns (GBLBP) are demonstrated using a publicly available texture dataset. We use the proposed feature extraction methodology to extract texture features from Chromoendoscopy (CH) images for the classification of cancer lesions. The proposed feature set is later used in conjuncture with convolutional neural networks to classify the CH images. The proposed convolutional neural network is a shallow network comprising of fewer parameters in contrast to other state-of-the-art networks exhibiting millions of parameters required for effective training. The obtained results reveal that the proposed GBLBP performs favorably to several other state-of-the-art methods including both hand crafted and convolutional neural networks-based features.
更多查看译文
关键词
gastric lesions,classification
AI 理解论文
溯源树
样例
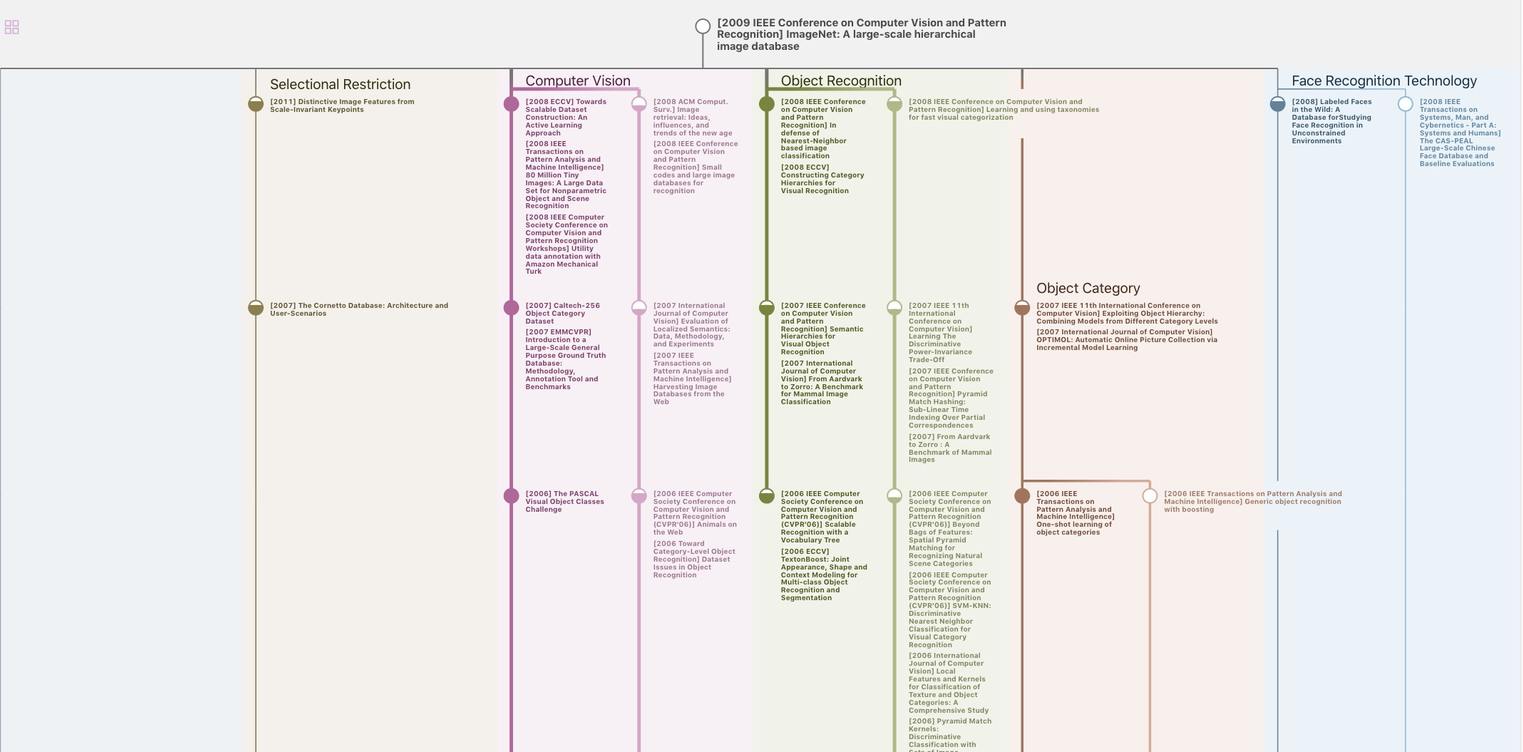
生成溯源树,研究论文发展脉络
Chat Paper
正在生成论文摘要